Multi-Stage Network with Geometric Semantic Attention for Two-View Correspondence Learning.
IEEE transactions on image processing : a publication of the IEEE Signal Processing Society(2024)
摘要
The removal of outliers is crucial for establishing correspondence between two images. However, when the proportion of outliers reaches nearly 90%, the task becomes highly challenging. Existing methods face limitations in effectively utilizing geometric transformation consistency (GTC) information and incorporating geometric semantic neighboring information. To address these challenges, we propose a Multi-Stage Geometric Semantic Attention (MSGSA) network. The MSGSA network consists of three key modules: the multi-branch (MB) module, the GTC module, and the geometric semantic attention (GSA) module. The MB module, structured with a multi-branch design, facilitates diverse and robust spatial transformations. The GTC module captures transformation consistency information from the preceding stage. The GSA module categorizes input based on the prior stage's output, enabling efficient extraction of geometric semantic information through a graph-based representation and inter-category information interaction using Transformer. Extensive experiments on the YFCC100M and SUN3D datasets demonstrate that MSGSA outperforms current state-of-the-art methods in outlier removal and camera pose estimation, particularly in scenarios with a high prevalence of outliers. Source code is available at https://github.com/shuyuanlin.
更多查看译文
关键词
Correspondence learning,feature matching,outlier removal,camera pose estimation,deep learning
AI 理解论文
溯源树
样例
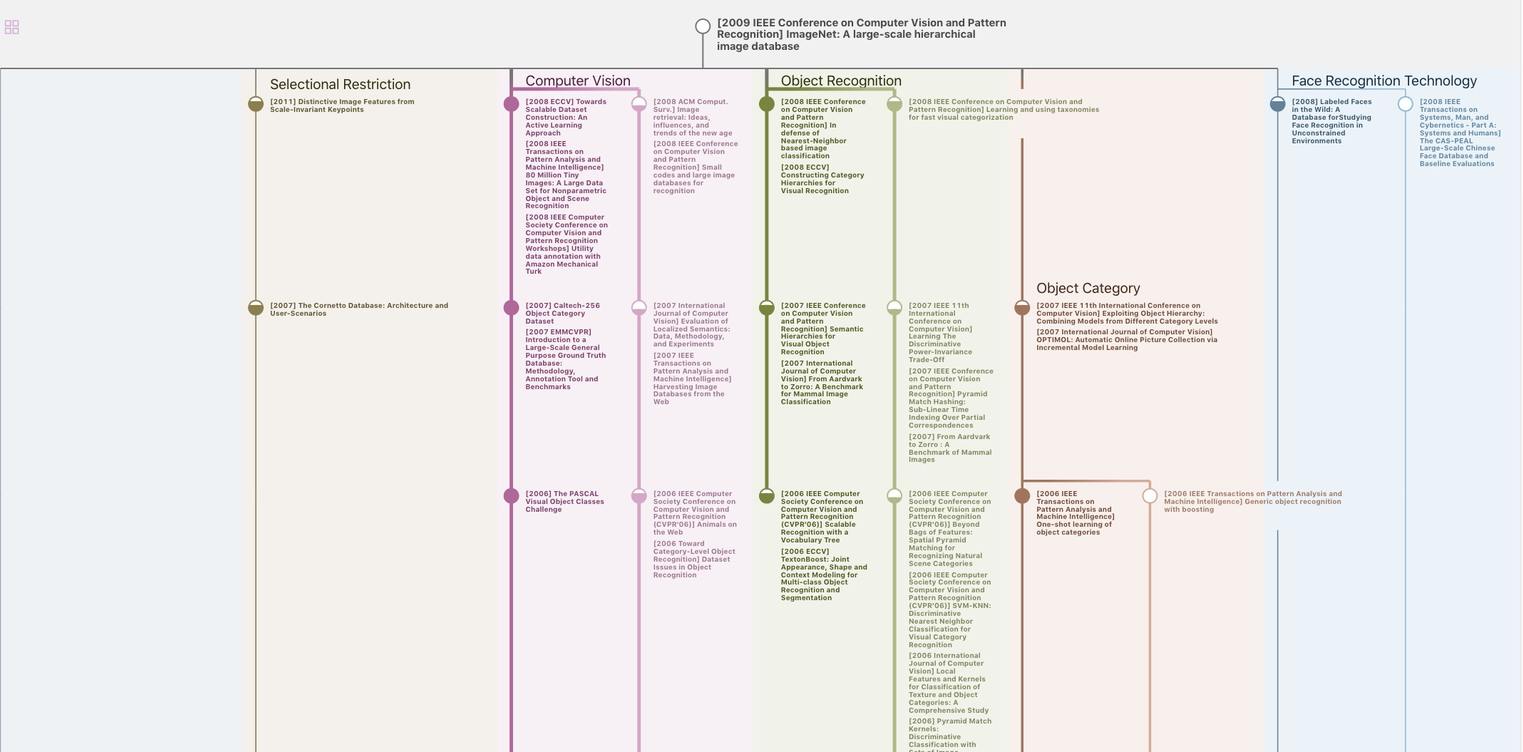
生成溯源树,研究论文发展脉络
Chat Paper
正在生成论文摘要