Fruitful Fusion: An Accuracy-Boosting Ensemble of VGG19 and Convolutional Neural Networks for Dragon Fruit Classification
2024 IEEE International Conference on Interdisciplinary Approaches in Technology and Management for Social Innovation (IATMSI)(2024)
摘要
Due to its complex features and variations, dragon fruit is an exotic and aesthetically pleasing fruit that poses a special challenge for precise and effective classification. To tackle this problem, we suggest a novel strategy that makes use of the combined strength of an ensemble that consists of the CNN and VGG19 models for the classification of dragon fruit. By utilising the unique advantages of each model, this group develops a cohesive system that has the potential to revolutionise the accuracy and consistency of fruit classification. Renowned for its hierarchical feature learning skills, the VGG19 model is excellent at identifying the finer details and patterns found in images of dragon fruit. Because of its deep architecture, which lays the groundwork for nuanced understanding, the model is able to identify minute differences in texture, colour, and shape that are essential for precise classification. By combining these models, the ensemble adds diversity to learning and guarantees a thorough approach to the classification task. Even in situations where labelled datasets may be scarce, the ensemble uses transfer learning to quickly adjust to the distinctive qualities of dragon fruit by utilising pre-trained weights. Strong decision-making is produced by the interaction of the VGG19 and CNN models, which lowers the possibility of incorrect classifications and increases the classification system’s overall accuracy. The proposed work has been implemented by ensembling the VGG19 model with the CNN model for dragon fruit classification. This method has potential for practical use, especially in sorting facilities where quick and precise classification is necessary for quality assurance. The ensemble’s flexibility makes it scalable to changing challenges in the classification of dragon fruit, which makes it an adaptable solution for dynamic agricultural environments. The proposed model has been implemented at different scales of learning rate such as 0.05, 0.15, 0.015, and 0.0015. The results depict that the proposed model outperforms by showing the highest testing accuracy at a learning rate value of 0.015 as 95.01%, whereas at a learning rate of 0.05, the testing accuracy of 83.675% has been identified.
更多查看译文
关键词
Dragon fruit classification,VGG19,image preprocessing,image augmentation,feature extraction
AI 理解论文
溯源树
样例
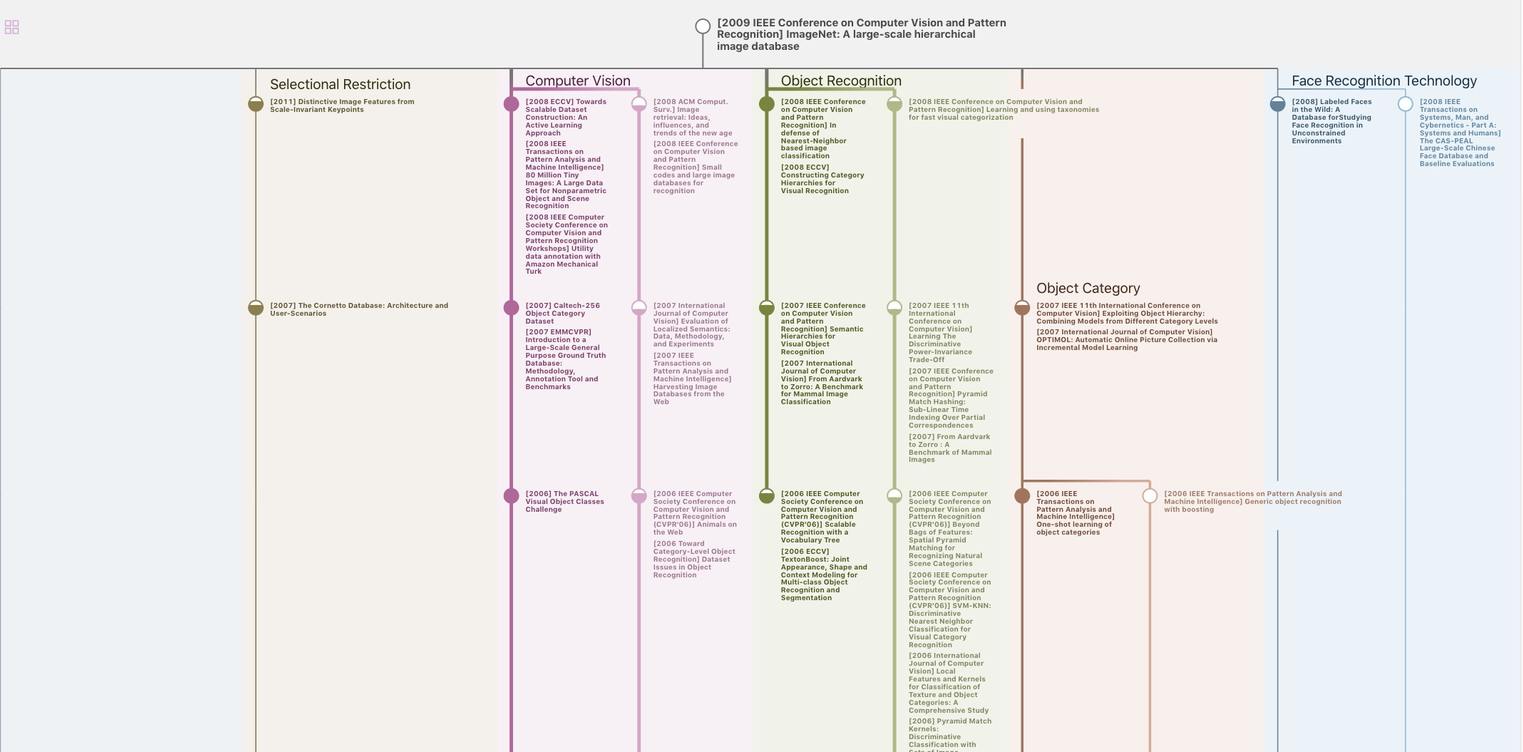
生成溯源树,研究论文发展脉络
Chat Paper
正在生成论文摘要