Multi-Label Action Anticipation for Real-World Videos with Scene Understanding.
IEEE transactions on image processing : a publication of the IEEE Signal Processing Society(2024)
摘要
With human action anticipation becoming an essential tool for many practical applications, there has been an increasing trend in developing more accurate anticipation models in recent years. Most of the existing methods target standard action anticipation datasets, in which they could produce promising results by learning action-level contextual patterns. However, the over-simplified scenarios of standard datasets often do not hold in reality, which hinders them from being applied to real-world applications. To address this, we propose a scene-graph-based novel model SEAD that learns the action anticipation at the high semantic level rather than focusing on the action level. The proposed model is composed of two main modules, 1) the scene prediction module, which predicts future scene graphs using a grammar dictionary, and 2) the action anticipation module, which is responsible for predicting future actions with an LSTM network by taking as input the observed and predicted scene graphs. We evaluate our model on two real-world video datasets (Charades and Home Action Genome) as well as a standard action anticipation dataset (CAD-120) to verify its efficacy. The experimental results show that SEAD is able to outperform existing methods by large margins on the two real-world datasets and can also yield stable predictions on the standard dataset at the same time. In particular, our proposed model surpasses the state-of-the-art methods with mean average precision improvements consistently higher than 65% on the Charades dataset and an average improvement of 40.6% on the Home Action Genome dataset.
更多查看译文
关键词
Action anticipation,real-world datasets,scene graph,stochastic grammar
AI 理解论文
溯源树
样例
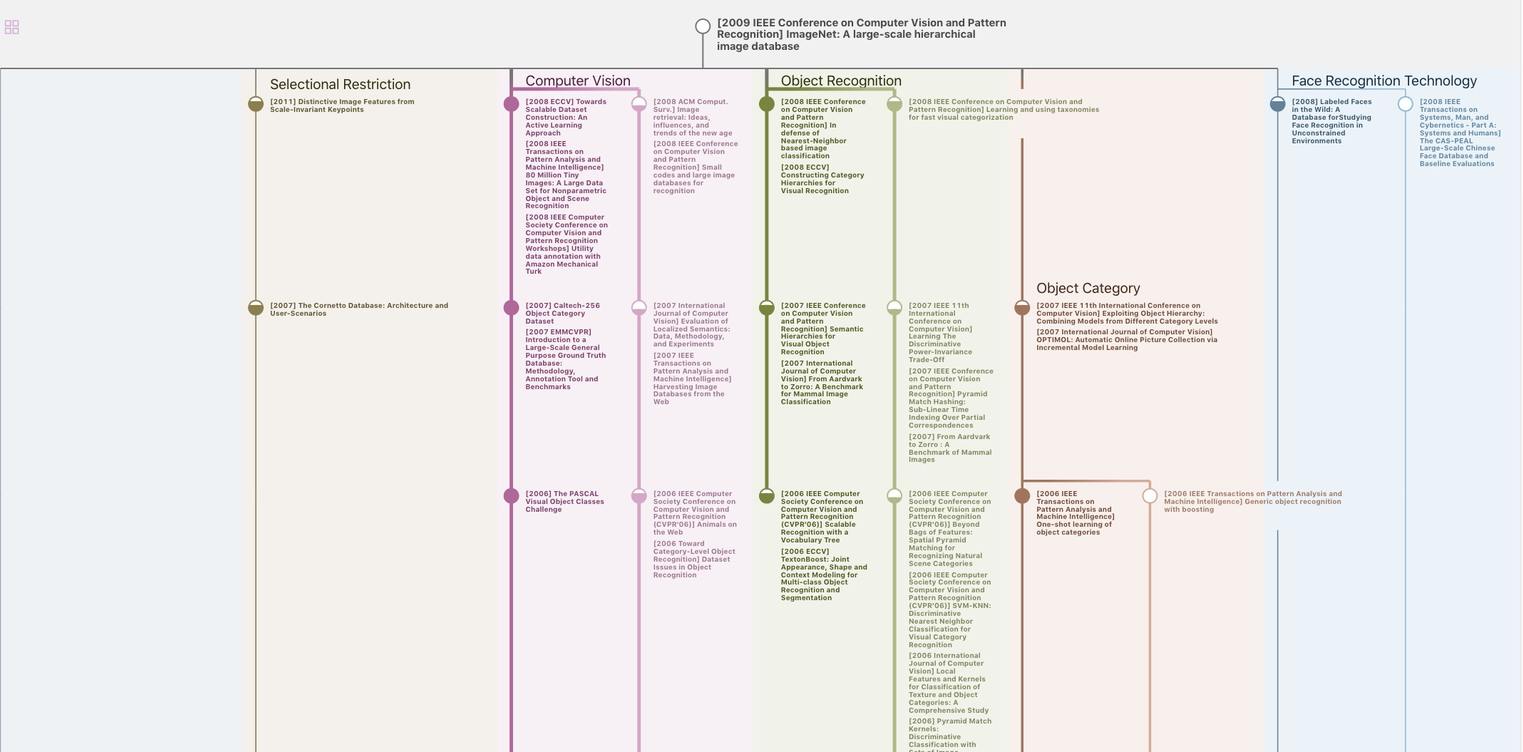
生成溯源树,研究论文发展脉络
Chat Paper
正在生成论文摘要