Compressive Hyperspectral Target Detection with Restricted Distribution Property
IEEE Transactions on Geoscience and Remote Sensing(2024)
摘要
Compressive sensing is a widely utilized technique in hyperspectral imaging front-end, and hyperspectral target detection (HTD) in the compressive sensing domain is crucial for conserving computational and storage resources on front-end platforms and achieving real-time detection. However, existing HTD methods can only handle reconstructed hyperspectral images. To address this limitation, this study investigates a novel property under hyperspectral compressive sensing and proposes an algorithm framework for HTD without reconstruction. The newly proposed property, named Restricted Distribution Property (RDP), is based on assumptions about spectral random vectors and derivations from compressive sensing model. It indicates that the spectral vectors in the compressed hyperspectral follow a deterministic Gaussian distribution under specific conditions, and provides a generalized expression for their covariance matrix. Building upon this property, a KL divergence subspace distribution estimator is developed, enabling HTD without reconstruction. Experimental result demonstrates that the performance of proposed detector in compressed hyperspectral data is comparable to or even superior to conventional methods in original hyperspectral images. The result is inspiring for future research.
更多查看译文
关键词
Hyperspectral,compressive sensing,target detect,restricted distribution property(RDP)
AI 理解论文
溯源树
样例
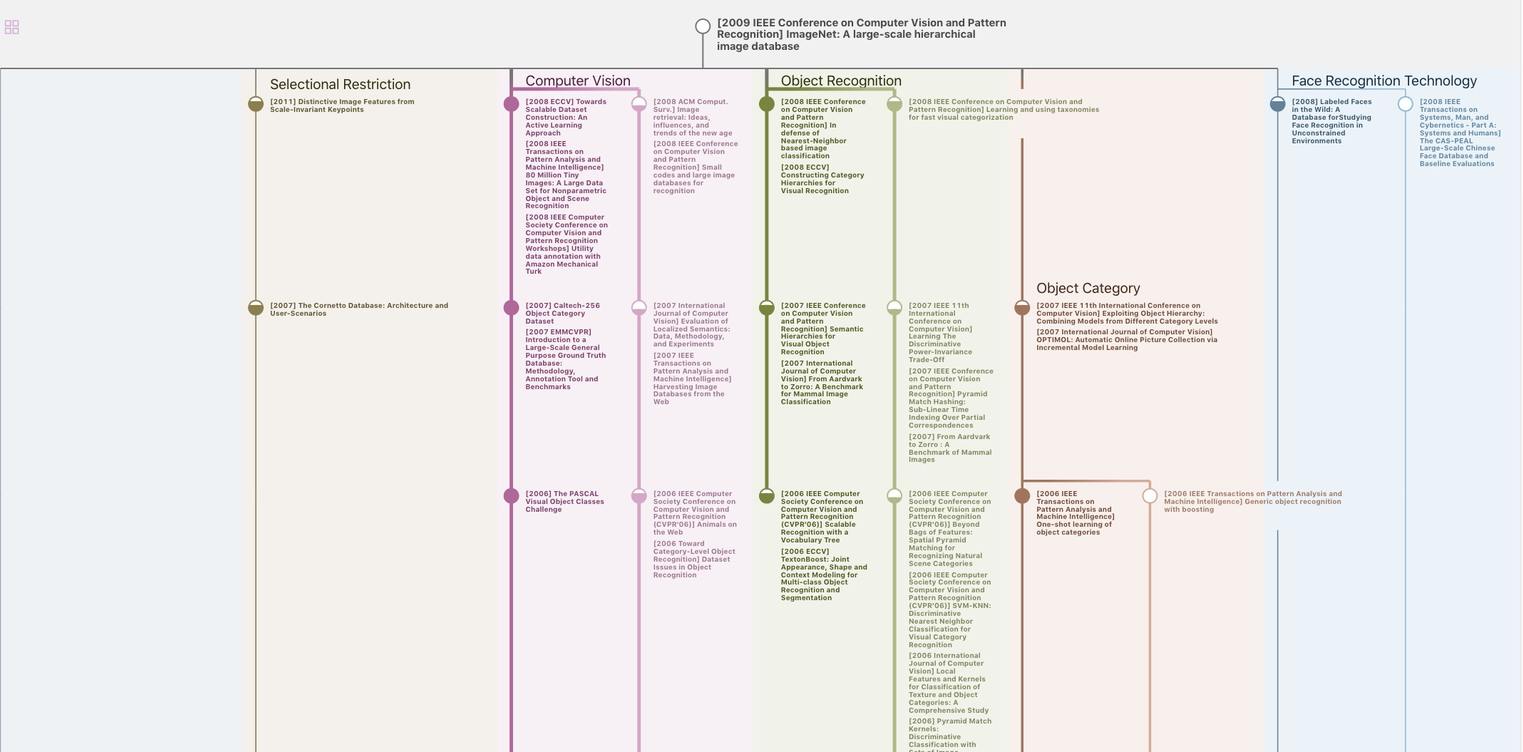
生成溯源树,研究论文发展脉络
Chat Paper
正在生成论文摘要