Framework Based on Machine Learning Approach for Prediction of the Remaining Useful Life: A Case Study of an Aviation Engine
Journal of Failure Analysis and Prevention(2024)
摘要
This paper provides a framework based on machine learning approach in I4.0 environment to predict the remaining useful life of an aviation engine. For illustration purpose, an industrial case study is presented which applies machine learning algorithms to analyze the data collected using the Commercial Modular Aero-Propulsion System Simulation (C-MAPSS) simulation which includes run-to-degradation data for a turbofan engine. The results obtained from the study validate the proposed framework to identify prominent features and perform sequential analysis on unstructured data for predicting the remaining useful life of an aviation engine. Six machine learning models are applied to the dataset containing four subsets: FD001, FD002, FD003 and FD004 in C-MAPSS dataset each working on different degradation conditions for turbofan engine. For FD001, random forest had the lowest RMSE (11.59), and for FD002, FD003 and FD004, the lowest RMSE was given by LGBM classifier (12.78, 7.95 and 11.04), respectively. From the findings, it is observed that LGBM performs better with higher AUC 89
更多查看译文
关键词
Industry 4.0,Predictive maintenance,Remaining useful life,Prognostics,Feature extraction
AI 理解论文
溯源树
样例
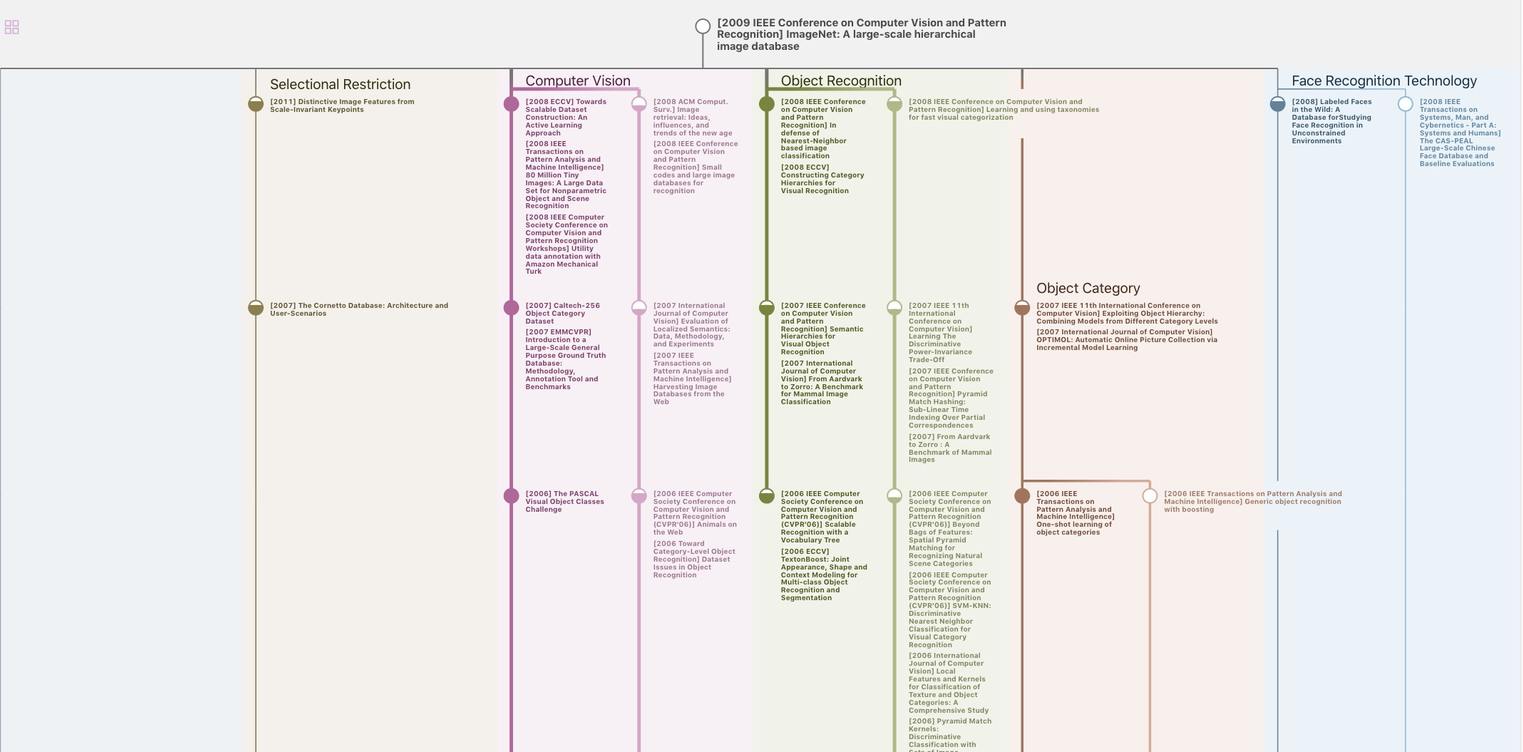
生成溯源树,研究论文发展脉络
Chat Paper
正在生成论文摘要