Estimating Empirical Ground-Motion Models including Spatial correlations with the Integrated Nested Laplace Approximation
crossref(2024)
摘要
A Bayesian method to include spatial correlation structure of residuals in empirical ground-motion models is presented, based on the integrated nested Laplace approximation. The method is evaluated on a simulated data set as well as Italian strong-motion data. Results based on the synthetic data set indicate that the parameters of the model, as well as associated uncertainties, are well captured. The presented method facilitates the use of more complex models, such as accounting for nonergodic source, path, and site effects, as well as non-stationary correlation functions. Excluding spatial correlations in the empirical GMM leads to an increased value of the between-event variability; however, the total variability tends to be the same. Ground-Motion Models that include spatial correlation lead to better predictive performance for within-event predictions. On the other hand, when predicting for new events, performance decreases slightly compared to models that do not account for spatial correlations.
更多查看译文
AI 理解论文
溯源树
样例
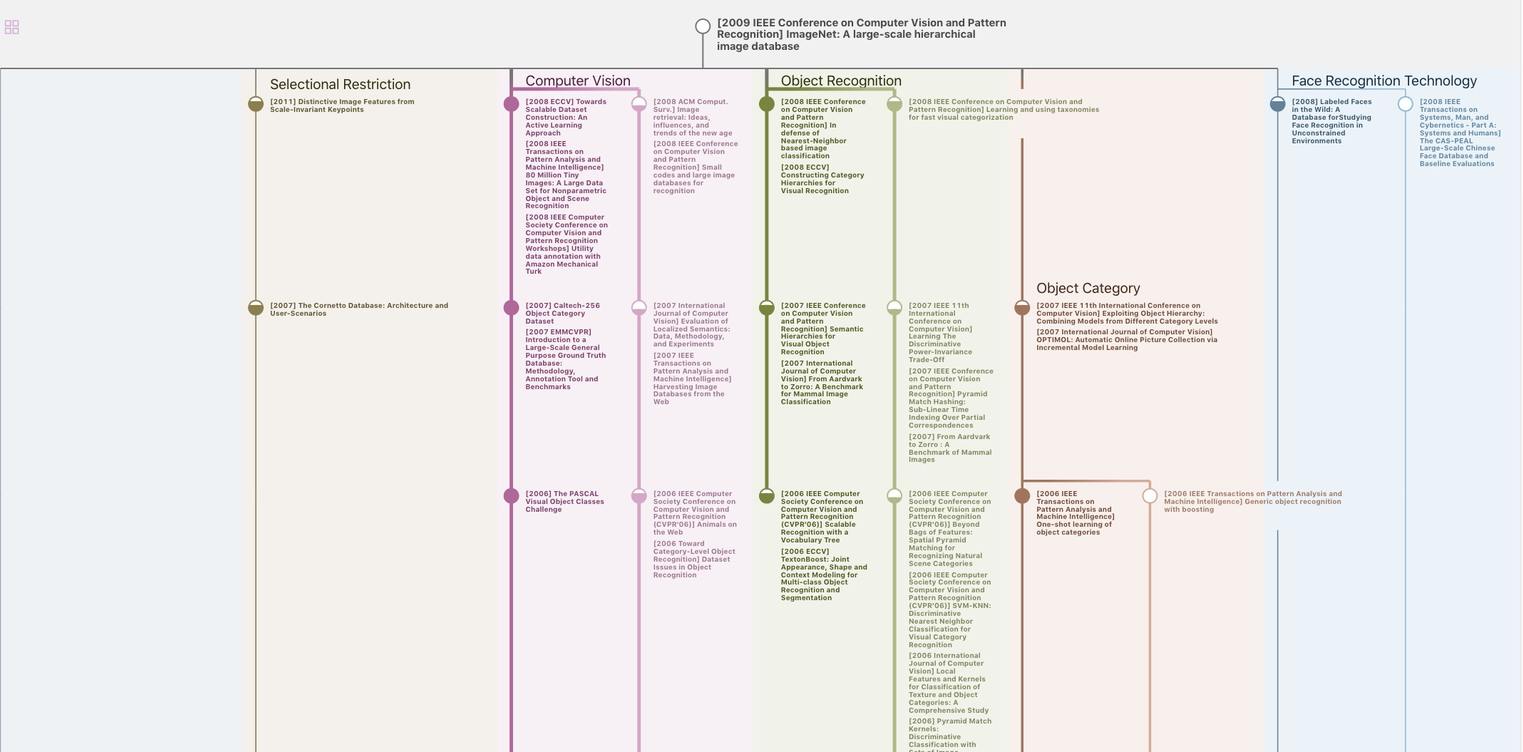
生成溯源树,研究论文发展脉络
Chat Paper
正在生成论文摘要