Detection of bladder cancer cells using quantitative interferometric label-free imaging flow cytometry
CYTOMETRY PART A(2024)
摘要
Bladder cancer is one of the most common cancers with a high recurrence rate. Patients undergo mandatory yearly scrutinies, including cystoscopies, which makes bladder cancer highly distressing and costly. Here, we aim to develop a non-invasive, label-free method for the detection of bladder cancer cells in urine samples, which is based on interferometric imaging flow cytometry. Eight urothelial carcinoma and one normal urothelial cell lines, along with red and white blood cells, imaged quantitatively without staining by an interferometric phase microscopy module while flowing in a microfluidic chip, and classified by two machine-learning algorithms, based on deep-learning semantic segmentation convolutional neural network and extreme gradient boosting. Furthermore, urine samples obtained from bladder-cancer patients and healthy volunteers were imaged, and classified by the system. We achieved accuracy and area under the curve (AUC) of 99% and 97% for the cell lines on both machine-learning algorithms. For the real urine samples, the accuracy and AUC were 96% and 96% for the deep-learning algorithm and 95% and 93% for the gradient-boosting algorithm, respectively. By combining label-free interferometric imaging flow cytometry with high-end classification algorithms, we achieved high-performance differentiation between healthy and malignant cells. The proposed technique has the potential to supplant cystoscopy in the bladder cancer surveillance and diagnosis space.
更多查看译文
关键词
bladder,cancer,classification,detection,interferometry,noninvasive
AI 理解论文
溯源树
样例
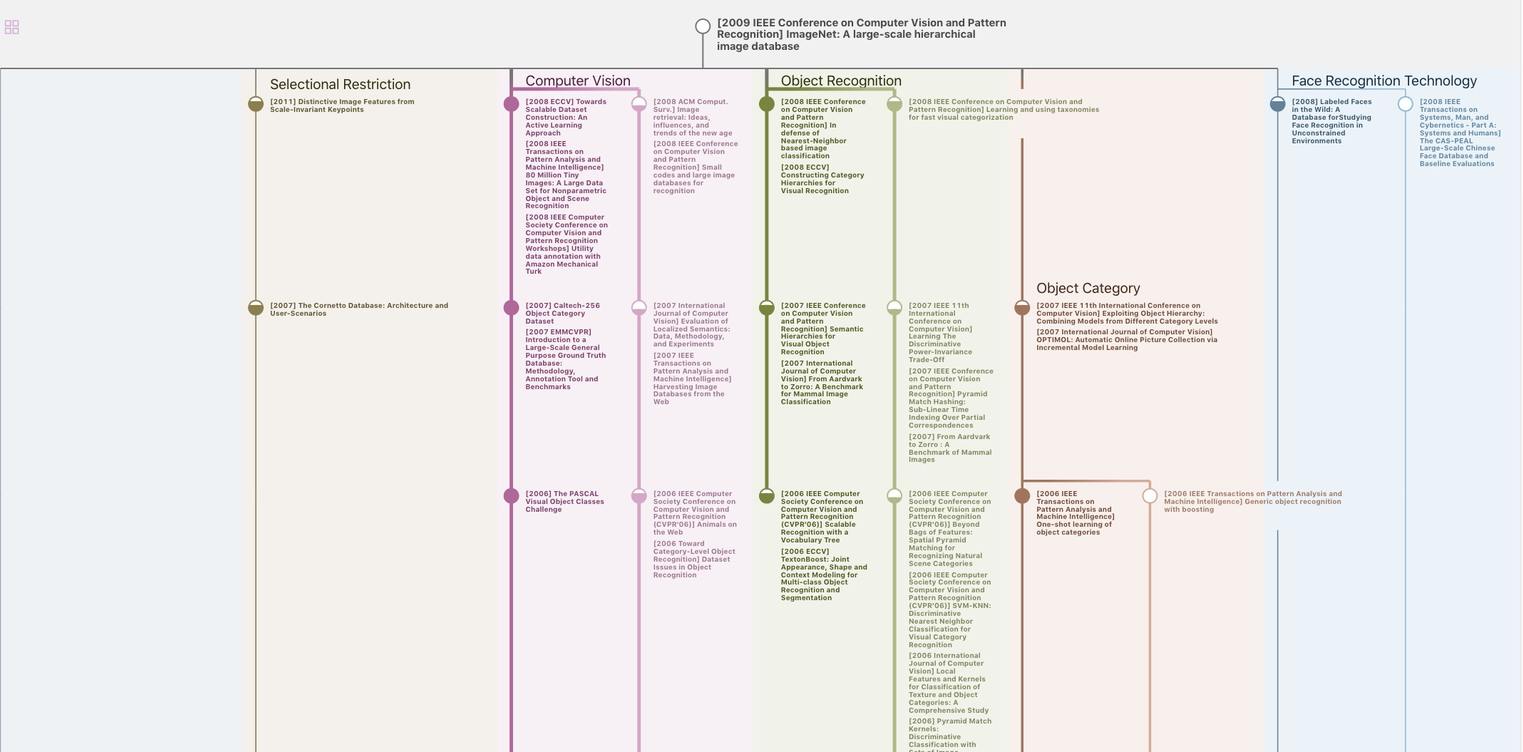
生成溯源树,研究论文发展脉络
Chat Paper
正在生成论文摘要