Federated vs. Central Machine Learning on Diabetic Foot Ulcer Images: Comparative Simulations
IEEE Access(2024)
摘要
This research examines the implementation of the U-Net model within a federated learning framework, focusing on the semantic segmentation of Diabetic Foot Ulcers (DFUs) images. The objective is to start with a set of random parameters for a U-Net model and train in a federated learning setting and a centralized setting. Due to the sensitive nature of medical images, we use an open-source dataset of diabetic foot ulcers provided by Medetec. Federated learning enables us to decentralize our approach to machine learning, which eliminates the need for centralizing raw data. Methods used include comparative simulations between federated and centralized machine learning systems. The results indicate that federated learning, combined with the U-Net architecture, eliminates centralized data collection and achieves a notable dice score of 0.9, paralleling the performance of centralized models. This conclusion underscores the potential of federated learning in enhancing detection methods for DFUs, balancing privacy concerns with analytical accuracy. Significantly, this study contributes to the biomedical imaging field by providing a set of federated learning codebases that enable interested researchers to reproduce the results and expand upon them. The source code can be accessed here [35].
更多查看译文
关键词
Comparative Simulations,Deep Learning,Diabetic Foot Ulcer,Federated Learning,U-Net Model
AI 理解论文
溯源树
样例
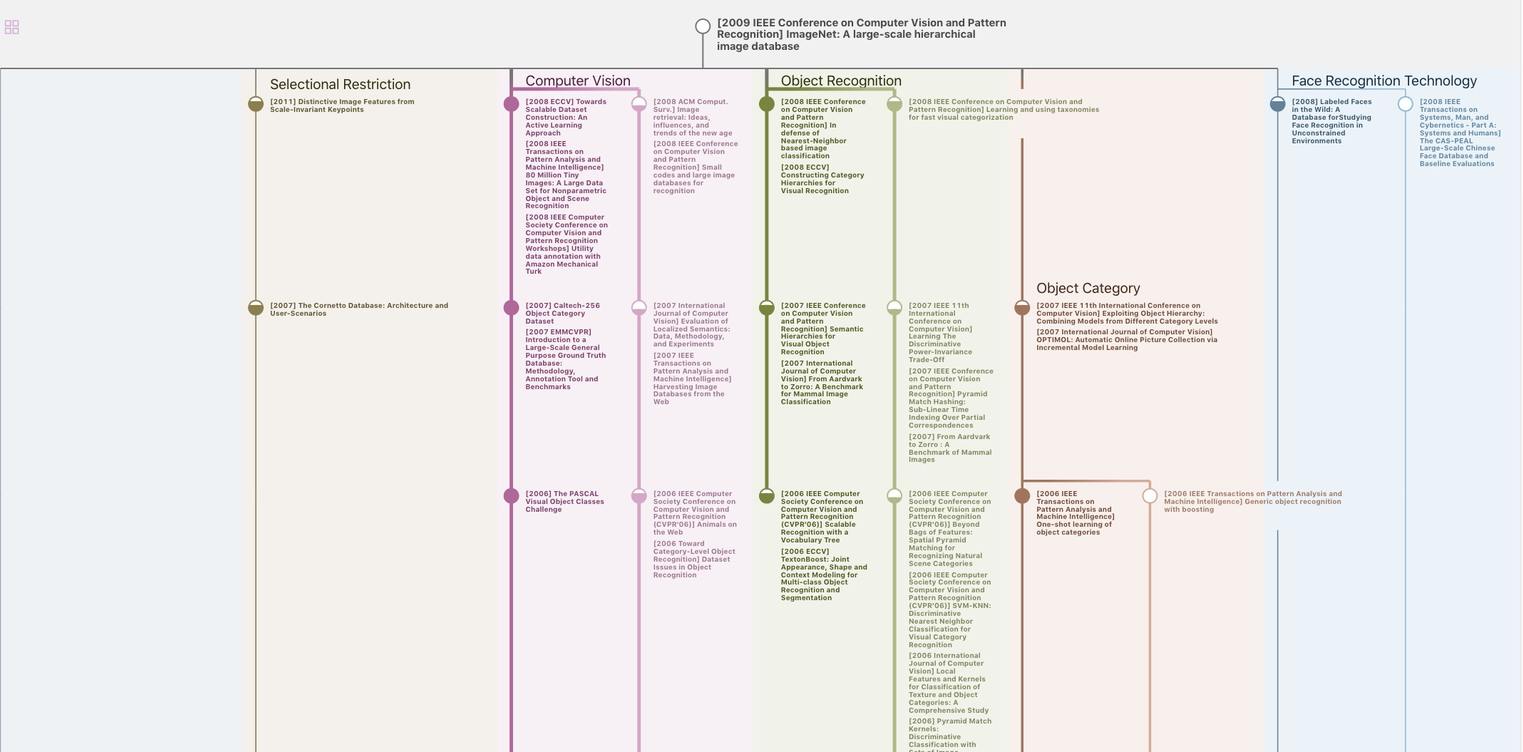
生成溯源树,研究论文发展脉络
Chat Paper
正在生成论文摘要