Deep Learning-Based Raman Spectroscopy Qualitative Analysis Algorithm: A Convolutional Neural Network and Transformer Approach
Talanta(2024)
摘要
Raman spectroscopy is a general and non-destructive detection technique that can obtain detailed information of the chemical structure of materials. In the past, when using chemometric algorithms to analyze the Raman spectra of mixtures, the challenges of complex spectral overlap and noise often limited the accurate identification of components. The emergence of deep learning has introduced a novel approach to qualitative analysis of mixed Raman spectra. In this paper, we propose a deep learning-based Raman spectroscopy qualitative analysis algorithm (RST) by borrowing the ideas of convolutional neural network and Transformer. By transforming the Raman spectrum into 64 word vectors, the contribution weights of each word vector to the components are obtained. For the 75 spectral data used for validation, the positive identification rate can reach 100.00%, the recall rate can reach 99.3%, the average identification score can reach 9.51, and it is applicable to the fields of Raman and surface-enhanced Raman spectroscopy. Furthermore, compared with traditional CNN models, RST has excellent accuracy and robustness in identifying components in complex mixtures. The model's interpretability has been enhanced, aiding in a deeper understanding of spectroscopic learning patterns for future analysis of more complex mixtures.
更多查看译文
关键词
Raman spectroscopy,Deep learning,Transformer,Mixtures,qualitative analysis
AI 理解论文
溯源树
样例
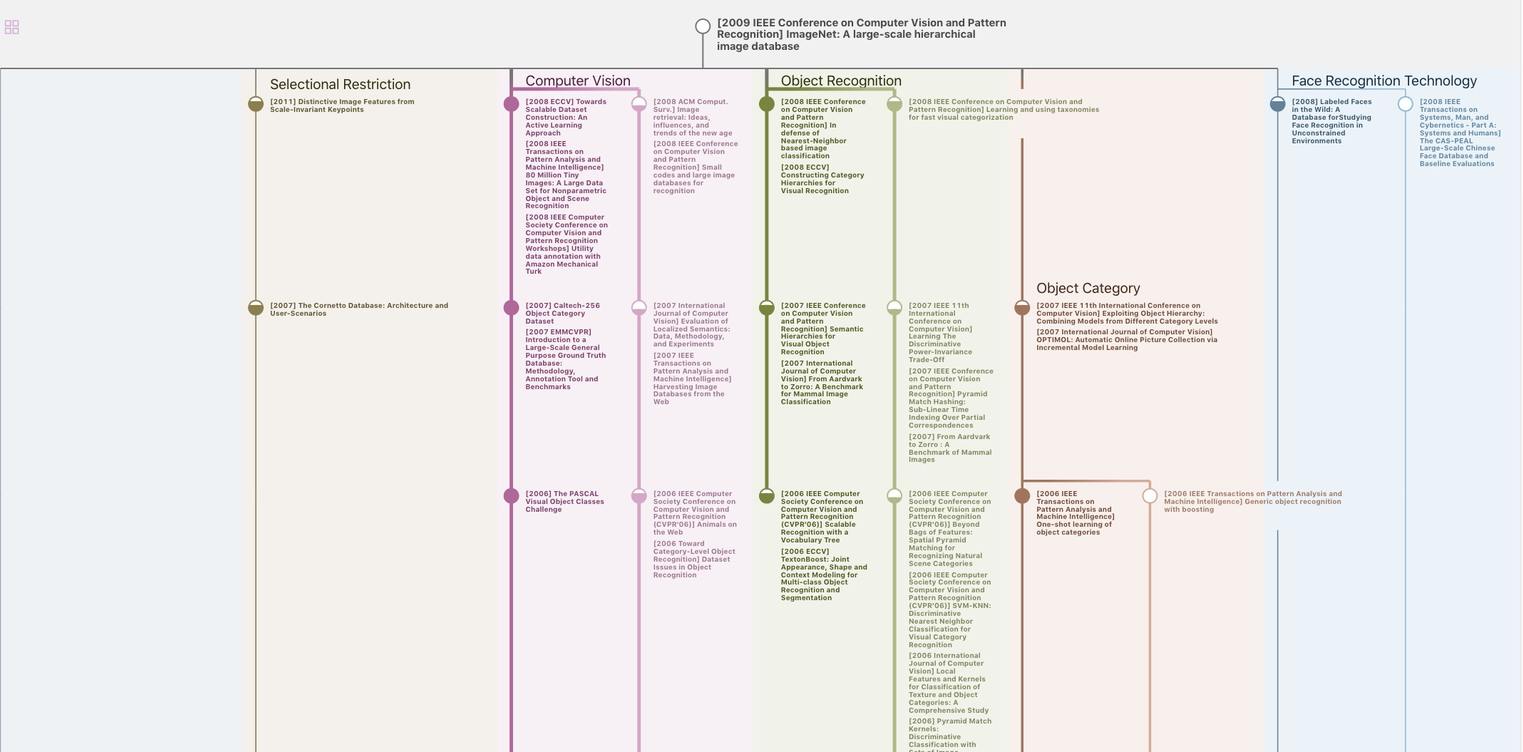
生成溯源树,研究论文发展脉络
Chat Paper
正在生成论文摘要