Boosting computational thermodynamic analysis of the CVD of SiC coating via machine learning
Journal of Crystal Growth(2024)
摘要
Although thermodynamic analysis provides the possibility to perform a full-range study on the chemical vapor deposition (CVD) of SiC, billions of calculations are required to achieve a high-resolution study for such a high-dimensional space. Similar issues are also encountered for other materials modeling and simulation techniques. Therefore, using the database from the thermodynamic analysis of CVD of SiC as an example, we demonstrate a machine learning framework to accelerate physical-based materials modeling and simulations. Using only 6 % of randomly selected data generated in the thermodynamic analysis of CVD of SiC, a high-fidelity random forest (RF) with an accuracy (R2) of close to 1 was obtained. The derived key CVD metrics, i.e., deposit purity, deposition efficiency of SiC, and precursor utilization efficiency by the RF model are in excellent agreement with those determined by thermodynamic analysis.
更多查看译文
关键词
Machine learning,CVD,SiC,Computational thermodynamics
AI 理解论文
溯源树
样例
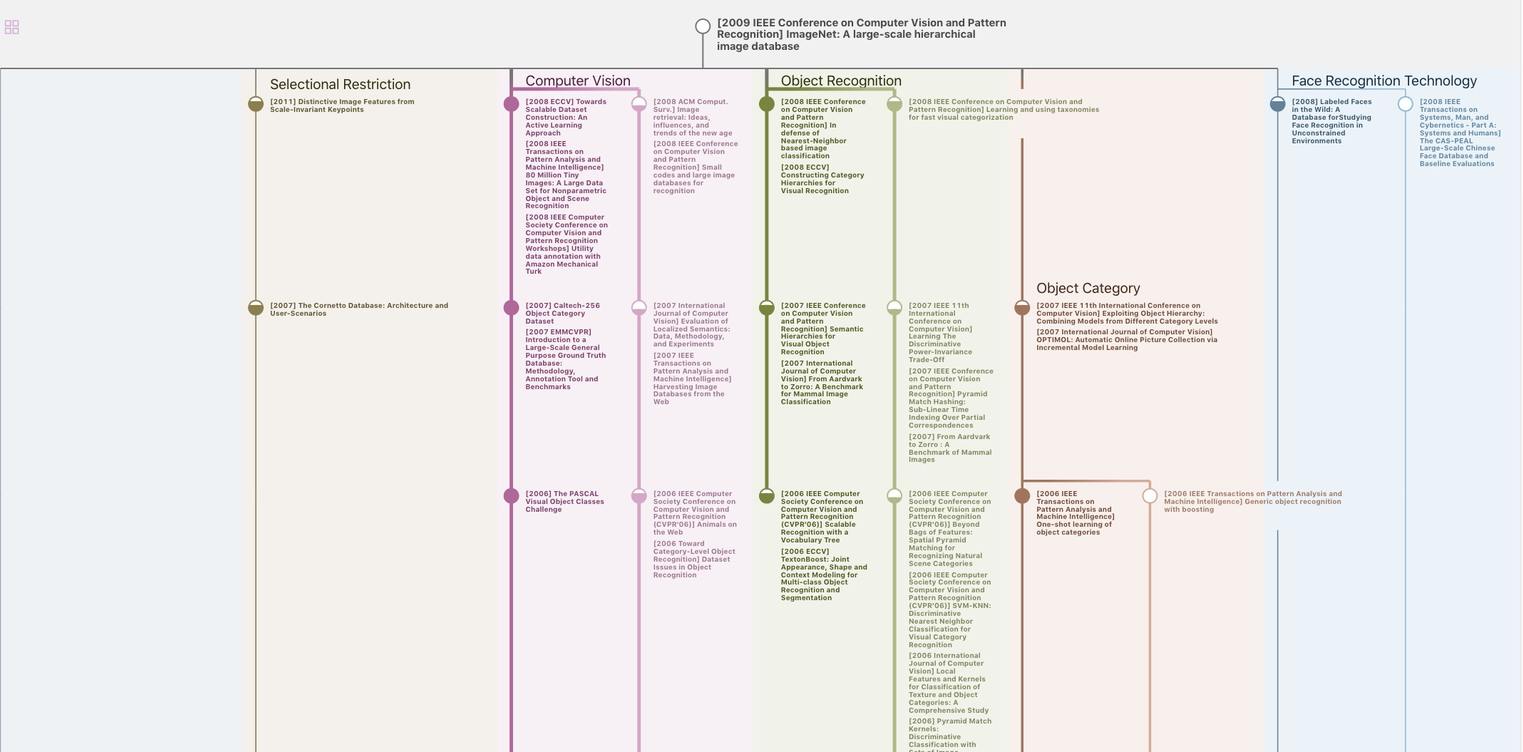
生成溯源树,研究论文发展脉络
Chat Paper
正在生成论文摘要