Large Language Models in Randomized Controlled Trials Design
crossref(2024)
摘要
Abstract We investigate the potential of large language models (LLMs) in enhancing the design of randomized controlled trials (RCTs) to address challenges related to generalizability, recruitment diversity, and failure rates. We selected 20 RCTs for analysis, including both completed and ongoing studies, with a focus on their design aspects such as eligibility criteria, recruitment strategies, interventions, and outcomes measurement. Our evaluation revealed that LLMs can design RCT with 72% overall accuracy. Qualitative assessments indicated that LLM-generated designs were clinically aligned, scoring above 2 on a Likert scale across safety, accuracy, objectivity, pragmatism, inclusivity, and diversity domains. The results highlight LLM's capability to avoid critical safety and ethical issues, suggesting its potential as an assistive tool in RCT design to improve generalizability and reduce failure rates. However, expert oversight and regulatory measures are emphasized as essential to ensure patient safety and ethical conduct in clinical research.
更多查看译文
AI 理解论文
溯源树
样例
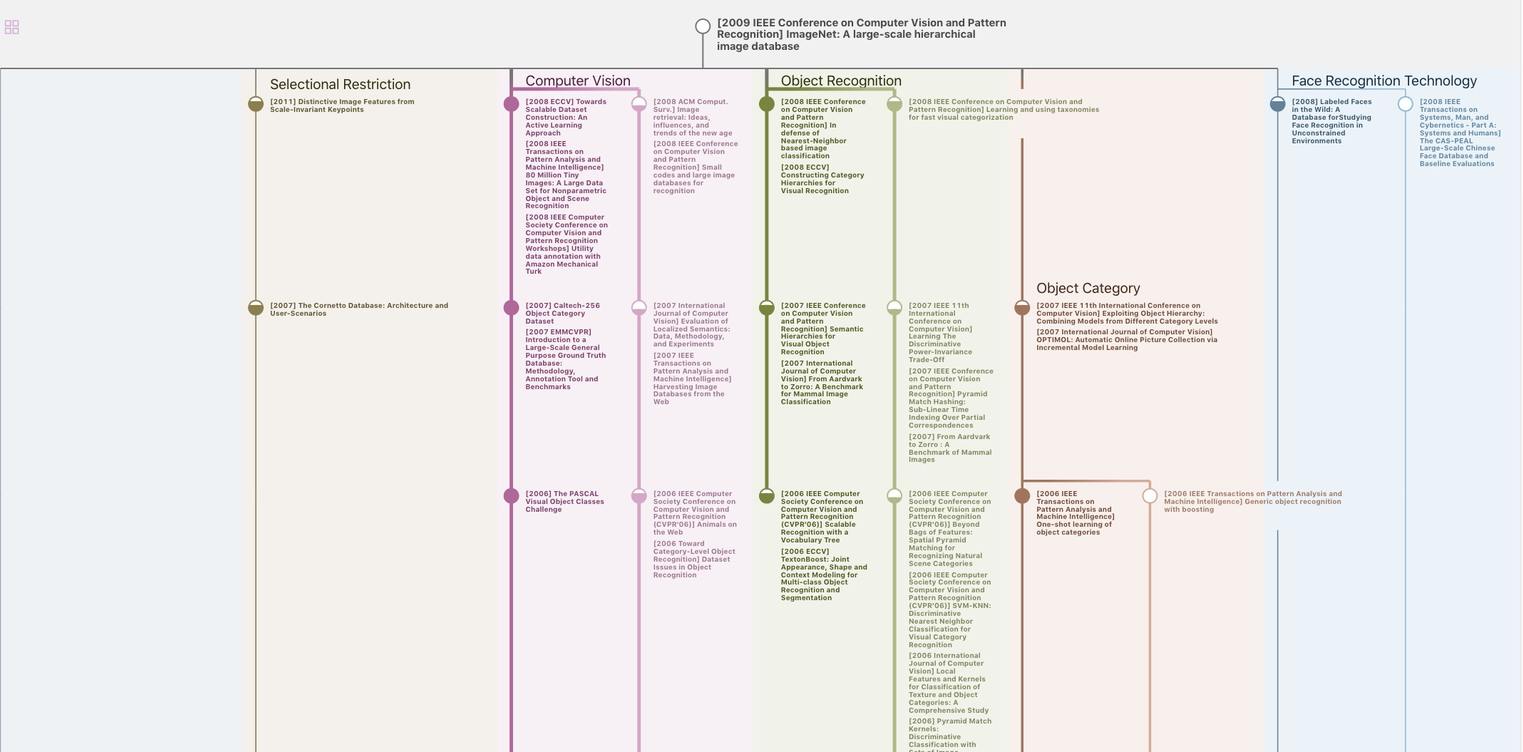
生成溯源树,研究论文发展脉络
Chat Paper
正在生成论文摘要