Non-invasive screening and subtyping for breast cancer by serum SERS combined with LGB-DNN algorithms
Talanta(2024)
摘要
Early detection of breast cancer and its molecular subtyping is crucial for guiding clinical treatment and improving survival rate. Current diagnostic methods for breast cancer are invasive, time consuming and complicated. In this work, an optical detection method integrating surface-enhanced Raman spectroscopy (SERS) technology with feature selection and deep learning algorithm was developed for identifying serum components and building diagnostic model, with the aim of efficient and accurate noninvasive screening of breast cancer. First, the high quality of serum SERS spectra from breast cancer (BC), breast benign disease (BBD) patients and healthy controls (HC) were obtained. Chi-square tests were conducted to exclude confounding factors, enhancing the reliability of the study. Then, LightGBM (LGB) algorithm was used as the base model to retain useful features to significantly improve classification performance. The DNN algorithm was trained through backpropagation, adjusting the weights and biases between neurons to improve the network's predictive ability. In comparison to traditional machine learning algorithms, this method provided more accurate information for breast cancer classification, with classification accuracies of 91.38% for BC and BBD, and 96.40% for BC, BBD, and HC. Furthermore, the accuracies of 90.11% for HR+/HR- and 88.89% for HER2+/HER2- can be reached when evaluating BC patients’ molecular subtypes. These results demonstrate that serum SERS combined with powerful LGB-DNN algorithm would provide a supplementary method for clinical breast cancer screening.
更多查看译文
关键词
Raman spectroscopy,Breast cancer,Feature selection,Deep neural network,Diagnostic model
AI 理解论文
溯源树
样例
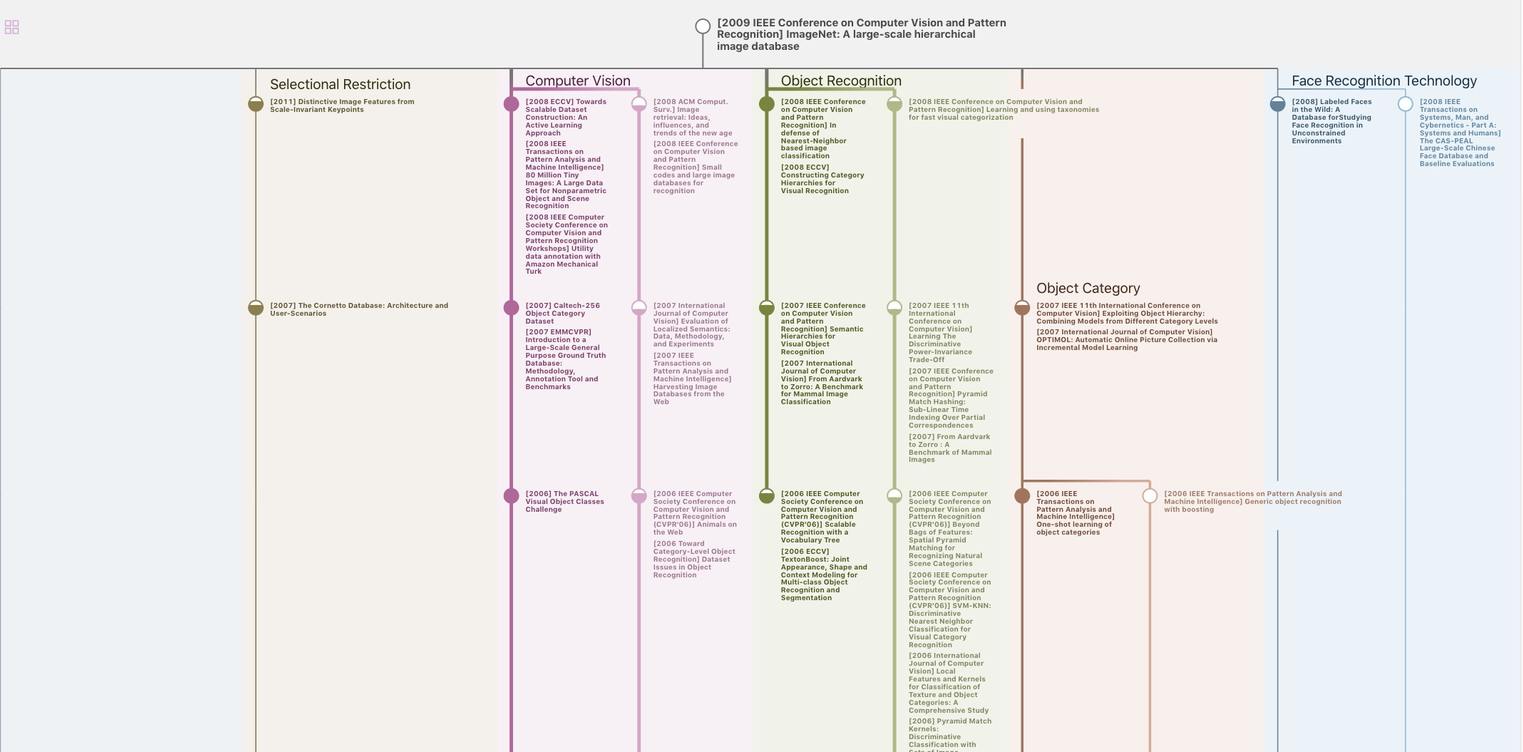
生成溯源树,研究论文发展脉络
Chat Paper
正在生成论文摘要