Use of CT-derived Radiomic Features to Preoperatively Identify Invasive Mucinous Adenocarcinoma in Solitary Pulmonary Nodules ≤3 cm
Heliyon(2024)
摘要
Objective
In this study, we aimed to utilize computed tomography (CT)-derived radiomics and various machine learning approaches to differentiate between invasive mucinous adenocarcinoma (IMA) and invasive non-mucinous adenocarcinoma (INMA) preoperatively in solitary pulmonary nodules (SPN) ≤3 cm.
Methods
A total of 538 patients with SPNs measuring ≤3 cm were enrolled, categorized into either the IMA group (n = 50) or INMA group (n = 488) based on postoperative pathology. Radiomic features were extracted from non-contrast-enhanced CT scans and identified using the least absolute shrinkage and selection operator (LASSO) algorithm. In constructing radiomics-based models, logistic regression, support vector machines, classification and regression trees, and k-nearest neighbors were employed. Additionally, a clinical model was developed, focusing on CT radiological features. Subsequently, this clinical model was integrated with the most effective radiomic model to create a combined model. Performance assessments of these models were conducted, utilizing metrics such as the area under the receiver operating characteristic curve (AUC), DeLong’s test, net reclassification index (NRI), and integrated discrimination improvement (IDI).
Results
The support vector machine approach showed superior predictive efficiency, with AUCs of 0.829 and 0.846 in the training and test cohorts, respectively. The clinical model had AUCs of 0.760 and 0.777 in the corresponding cohorts. The combined model had AUCs of 0.847 and 0.857 in the corresponding cohorts. Furthermore, compared to the radiomic model, the combined model significantly improved performance in both the training (DeLong test P = 0.045, NRI 0.206, IDI 0.024) and test cohorts (P = 0.029, NRI 0.125, IDI 0.032), as well as compared to the clinical model in both the training (P = 0.01, NRI 0.310, IDI 0.09) and test cohorts (P = 0.047, NRI 0.382, IDI 0.085).
Conclusion
the combined model exhibited excellent performance in distinguishing between IMA and INMA in SPNs ≤3 cm.
更多查看译文
关键词
invasive mucinous adenocarcinoma,solitary pulmonary nodules,radiomics,machine learning
AI 理解论文
溯源树
样例
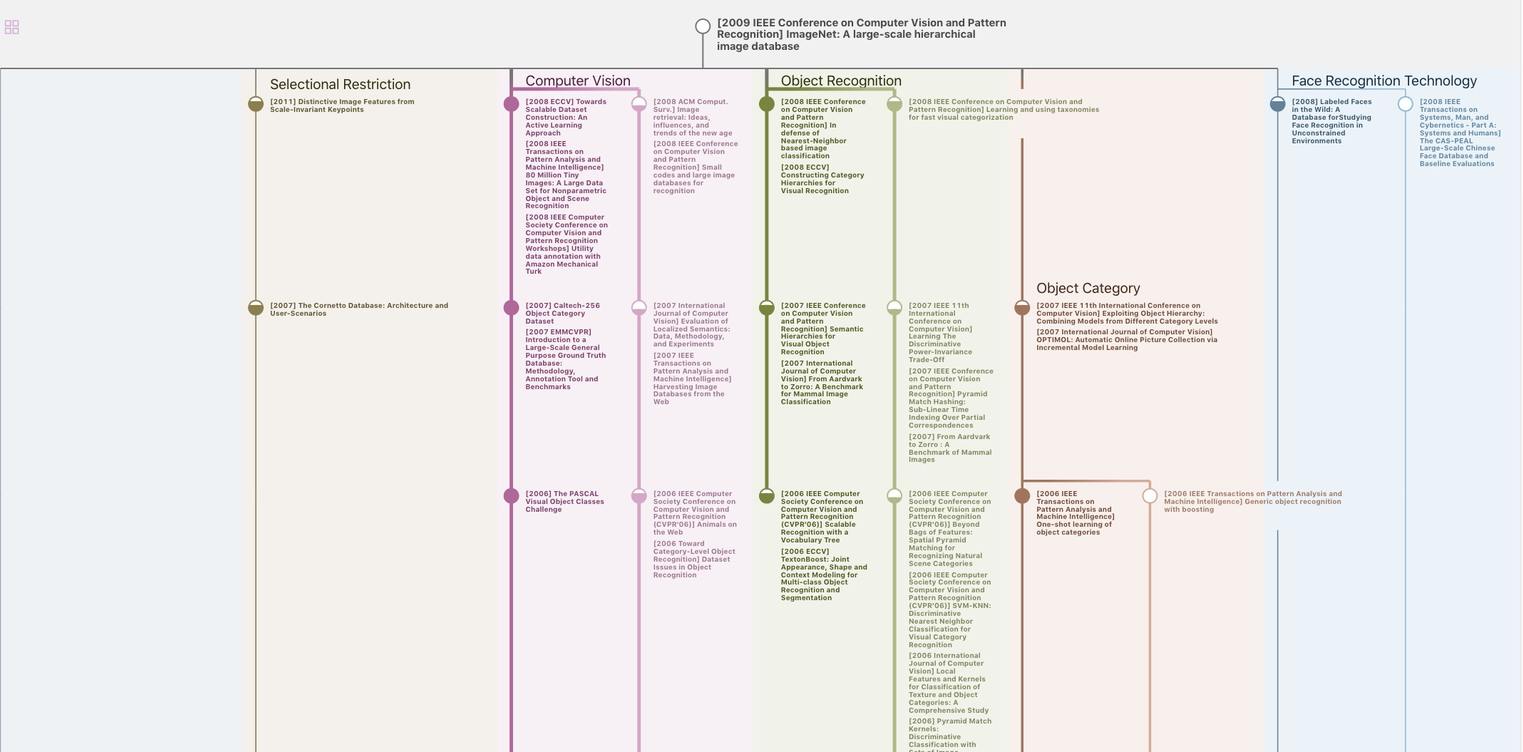
生成溯源树,研究论文发展脉络
Chat Paper
正在生成论文摘要