Parameter Selection by GCV and a χ^2 test within Iterative Methods for ℓ_1-regularized Inverse Problems
arxiv(2024)
摘要
ℓ_1 regularization is used to preserve edges or enforce sparsity in a
solution to an inverse problem. We investigate the Split Bregman and the
Majorization-Minimization iterative methods that turn this non-smooth
minimization problem into a sequence of steps that include solving an
ℓ_2-regularized minimization problem. We consider selecting the
regularization parameter in the inner generalized Tikhonov regularization
problems that occur at each iteration in these ℓ_1 iterative methods. The
generalized cross validation and χ^2 degrees of freedom methods are
extended to these inner problems. In particular, for the χ^2 method this
includes extending the χ^2 result for problems in which the regularization
operator has more rows than columns, and showing how to use the A-weighted
generalized inverse to estimate prior information at each inner iteration.
Numerical experiments for image deblurring problems demonstrate that it is more
effective to select the regularization parameter automatically within the
iterative schemes than to keep it fixed for all iterations. Moreover, an
appropriate regularization parameter can be estimated in the early iterations
and used fixed to convergence.
更多查看译文
AI 理解论文
溯源树
样例
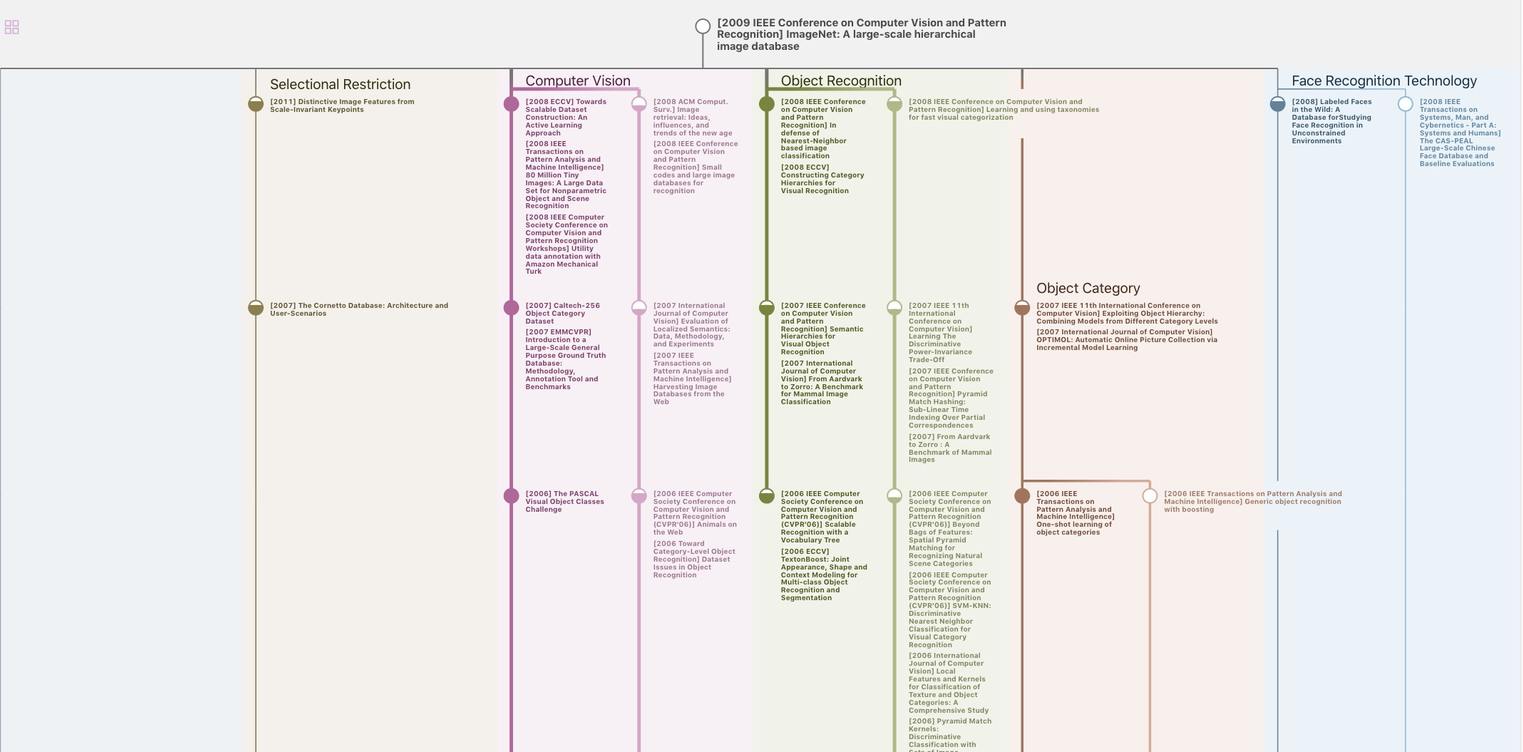
生成溯源树,研究论文发展脉络
Chat Paper
正在生成论文摘要