Prediction analysis of TBI 24-h survival outcome based on machine learning.
Heliyon(2024)
摘要
Background:Traumatic brain injury (TBI) is the major reason for the death of young people and is well known for its high mortality and morbidity. This paper aim to predict the 24h survival of patients with TBI.
Methods:A total of 1224 samples were involved in this analysis, and the clinical indicators involved included age, gender, blood pressure, MGAP and other fields, among which the target variable was "outcome", which was a binary variable. The methods mainly involved in this paper include data visualization analysis, single factor analysis, feature engineering analysis, random forest model (RF), K-Nearst Neighbors (KNN) model, and so on. Logistic regression model (LR) and deep neural network model (DNN). We will oversample the training set using the SMOTE method because of the very unbalanced labeling of the sample itself.
Results:Although the accuracy of all models is very high, the recall rate is relatively low. The DNN model with the best performance only reaches 0.17, and the corresponding AUC is 0.80. After resampling, we find that the recall rate of positive samples of all models has increased a lot, but the AUC of some models has decreased. Finally, the optimal model is LR, whose positive sample recall rate is 0.67 and AUC is 0.82.
Conclusion:Through resampling, we obtained that the best model is the RF model, whose recall rate and AUC are the best, and the AUC level is about 0.87, indicating that the accuracy performance of the model is still good.
更多查看译文
关键词
survival,Trauma,Machine learning,RF,KNN,LR
AI 理解论文
溯源树
样例
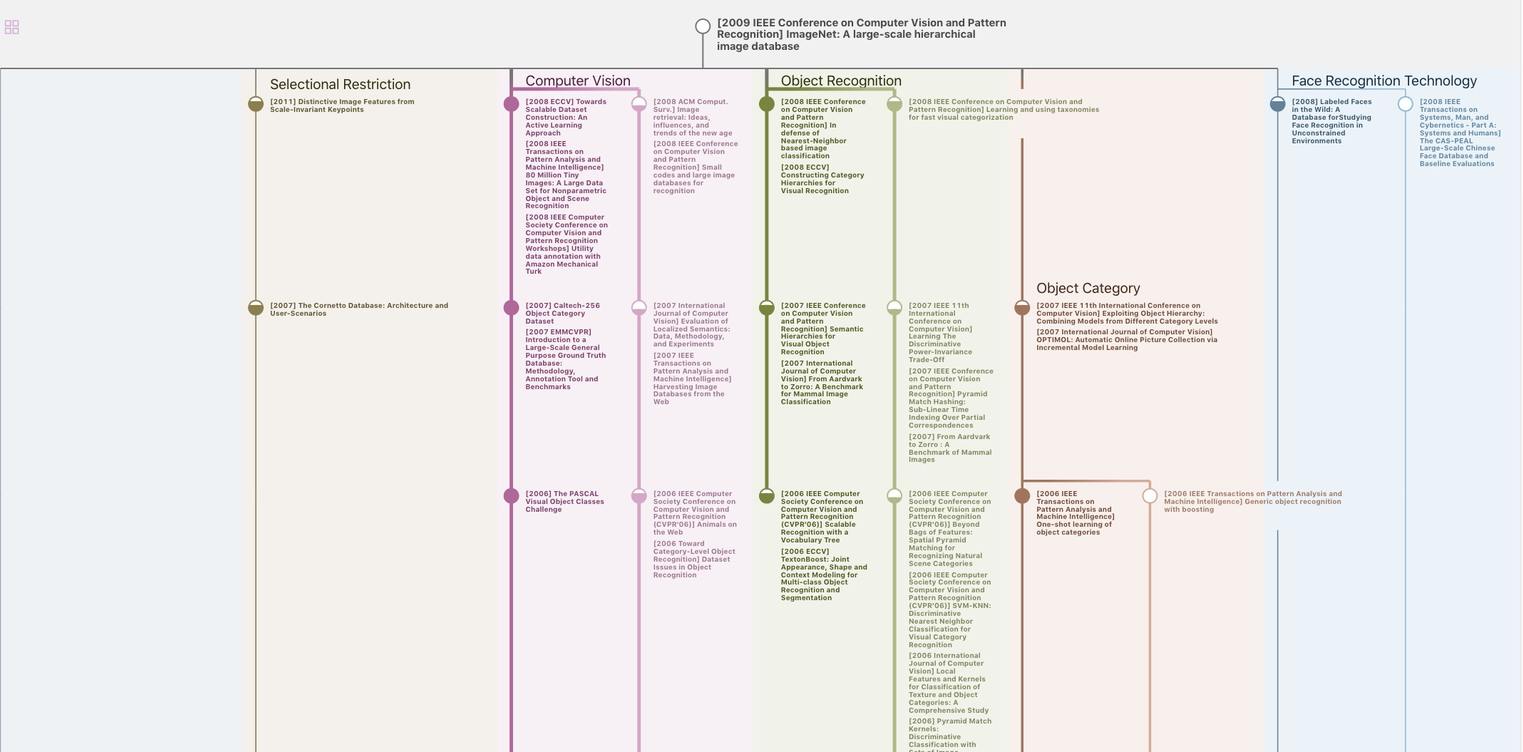
生成溯源树,研究论文发展脉络
Chat Paper
正在生成论文摘要