Performance of machine learning for ozone modeling in Southern California during the COVID-19 shutdown
ENVIRONMENTAL SCIENCE-ATMOSPHERES(2024)
摘要
We combine machine learning (ML) and geospatial interpolations to create two-dimensional high-resolution ozone concentration fields over the South Coast Air Basin (SoCAB) for the entire year of 2020. The interpolated ozone concentration fields were constructed using 15 building sites whose daily trends were predicted by random forest regression. Spatially interpolated ozone concentrations were evaluated at 12 sites that were independent from the machine learning sites and historical data to find the most suitable prediction method for SoCAB. Ordinary kriging interpolation had the best performance overall for 2020. The model is best at interpolating ozone concentrations inside the sampling region (bounded by the building sites), with R2 ranging from 0.56 to 0.85 for those sites. All interpolation methods poorly predicted and underestimated ozone concentrations for Crestline during summer, indicating that the site has a distribution of ozone concentrations that is independent from all other sites. Therefore, historical data from coastal and inland sites should not be used to predict ozone in Crestline using data-driven spatial interpolation approaches. The study demonstrates the utility of ML and geospatial techniques for evaluating air pollution levels during anomalous periods. Both ML and the Community Multiscale Air Quality model do not fully capture the irregularities caused by emission reductions during the COVID-19 lockdown period (March-May) in the SoCAB. Including 2020 training data in the ML model training improves the model's performance and its potential to predict future abnormalities in air quality. We combine machine learning (ML) and geospatial interpolations to create two-dimensional high-resolution ozone concentration fields over the South Coast Air Basin for the entire year of 2020.
更多查看译文
AI 理解论文
溯源树
样例
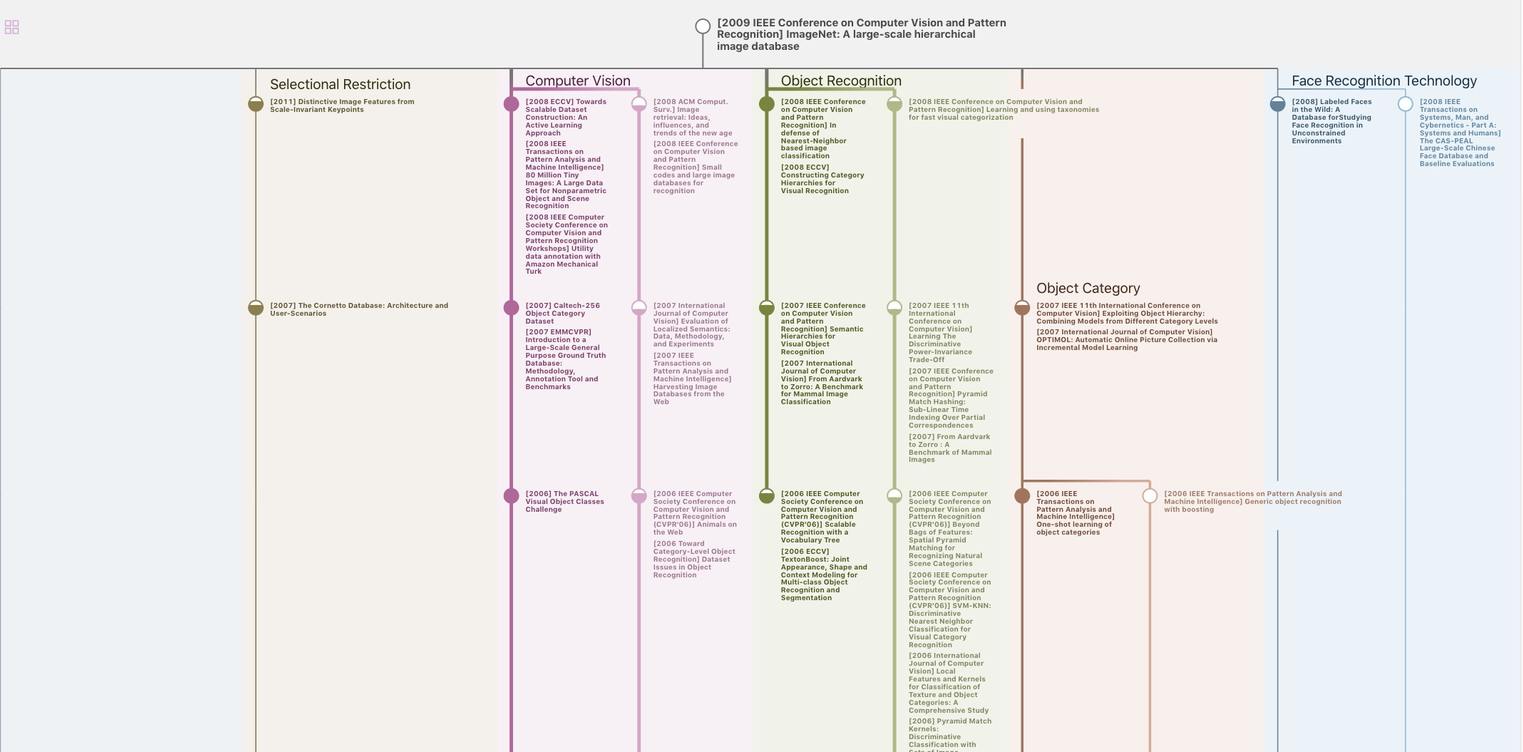
生成溯源树,研究论文发展脉络
Chat Paper
正在生成论文摘要