When to Trust LLMs: Aligning Confidence with Response Quality
arxiv(2024)
摘要
Despite the success of large language models (LLMs) in natural language
generation, much evidence shows that LLMs may produce incorrect or nonsensical
text. This limitation highlights the importance of discerning when to trust
LLMs, especially in safety-critical domains. Existing methods, which rely on
verbalizing confidence to tell the reliability by inducing top-k responses and
sampling-aggregating multiple responses, often fail, due to the lack of
objective guidance of confidence. To address this, we propose
CONfidence-Quality-ORDerpreserving alignment approach (CONQORD), leveraging
reinforcement learning with a tailored dual-component reward function. This
function encompasses quality reward and orderpreserving alignment reward
functions. Specifically, the order-preserving reward incentivizes the model to
verbalize greater confidence for responses of higher quality to align the order
of confidence and quality. Experiments demonstrate that our CONQORD
significantly improves the alignment performance between confidence levels and
response accuracy, without causing the model to become over-cautious.
Furthermore, the aligned confidence provided by CONQORD informs when to trust
LLMs, and acts as a determinant for initiating the retrieval process of
external knowledge. Aligning confidence with response quality ensures more
transparent and reliable responses, providing better trustworthiness.
更多查看译文
AI 理解论文
溯源树
样例
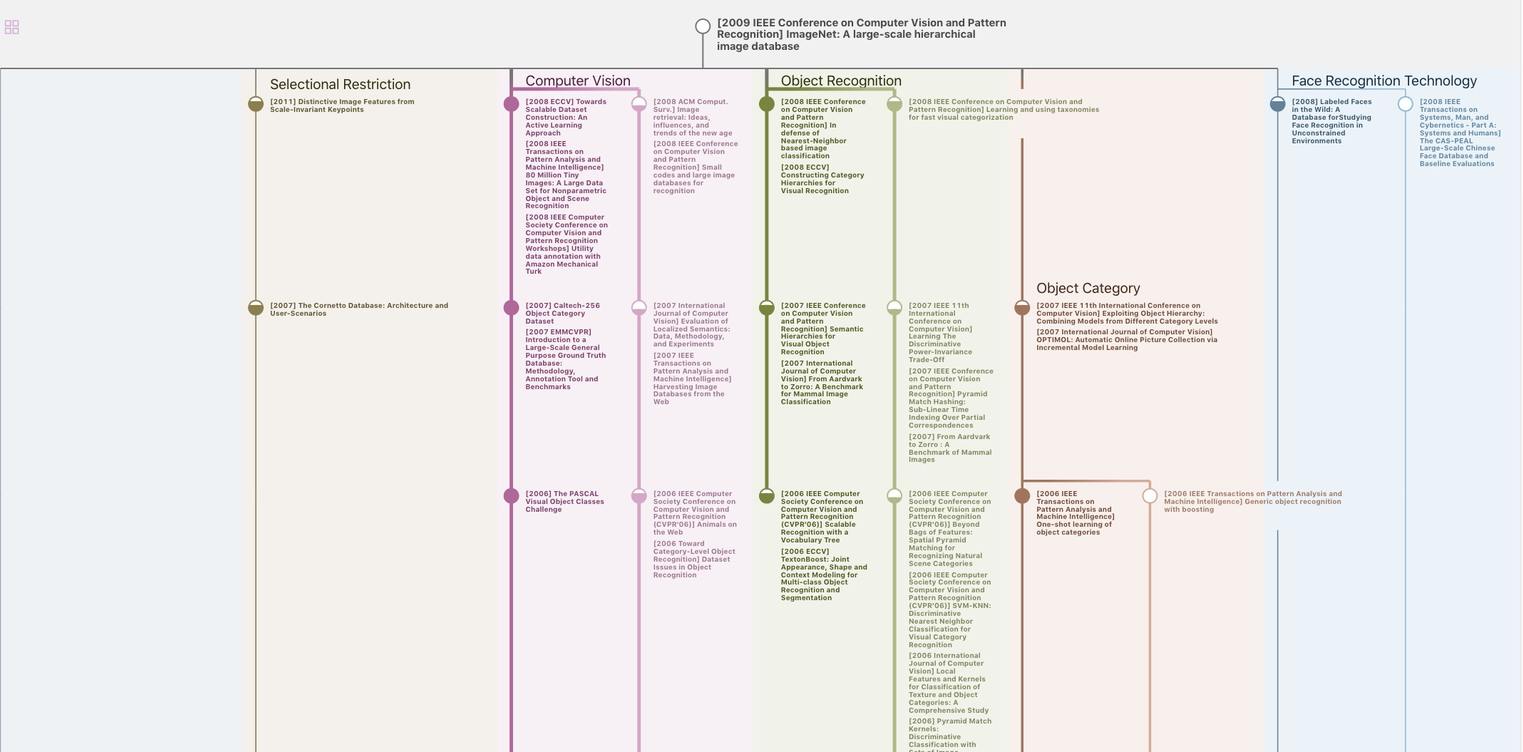
生成溯源树,研究论文发展脉络
Chat Paper
正在生成论文摘要