Device Feature based on Graph Fourier Transformation with Logarithmic Processing For Detection of Replay Speech Attacks
arxiv(2024)
摘要
The most common spoofing attacks on automatic speaker verification systems
are replay speech attacks. Detection of replay speech heavily relies on replay
configuration information. Previous studies have shown that graph Fourier
transform-derived features can effectively detect replay speech but ignore
device and environmental noise effects. In this work, we propose a new feature,
the graph frequency device cepstral coefficient, derived from the graph
frequency domain using a device-related linear transformation. We also
introduce two novel representations: graph frequency logarithmic coefficient
and graph frequency logarithmic device coefficient. We evaluate our methods
using traditional Gaussian mixture model and light convolutional neural network
systems as classifiers. On the ASVspoof 2017 V2, ASVspoof 2019 physical access,
and ASVspoof 2021 physical access datasets, our proposed features outperform
known front-ends, demonstrating their effectiveness for replay speech
detection.
更多查看译文
AI 理解论文
溯源树
样例
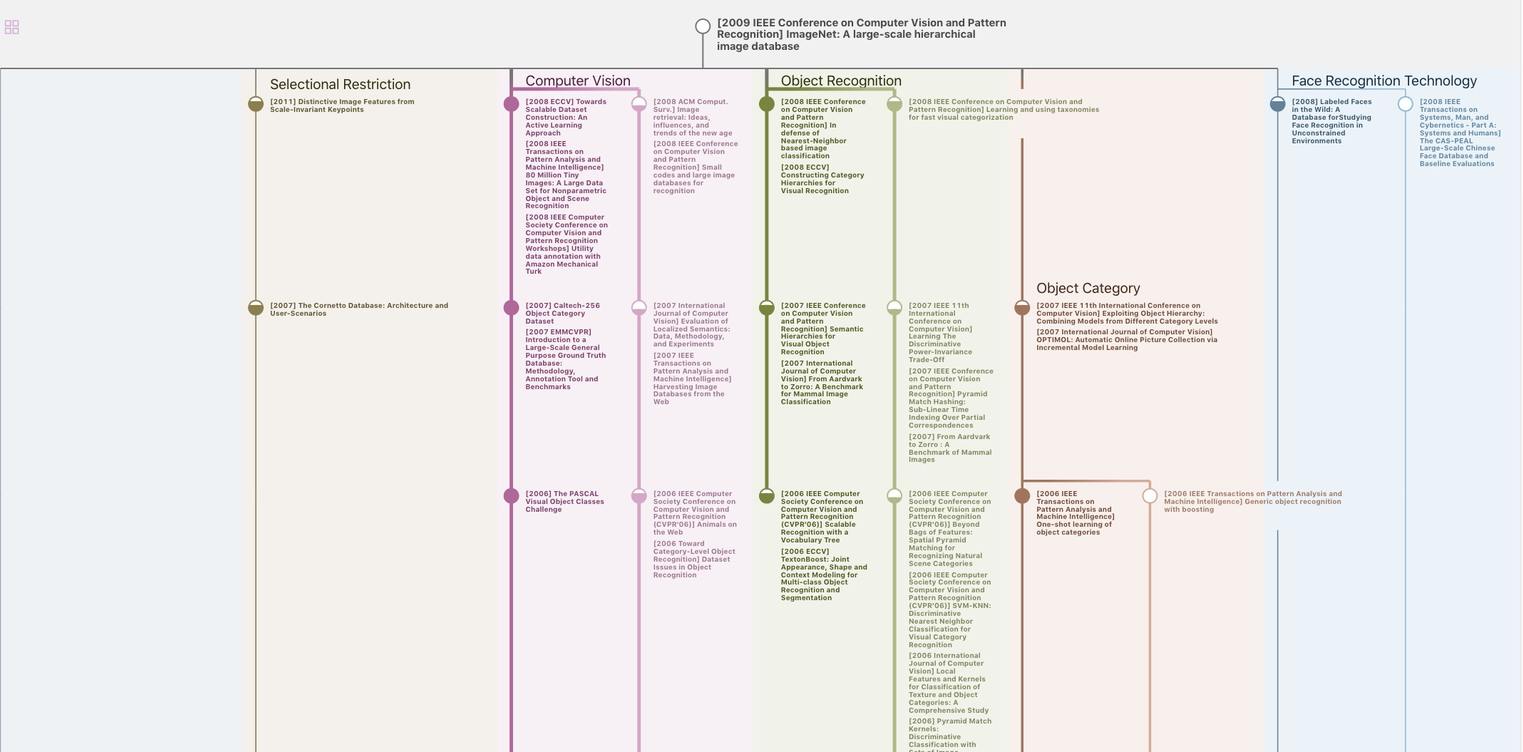
生成溯源树,研究论文发展脉络
Chat Paper
正在生成论文摘要