Exploring the potential of deep learning for streamflow forecasting: A comparative study with hydrological models for seasonal and perennial rivers
Expert Systems with Applications(2024)
摘要
Improving streamflow prediction plays a significant role in flood warning, mitigation and development purposes. Therefore, this paper aims to compare the prediction capability of a useful conceptual hydrologic model hydrologiska byråns vattenbalansavdelning (HBV) enhanced and equipped with an automatic calibration procedure (Genetic Algorithm) along with two well-known deep learning (DL) algorithms, including Long-Short-Term Memory (LSTM) and Gated Recurrent Unit (GRU). This study was concentrated on the daily streamflow of two river basins with diverse climatic and hydrological conditions, namely the Halilrood River with fragmented and intermittent flows (located in Iran) and the Tweed River with a permanent stream flow (Located across the border of Scotland and northern England). These were particularly selected to address both seasonal and perennial riverine cases. For reproducing the streamflow, 10-year records of the daily discharge, precipitation, and temperature (from satellite and ground resources), and evapotranspiration were used as the main input data. The accuracy of the implemented models was assessed using several indices. The findings show that 1) the LSTM and GRU show better accuracy than the employed hydrologic model for estimating daily discharges (especially in the seasonal river basin); 2) the DL approaches need only meteorological data inputs (precipitation and temperature) and are independent of involved uncertainties in hydrological modeling such as physical characteristics of basins; and 3) best streamflow simulations were achieved by the LSTM (best agreement with the observations). In general, LSTM has the highest priority to be connected with online hydrological rainfall-runoff simulations and dynamical forecast models.
更多查看译文
关键词
LSTM,GRU,HBV,Halilrood,Tweed,Streamflow Forecasting
AI 理解论文
溯源树
样例
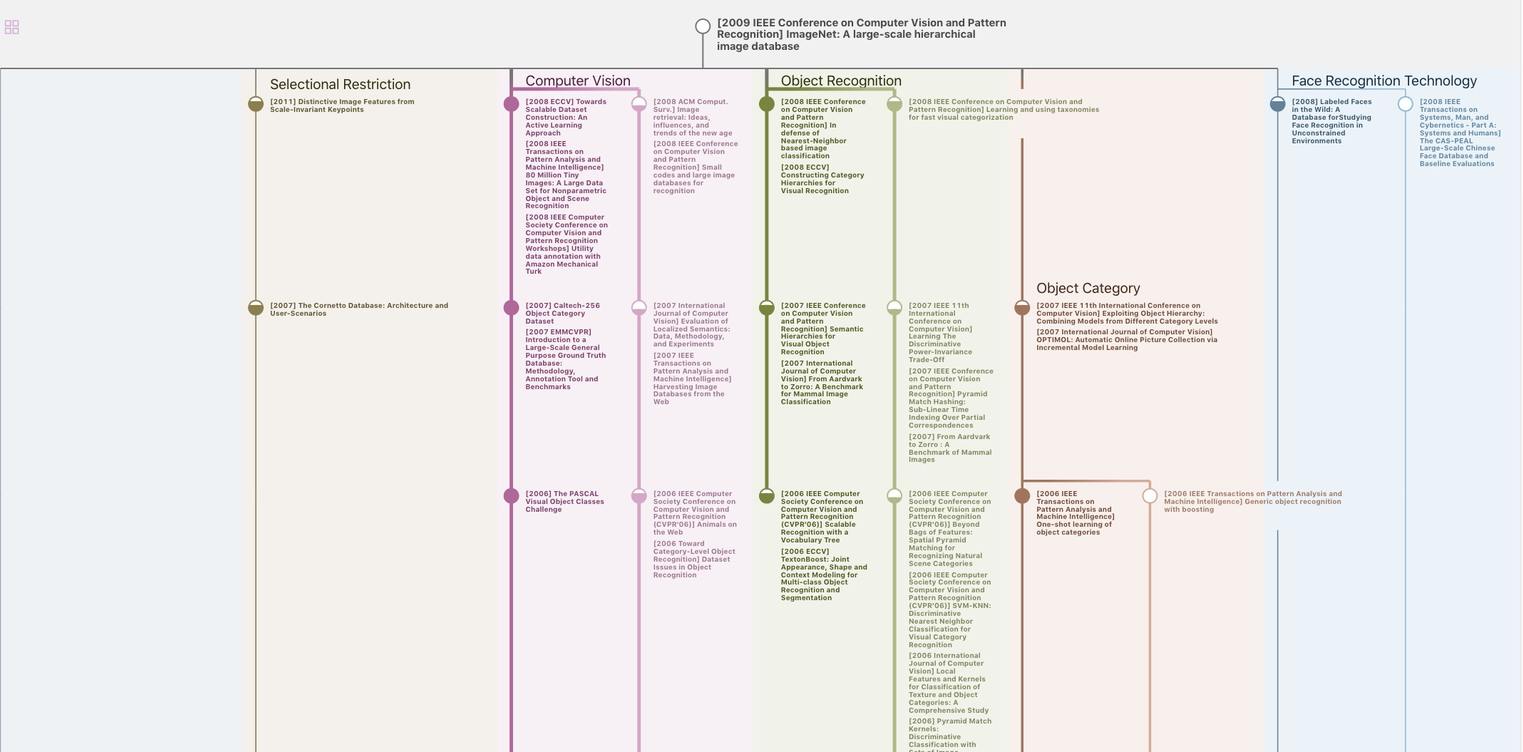
生成溯源树,研究论文发展脉络
Chat Paper
正在生成论文摘要