Target Detection with Spectral Graph Contrast Clustering Assignment and Spectral Graph Transformer in Hyperspectral Imagery
IEEE Transactions on Geoscience and Remote Sensing(2024)
摘要
Hyperspectral target detection is a method that recognizes objects of interest in a scene by a priori target spectrum. Local details and global information on the spectra are critical for accurate target identification. Detectors with excellent discrimination of spectral differences can better highlight targets while suppressing background. To this end, this paper proposes a hyperspectral target detection method based on spectral graph contrast clustering assignment and Spectral Graph Transformer to solve these problems. Specifically, for local-global feature extraction of spectra, the pixel spectra are first constructed as the spectral graph. Then, the representations of the first-order or higher-order neighbors of the nodes in the spectral graph are aggregated using graph convolutional networks to extract the local detail information of the spectra. The self-attention in Transformer is utilized to learn the global information of the spectra. Secondly, a novel spectral graph contrast clustering assignment method is proposed to equip the model with excellent spectral discrimination ability. It maintains clustering consistency by swapping predictive clustering assignments while maximizing the similarity of semantically similar graph clusters and keeping other semantically different graph clusters away from them to better discriminate differences between spectra. Finally, comparisons with seven state-of-the-art hyperspectral target detection methods on four real hyperspectral data sets and ablation studies verify the effectiveness of the proposed method in hyperspectral target detection.
更多查看译文
关键词
Contrast clustering,hyperspectral imagery,Spectral Graph Transformer,target detection
AI 理解论文
溯源树
样例
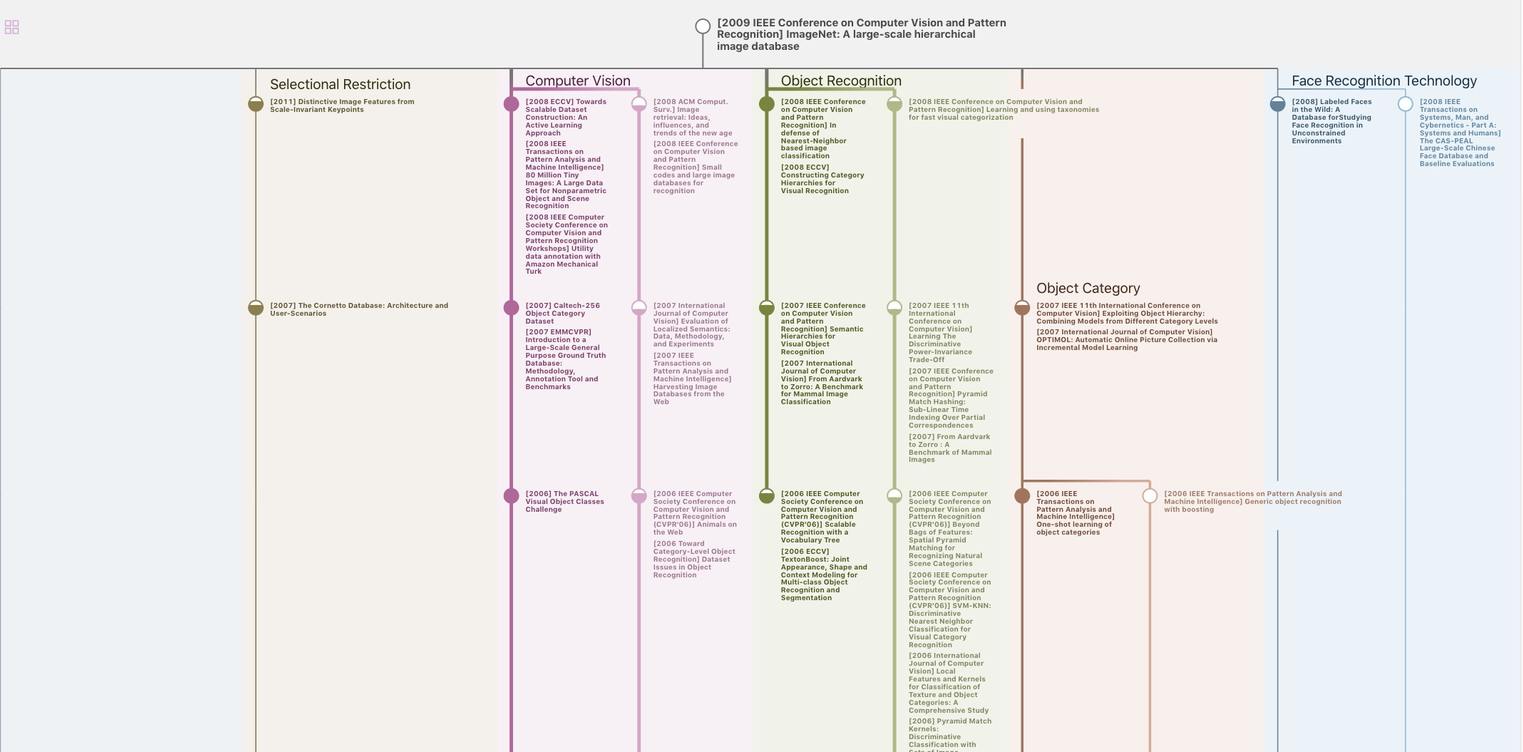
生成溯源树,研究论文发展脉络
Chat Paper
正在生成论文摘要