Transfer Kernel Sparse Coding Based on Dynamic Distribution Alignment for Image Representation
2024 4th International Conference on Consumer Electronics and Computer Engineering (ICCECE)(2024)
摘要
Sparse coding based domain adaptation methods aim to learn a robust transfer classifier by utilizing the knowledge from source domain and the learned new representation of both domains. Most existing works have achieved remarkable results in solving linear domain shift problems, but have poor performance in nonlinear domain shift problems. In this paper, we propose a Transfer kernel sparse coding based on dynamic distribution alignment (TKSC-DDA) approach for cross-domain visual recognition, which incorporates dynamic distributed alignment into kernel sparse coding to learn discriminative and robust sparse representations. Extensive experiment on visual transfer learning tasks demonstrate that our proposed method can significantly out-perform serval state-of-the-art approaches.
更多查看译文
关键词
sparse coding,transfer learning,domain adaptation,distribution alignment
AI 理解论文
溯源树
样例
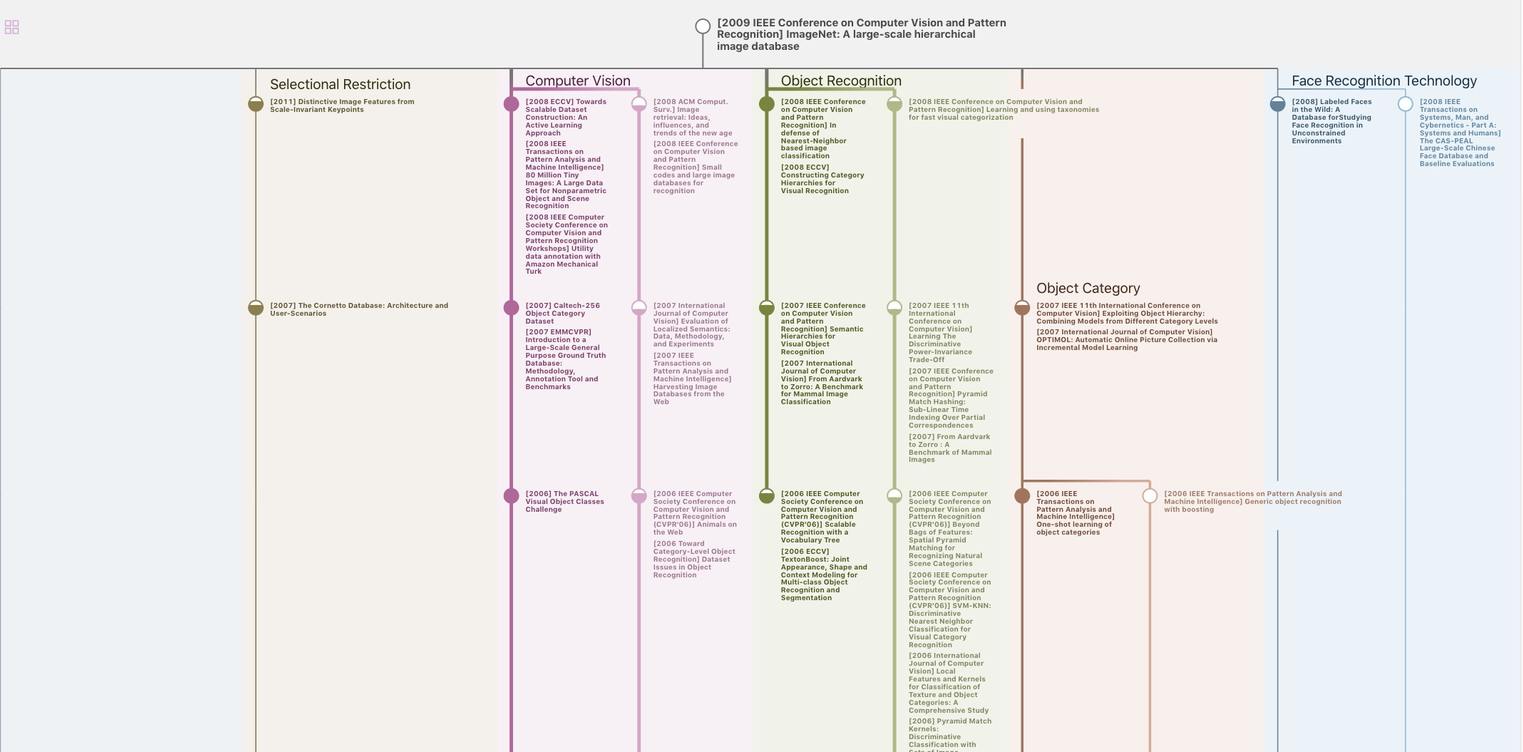
生成溯源树,研究论文发展脉络
Chat Paper
正在生成论文摘要