Evaluation of Transformer Architectures for Electrical Load Time-Series Forecasting
Proceedings - 32. Workshop Computational Intelligence: Berlin, 1. - 2. Dezember 2022(2022)
摘要
Accurate forecasts of the electrical load are needed to stabilize the electrical grid and maximize the use of renewable energies. Many good forecasting methods exist, including neural networks, and we compare them to the recently developed Transformers, which are the state-of-the-art machine learning technique for many sequence-related tasks. We apply different types of Transformers, namely the Time-Series Transformer, the Convolutional Self- Attention Transformer and the Informer, to electrical load data from Baden- Württemberg. Our results show that the Transformes give up to 11% better forecasts than multi-layer perceptrons for long prediction horizons. Furthermore, we analyze the Transformers’ attention scores to get insights into the model.
更多查看译文
AI 理解论文
溯源树
样例
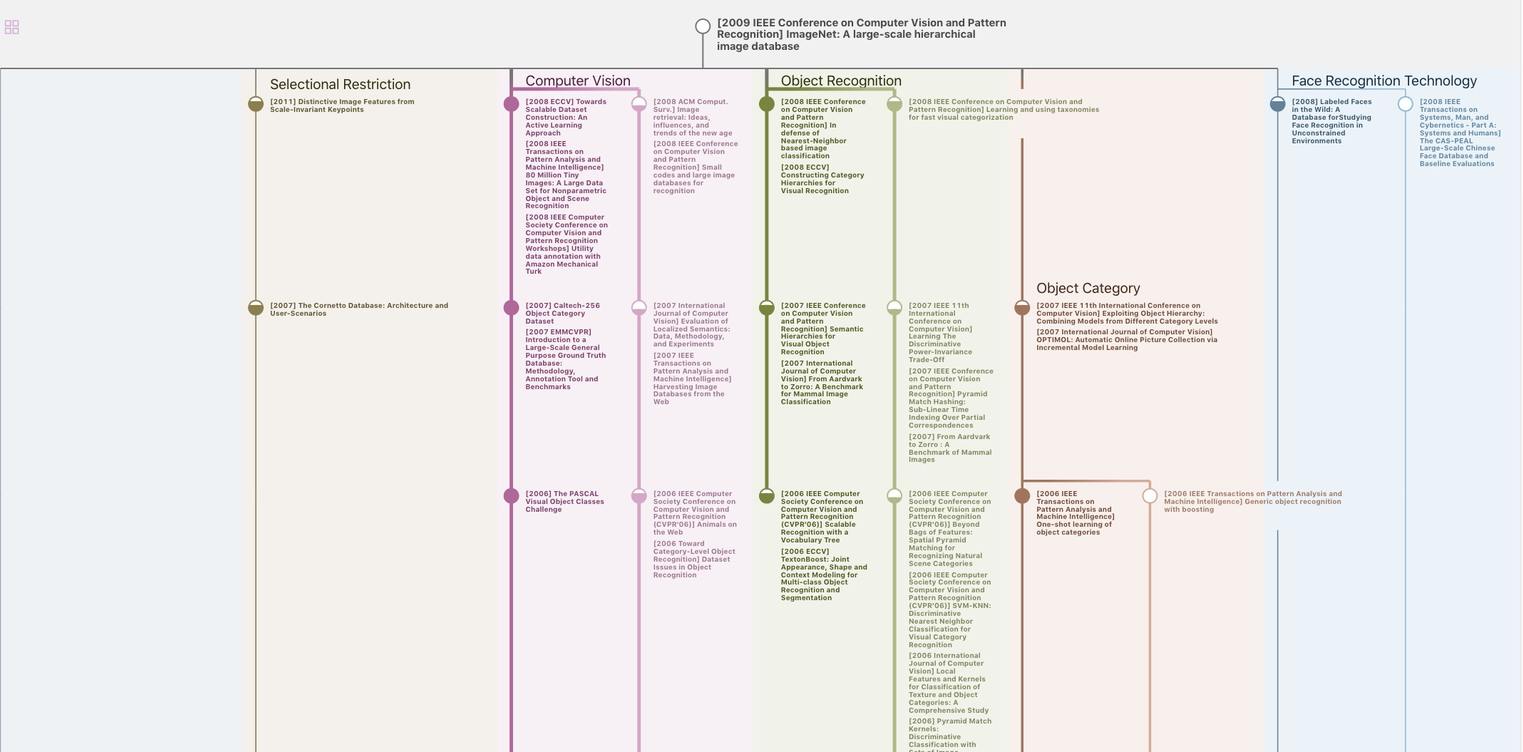
生成溯源树,研究论文发展脉络
Chat Paper
正在生成论文摘要