A classification framework with multi-level fusion of object-based analysis and convolutional neural network: a case study for land use classification in mining areas
Geo-spatial Information Science(2024)
摘要
ABSTRACTTo enhance the accuracy of land use classification in mining areas, the Object-based Convolutional Neural Network (OCNN) method has been widely used. However, existing researches tend to neglect the importance of decision-level fusion, focusing only on feature-level fusion. This study proposes a new classification framework with Multi-level Fusion of Object-based analysis and CNN (MFOCNN) to achieves high-accuracy land use classification in mining areas. First, Simple Linear Iterative Cluster (SLIC) is employed to generate image objects, which serve as the basic unit for classification. Second, an improved DenseNet is proposed to extract deep features from image patches, which represent image objects, and provide the classification result. Third, handcrafted features including spectral, textural and geometric of the image objects are extracted and fused with the deep features to obtain the classification result with random forest classifier. Finally, the Dempster-Shafer (DS) evidence theory is applied to fuse the two previously described classification results at the decision-level to obtain the final result. Experiments conducted in the mining areas of Erdos using Gaofen-6 images demonstrate that the proposed MFOCNN achieves the best visual performance and accuracy among all tested methods. The MFOCNN, with its feature-level fusion and decision-level fusion, significantly improves the accuracy of land use classification in mining areas. The results suggest that the proposed MFOCNN is a promising method for achieving high-accuracy land use classification in mining areas.
更多查看译文
关键词
Mining areas,land use classification,object-based analysis,DenseNet
AI 理解论文
溯源树
样例
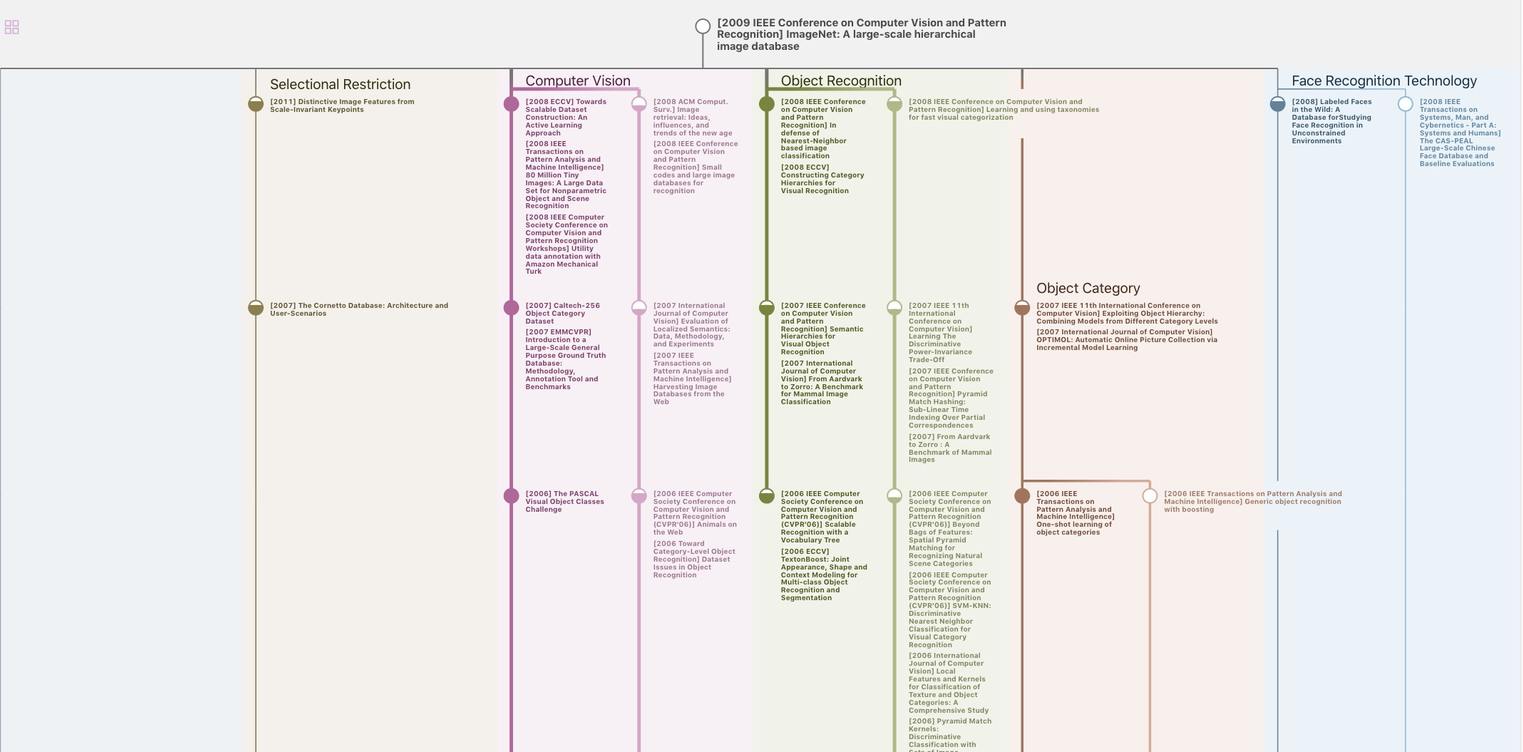
生成溯源树,研究论文发展脉络
Chat Paper
正在生成论文摘要