Interest Point Selection and Feature Extraction in Six-DoF Grasp Pose Detection
IEEE-ASME TRANSACTIONS ON MECHATRONICS(2024)
摘要
Six-DoF grasp detection has attracted increasing attention due to its high flexibility in manipulation tasks. Recent learning-based methods typically use point sampling or filtering to extract a small subset of scene points for grasp pose computation. However, the sampling method disregards the fact that each scene point contributes differently to the grasp, while the filtering method nearly destroys the geometrical structure of the scene. Concurrently, indirectly learning point features by inputting point coordinates to the network is insufficient for detecting grasp poses since this task heavily depends on the geometrical structure of the local point clouds. In order to solve the above-mentioned problems, we design a novel interest point selection algorithm in the grasp scene, and a novel explicit geometrical feature extraction algorithm for local point clouds in the six-DoF grasp pose detection task. We include the basic grasp points, nearby object points and background points as interest points in calculating the grasp poses. Moreover, we add a module that predicts grasp collision within the network to eliminate the time-consuming collision detection in postprocessing. The proposed network is trained and evaluated on the GraspNet1Billion dataset, and achieves state-of-the-art performance. We also conduct an ablation study to validate the proposed algorithms and the network. Furthermore, we successfully implement our network on a UR5e robotic arm to demonstrate its practical applicability in both single-object and multiobject grasp scenarios.
更多查看译文
关键词
Six-DoF grasp detection,interest point selection,point feature extraction,robotic grasp
AI 理解论文
溯源树
样例
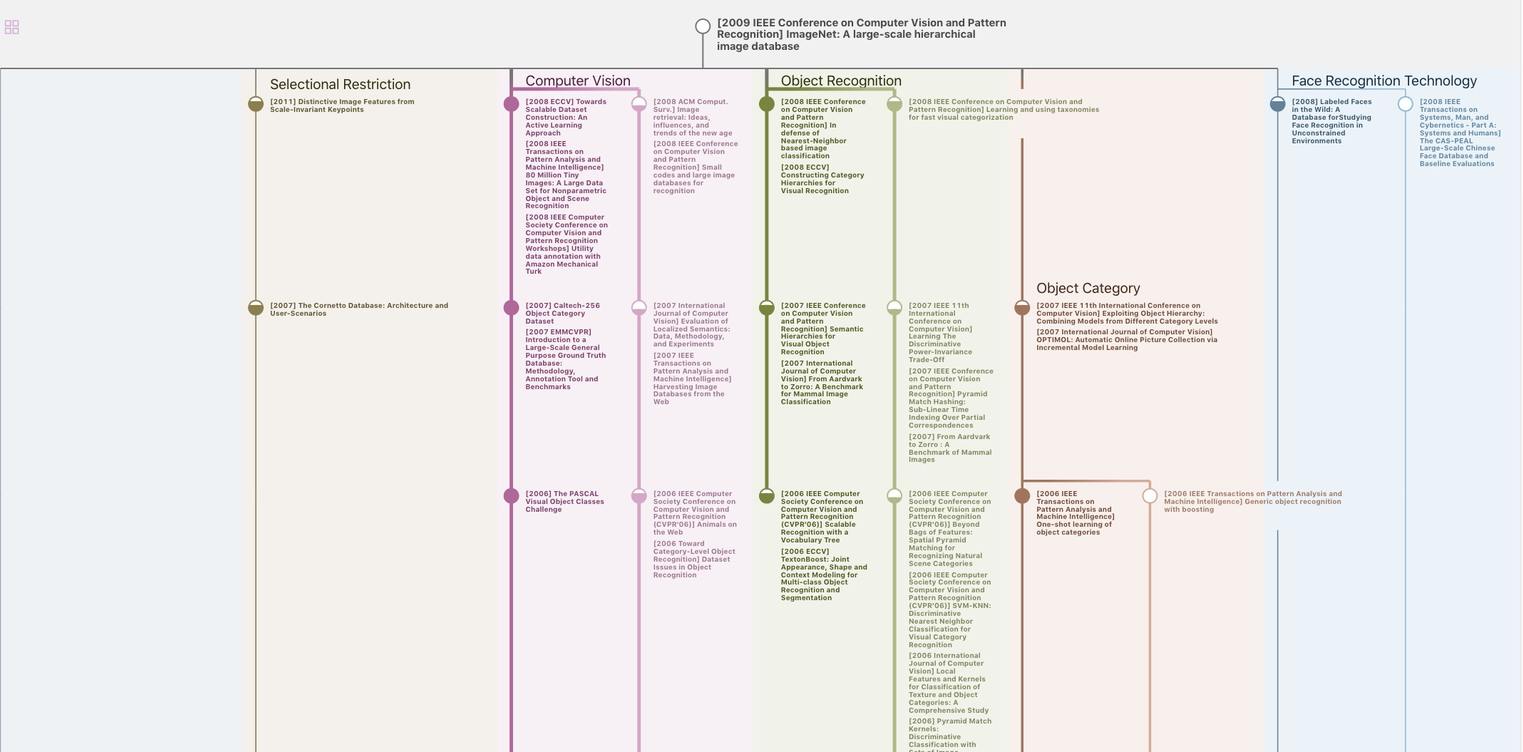
生成溯源树,研究论文发展脉络
Chat Paper
正在生成论文摘要