Dynamic Passenger Route Guidance in the Multimodal Transit System With Graph Representation and Attention Based Deep Reinforcement Learning
IEEE TRANSACTIONS ON INTELLIGENT TRANSPORTATION SYSTEMS(2024)
摘要
Recently, the limited capacity of the Urban Rail Transit (URT) has failed to meet passenger travel demands, especially in peak hours, which leads to crowded stations and oversaturated metro networks. Considering the diverse scenarios of the multimodal public transportation network and the need for rapid generation of strategies, this study proposes a deep reinforcement learning (RL) algorithm that guides passenger route selection in the multimodal transit network to operate better. The proposed RL algorithm, consisting of graph representation learning, convolution neural network, and self-attention mechanism, is used to generate the route guidance strategy for passengers, aiming to alleviate the congestion of the multimodal transit network, improve passengers' travel experience, and reduce CO2 emissions. Based on the multimodal transit network in Beijing, the simulation results demonstrate that the RL algorithm can well perceive the states and generate adaptive route guidance strategies that can decrease the section load rates, improve network-wide passengers' travel experience, and reduce CO2 emissions. Even in cases where passengers are not fully compliant with the route guidance, the proposed algorithm remains effective.
更多查看译文
关键词
Heuristic algorithms,Vehicle dynamics,Prediction algorithms,Urban areas,Convolutional neural networks,Training,Schedules,Deep reinforcement learning,passenger route guidance,graph representation,multimodal transit system,adaptive operation strategies
AI 理解论文
溯源树
样例
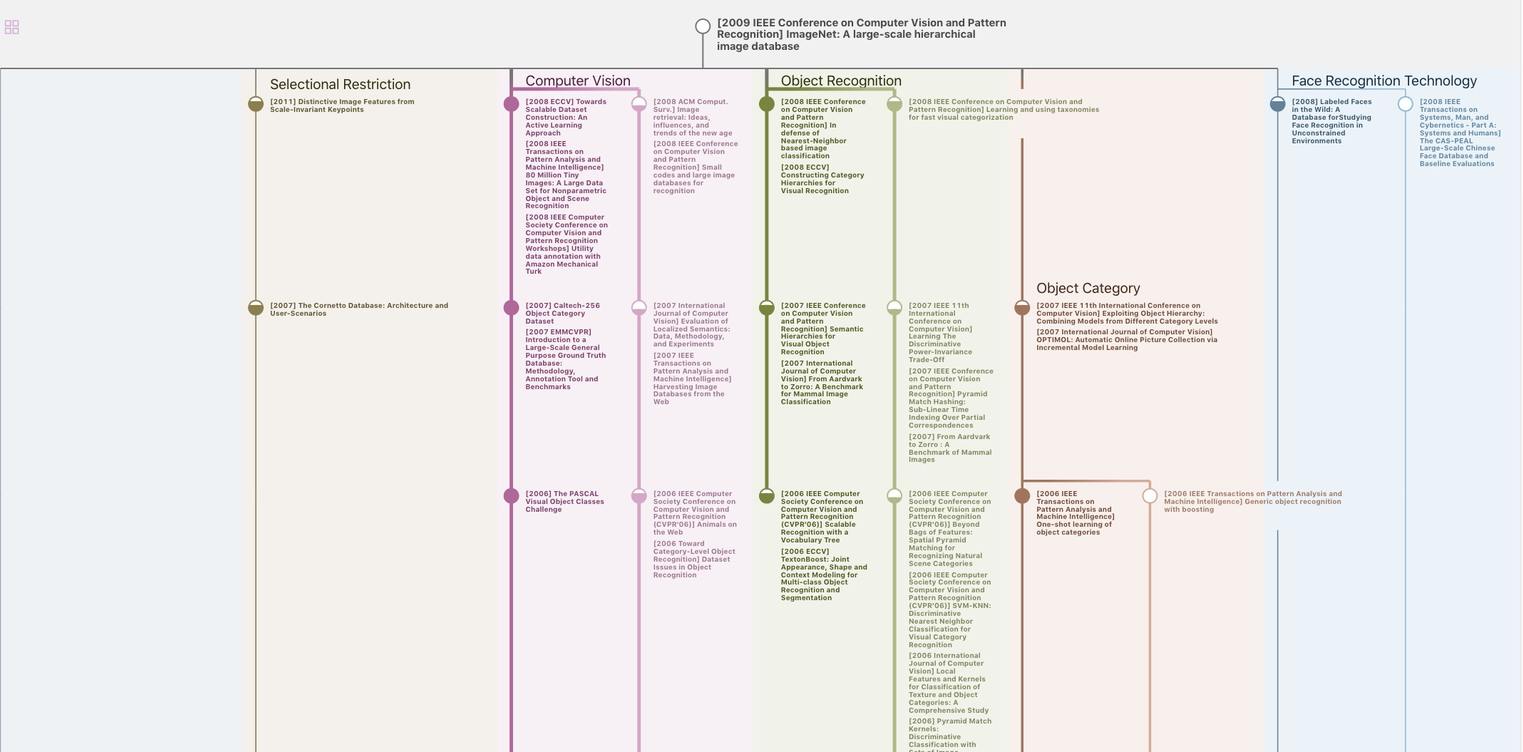
生成溯源树,研究论文发展脉络
Chat Paper
正在生成论文摘要