Anomaly detection framework for highly scattered and dynamic data on large-scale networks using AWS
International Journal of Information Technology(2024)
摘要
Network anomaly detection is crucial for securing computer networks and communications. However, handling highly scattered data in large-scale, dynamic networks poses challenges, leading to increased false positives and false negatives in anomaly identification. To address this, a new anomaly detection framework employing ensemble learning techniques was introduced. This framework uses decision trees for scattered data and gradient-boosting algorithms for accuracy in dynamic network behaviors. It successfully identifies known and unknown abnormalities while ensuring scalability and reducing false positives and negatives. The framework was tested on CICIDS2017, NSLKDD, and KDDCUP99 datasets, achieving outstanding accuracy rates of 100
更多查看译文
关键词
Anomaly detection,Decision tree,Ensemble learning,Gradient decent,Regularization
AI 理解论文
溯源树
样例
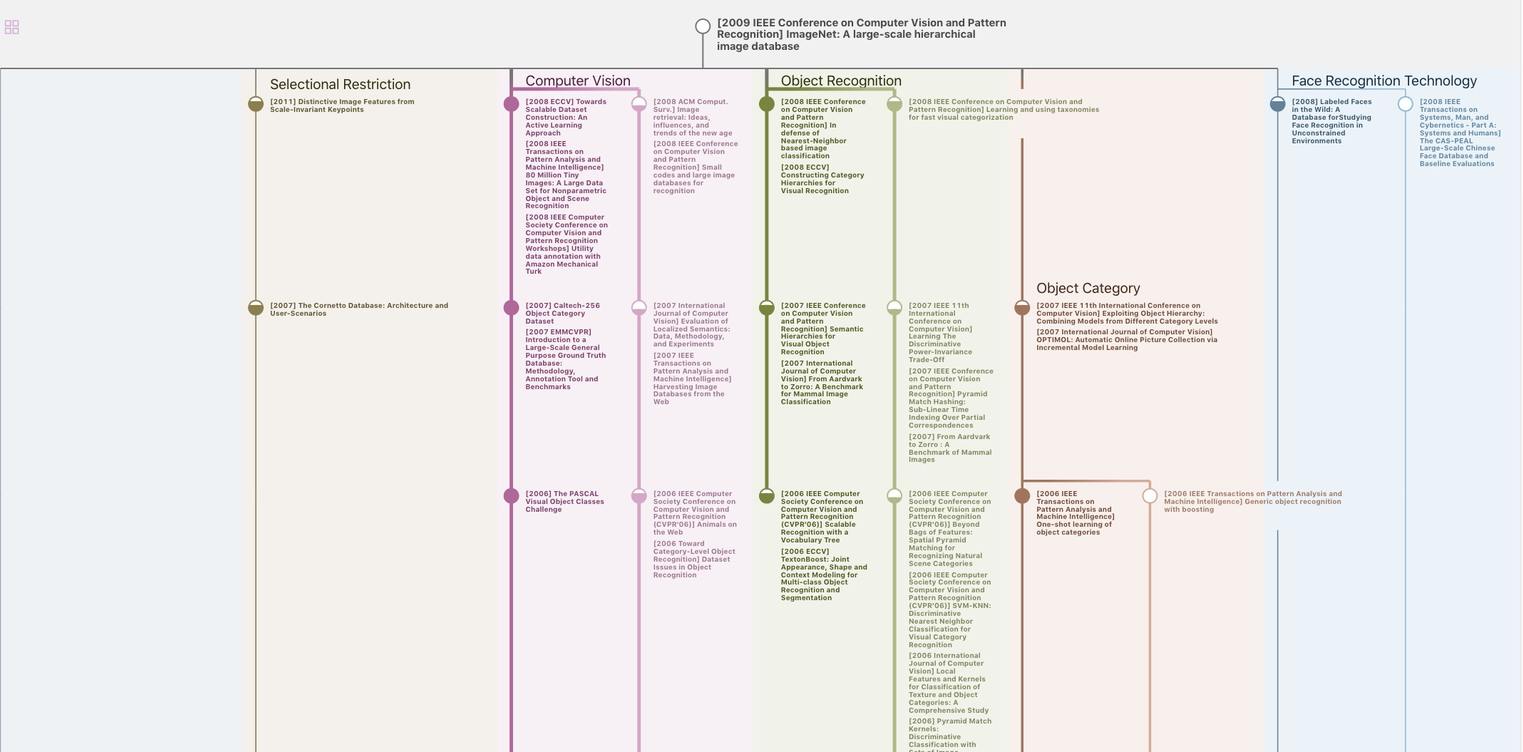
生成溯源树,研究论文发展脉络
Chat Paper
正在生成论文摘要