Deep Boosting Learning: A Brand-New Cooperative Approach for Image-Text Matching.
IEEE transactions on image processing : a publication of the IEEE Signal Processing Society(2024)
摘要
Image-text matching remains a challenging task due to heterogeneous semantic diversity across modalities and insufficient distance separability within triplets. Different from previous approaches focusing on enhancing multi-modal representations or exploiting cross-modal correspondence for more accurate retrieval, in this paper we aim to leverage the knowledge transfer between peer branches in a boosting manner to seek a more powerful matching model. Specifically, we propose a brand-new Deep Boosting Learning (DBL) algorithm, where an anchor branch is first trained to provide insights into the data properties, with a target branch gaining more advanced knowledge to develop optimal features and distance metrics. Concretely, an anchor branch initially learns the absolute or relative distance between positive and negative pairs, providing a foundational understanding of the particular network and data distribution. Building upon this knowledge, a target branch is concurrently tasked with more adaptive margin constraints to further enlarge the relative distance between matched and unmatched samples. Extensive experiments validate that our DBL can achieve impressive and consistent improvements based on various recent state-of-the-art models in the image-text matching field, and outperform related popular cooperative strategies, e.g., Conventional Distillation, Mutual Learning, and Contrastive Learning. Beyond the above, we confirm that DBL can be seamlessly integrated into their training scenarios and achieve superior performance under the same computational costs, demonstrating the flexibility and broad applicability of our proposed method.
更多查看译文
关键词
Image-text matching,Deep boosting learning,Deep cooperative learning,Deep metric learning
AI 理解论文
溯源树
样例
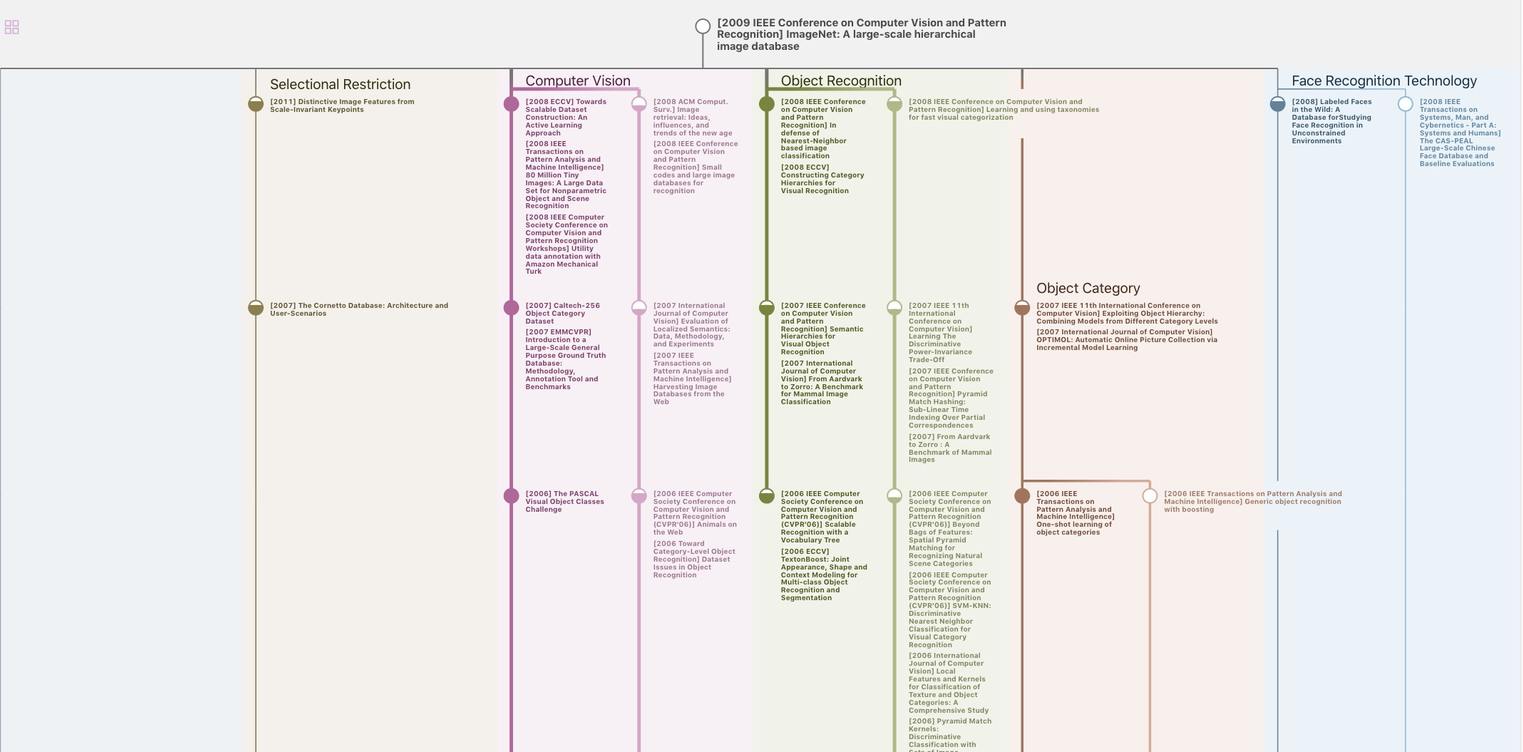
生成溯源树,研究论文发展脉络
Chat Paper
正在生成论文摘要