Estimation Method of Ship Propeller Parameters Based on Deep Convolutional Neural Network
2023 9th International Conference on Computer and Communications (ICCC)(2023)
摘要
In this paper, the deep convolution neural network is used to estimate the propeller parameters of ship. By using its ability to extract features form high-dimensional complex data, the accurate mapping from ship radiated noise to propeller parameters is realized. Firstly, the ship radiated noise is demodulated to generate DEMON spectrum. And through time accumulation and normalization processing, normalized DEMON spectrum samples conforming to deep learning are obtained. Then, a deep convolutional neural network model adapted to the usage scenario is constructed. In view of the large difference between the time and frequency dimension scales of the normalized DEMON spectrum graph, the different strides is adopted in some convolutional cores to reduce the influence of the asymmetry of the time and frequency dimension scales. In order to estimate the fundamental frequency and blade frequency of propeller simultaneously, two output layers are constructed on the top layer of the model to represent the estimation results of fundamental frequency and blade frequency respectively. On this basis, large-scale highly complex simulation DEMON spectrum samples are used to train and verify the performance of the deep convolutional neural network model. The results show that the model training process has good convergence. The accuracy of fundamental frequency estimation and blade number estimation is up to 92.9% and 95.6% under the condition of low signal-to-noise ratio, which verified the effectiveness of the deep convolutional neural network model to estimate propeller parameters.
更多查看译文
AI 理解论文
溯源树
样例
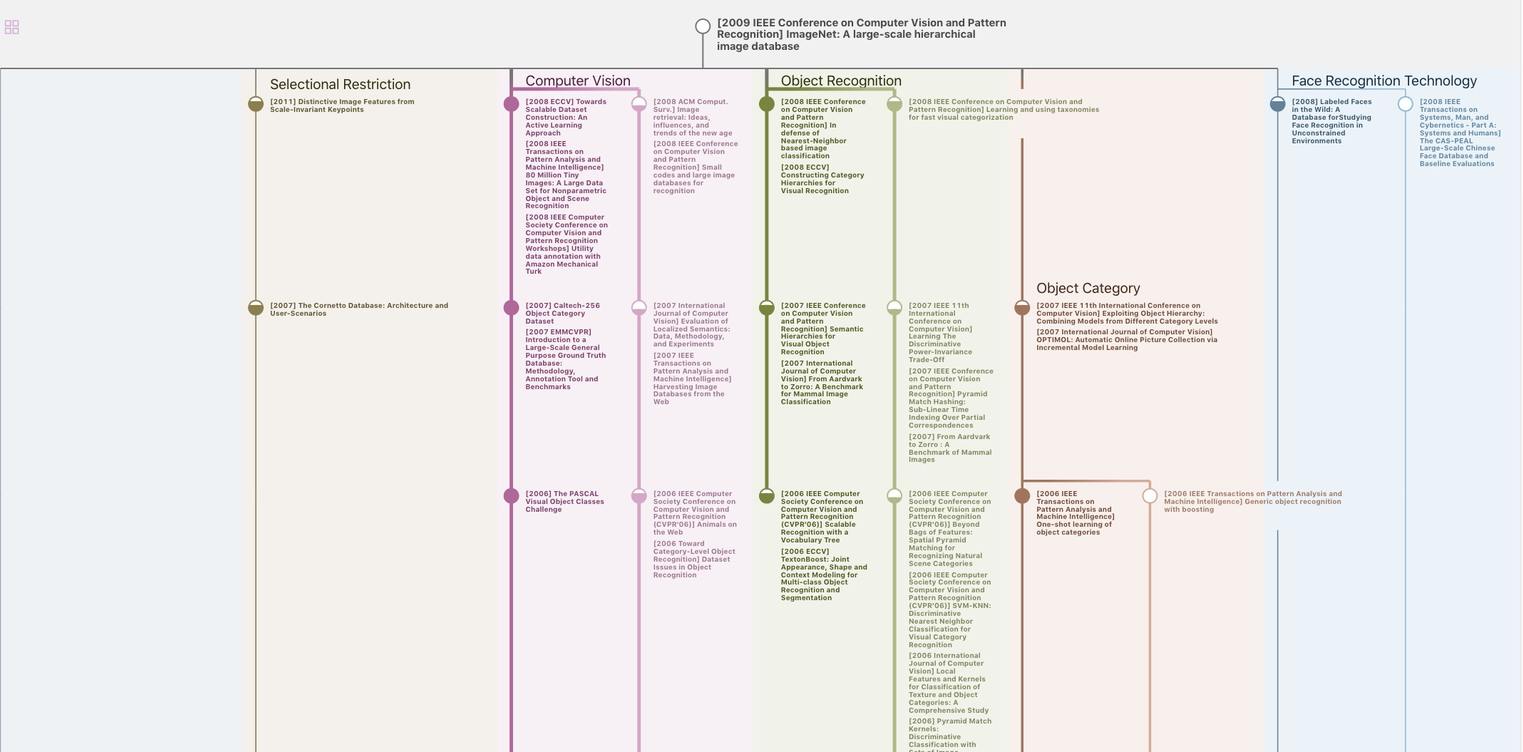
生成溯源树,研究论文发展脉络
Chat Paper
正在生成论文摘要