Transferable Adversarial Slow Feature Extraction Network for Few-Shot Quality Prediction in Coal-to-Ethylene Glycol Process
Chinese Journal of Chemical Engineering(2024)
摘要
In coal-to-ethylene glycol (CTEG) process, precisely estimating quality variables is crucial for process monitoring, optimization, and control. A significant challenge in this regard is relying on offline laboratory analysis to obtain these variables, which often incurs substantial monetary costs and significant time delays. The resulting few-shot learning scenarios present a hurdle to the efficient development of predictive models. To address this issue, our study introduces the Transferable Adversarial Slow Feature Extraction Network (TASF-Net), an innovative approach designed specifically for few-shot quality prediction in the CTEG process. TASF-Net uniquely integrates the slowness principle with a deep Bayesian framework, effectively capturing the nonlinear and inertial characteristics of the CTEG process. Additionally, the model employs a variable attention mechanism to identify quality-related input variables adaptively at each time step. A key strength of TASF-Net lies in its ability to navigate the complex measurement noise, outliers, and system interference typical in CTEG data. adversarial learning strategy using a min-max game is adopted to improve its robustness and ability to model irregular industrial data accurately and significantly. Furthermore, an incremental refining transfer learning framework is designed to further improve few-shot prediction performance achieved by transferring knowledge from the pre-trained model on the source domain to target domain. The effectiveness and superiority of TASF-Net have been empirically validated using a real-world CTEG dataset. Compared with some state-of-the-art methods, TASF-Net demonstrates exceptional capability in addressing the intricate challenges for few-shot quality prediction in the CTEG process.
更多查看译文
关键词
Chemical process,Neural networks,Slowness principle,Transfer learning,Prediction
AI 理解论文
溯源树
样例
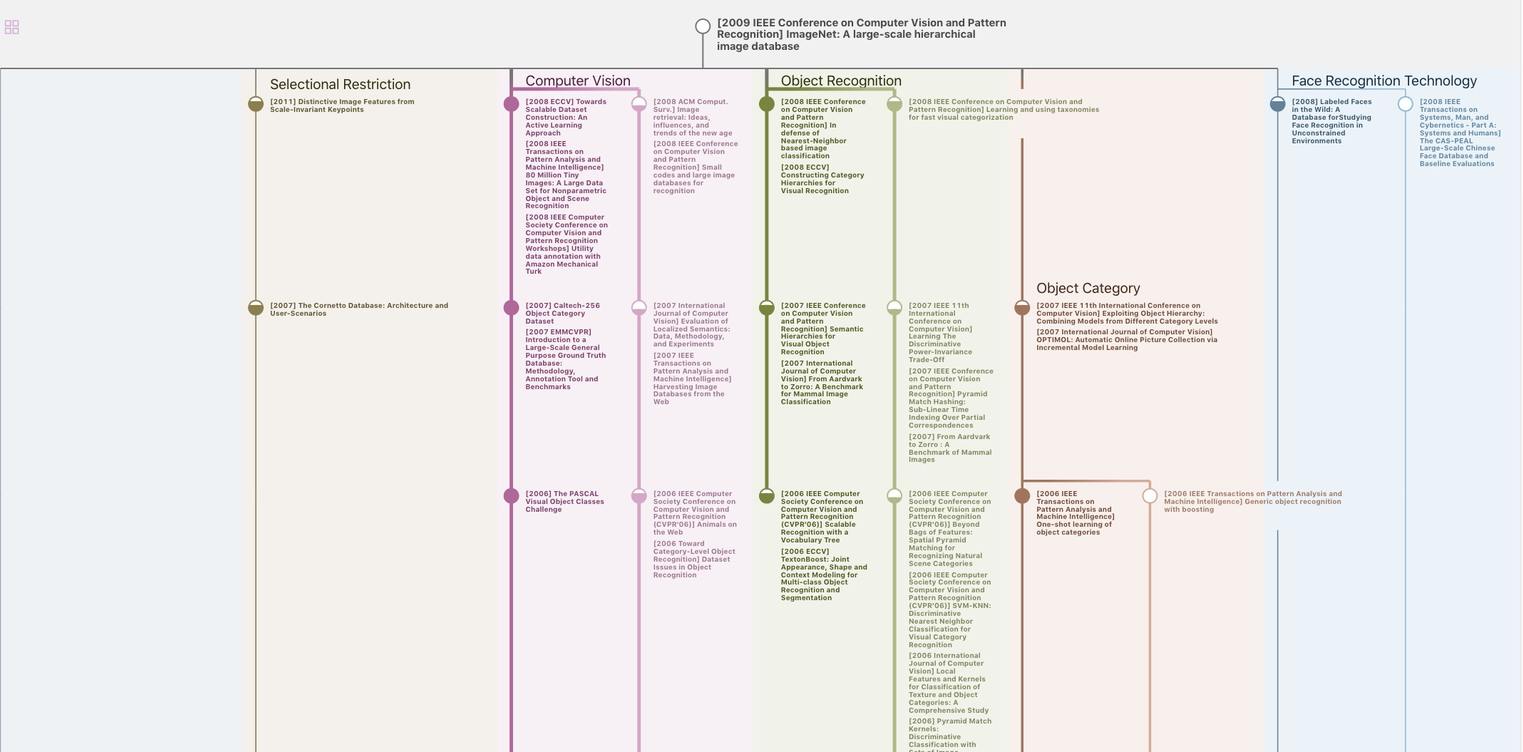
生成溯源树,研究论文发展脉络
Chat Paper
正在生成论文摘要