Self-Supervised State Calculation for Distribution Network via Masked Graph Reconstruction
2023 5th International Academic Exchange Conference on Science and Technology Innovation (IAECST)(2023)
摘要
With the continuous growth of power demand, the scale and complexity of distribution networks are also increasing. Therefore, accurate and efficient calculation of the status of distribution networks is of great significance for the safe, stable, and economic operation of power systems. Traditional distribution network status calculation methods are usually based on physical models of the power system, require a large amount of mathematical calculation and simulation analysis, and are difficult to meet the real-time requirements of modern power systems. To address this issue, this paper proposes a distribution network status calculation method based on heterogeneous graph mask modeling. This method utilizes a heterogeneous graph neural network to model the distribution network, represents the nodes and edges of the distribution network as nodes and edges in the graph, and utilizes graph mask technology to reconstruct node attributes and edges in self-supervised manner. Through a pre-trained graph neural network model, the status of the distribution network can be quickly and accurately calculated. Experimental results show that this method can accurately model the topological relationship and state variables of the distribution network, demonstrating high accuracy on downstream tasks. Compared with traditional distribution network status calculation methods, it has stronger adaptability and scalability.
更多查看译文
关键词
distribution network,state calculation,graph,mask reconstruction,self-supervised
AI 理解论文
溯源树
样例
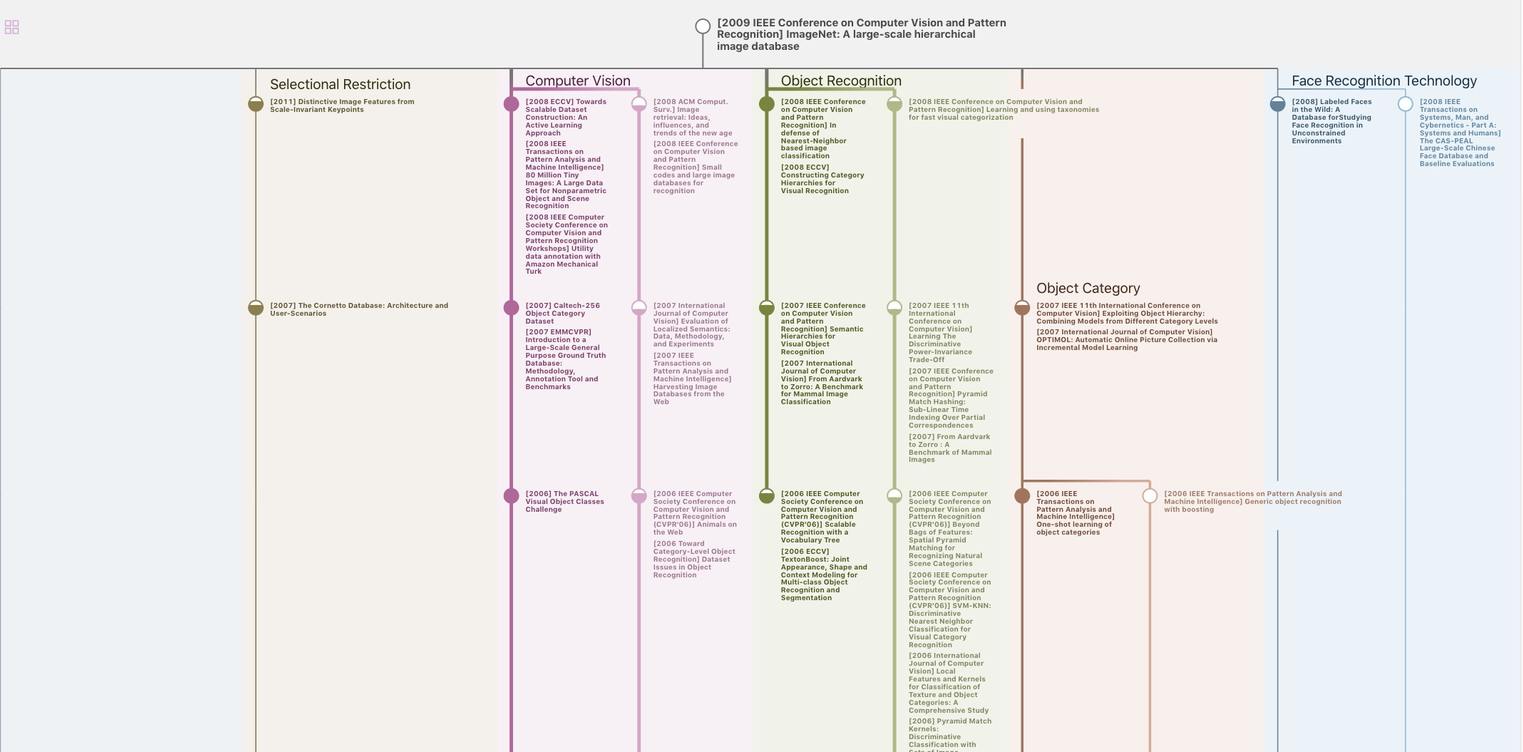
生成溯源树,研究论文发展脉络
Chat Paper
正在生成论文摘要