Hybrid quantum physics-informed neural networks for simulating computational fluid dynamics in complex shapes
Machine Learning: Science and Technology(2024)
摘要
Finding the distribution of the velocities and pressures of a fluid by solving the Navier–Stokes equations is a principal task in the chemical, energy, and pharmaceutical industries, as well as in mechanical engineering and in design of pipeline systems. With existing solvers, such as OpenFOAM and Ansys, simulations of fluid dynamics in intricate geometries are computationally expensive and require re-simulation whenever the geometric parameters or the initial and boundary conditions are altered. Physics-informed neural networks (PINNs) are a promising tool for simulating fluid flows in complex geometries, as they can adapt to changes in the geometry and mesh definitions, allowing for generalization across fluid parameters and transfer learning across different shapes. We present a hybrid quantum PINN (HQPINN) that simulates laminar fluid flow in 3D Y -shaped mixers. Our approach combines the expressive power of a quantum model with the flexibility of a PINN, resulting in a 21% higher accuracy compared to a purely classical neural network. Our findings highlight the potential of machine learning approaches, and in particular HQPINN, for complex shape optimization tasks in computational fluid dynamics. By improving the accuracy of fluid simulations in complex geometries, our research using hybrid quantum models contributes to the development of more efficient and reliable fluid dynamics solvers.
更多查看译文
关键词
quantum machine learning,physics-informed neural networks,computational fluid dynamics,3D simulation,Navier–Stokes equations,hybrid quantum neural networks
AI 理解论文
溯源树
样例
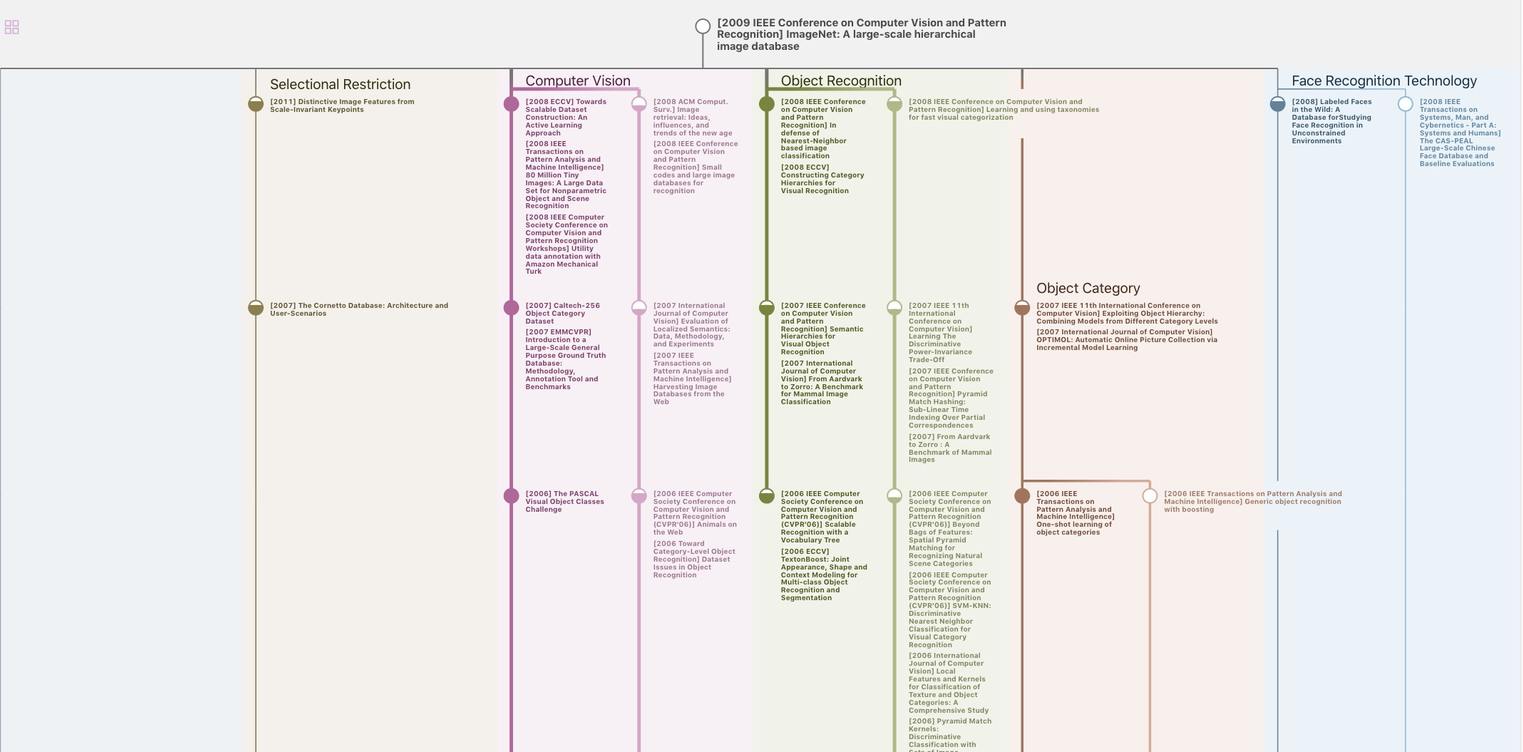
生成溯源树,研究论文发展脉络
Chat Paper
正在生成论文摘要