Real-Time Optimal Power Flow Method via Safe Deep Reinforcement Learning Based on Primal-Dual and Prior Knowledge Guidance
IEEE Transactions on Power Systems(2024)
摘要
High-level penetration of intermittent renewable energy sources (RESs) has introduced significant uncertainties into modern power systems. In order to rapidly and economically respond to the fluctuations of power system operating state, this paper proposes a safe deep reinforcement learning (SDRL) algorithm for the real-time optimal power flow problem. First, this problem is formulated as a Constrained Markov Decision Process model. Second, primal-dual proximal policy optimization (PD-PPO) is proposed to realize adaptively tuned binding effects on policy security constraints while achieving policy enhancement. Utilizing a cost critic network to evaluate policy security, actor gradients are estimated by a Lagrange advantage function derived from economic reward and violation cost critic networks with higher accuracy. Moreover, the performance of the PD-PPO method is further improved with an effective knowledge-driven action masking technique, which explicitly identifies critical action dimensions based on the physical model to encourage the policy in the safety direction with nonconservative exploration. Numerical tests are carried out on the IEEE 9-bus, 30-bus, 118-bus, and ACTIVSg2000 test systems. The results show that the well-trained SDRL agent can significantly improve the computation efficiency while satisfying security constraints and optimality requirements as much as possible.
更多查看译文
关键词
Knowledge-driven action masking,primal-dual proximal policy optimization,Real-time optimal power flow,safe deep reinforcement learning
AI 理解论文
溯源树
样例
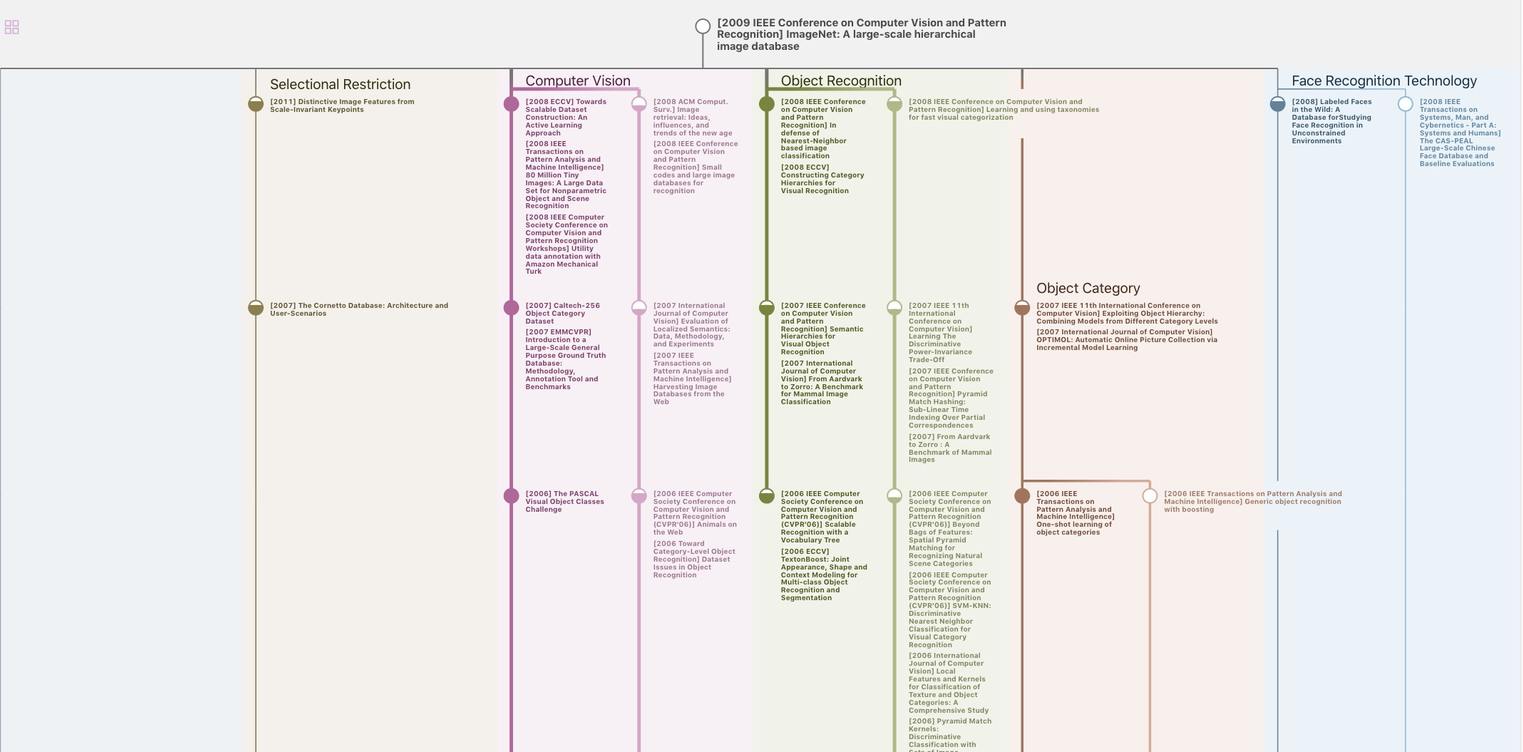
生成溯源树,研究论文发展脉络
Chat Paper
正在生成论文摘要