Improved Resolution and Image Quality of Musculoskeletal Magnetic Resonance Imaging using Deep Learning-based Denoising Reconstruction: A Prospective Clinical Study
Skeletal Radiology(2024)
摘要
To prospectively evaluate a deep learning-based denoising reconstruction (DLR) for improved resolution and image quality in musculoskeletal (MSK) magnetic resonance imaging (MRI). Images from 137 contrast-weighted sequences in 40 MSK patients were evaluated. Each sequence was performed twice, first with the routine parameters and reconstructed with a routine reconstruction filter (REF), then with higher resolution and reconstructed with DLR, and with three conventional reconstruction filters (NL2, GA43, GA53). The five reconstructions (REF, DLR, NL2, GA43, and GA53) were de-identified, randomized, and blindly reviewed by three MSK radiologists using eight scoring criteria and a forced ranking. Quantitative SNR, CNR, and structure’s full width at half maximum (FWHM) for resolution assessment were measured and compared. To account for repeated measures, Generalized Estimating Equations (GEE) with Bonferroni adjustment was used to compare the reader’s scores, SNR, CNR, and FWHM between DLR vs. NL2, GA43, GA53, and REF. Compared to the routine REF images, the resolution was improved by 47.61
更多查看译文
关键词
Human observer study,Image quality assessment,MRI deep learning reconstruction,Prospective clinical evaluation
AI 理解论文
溯源树
样例
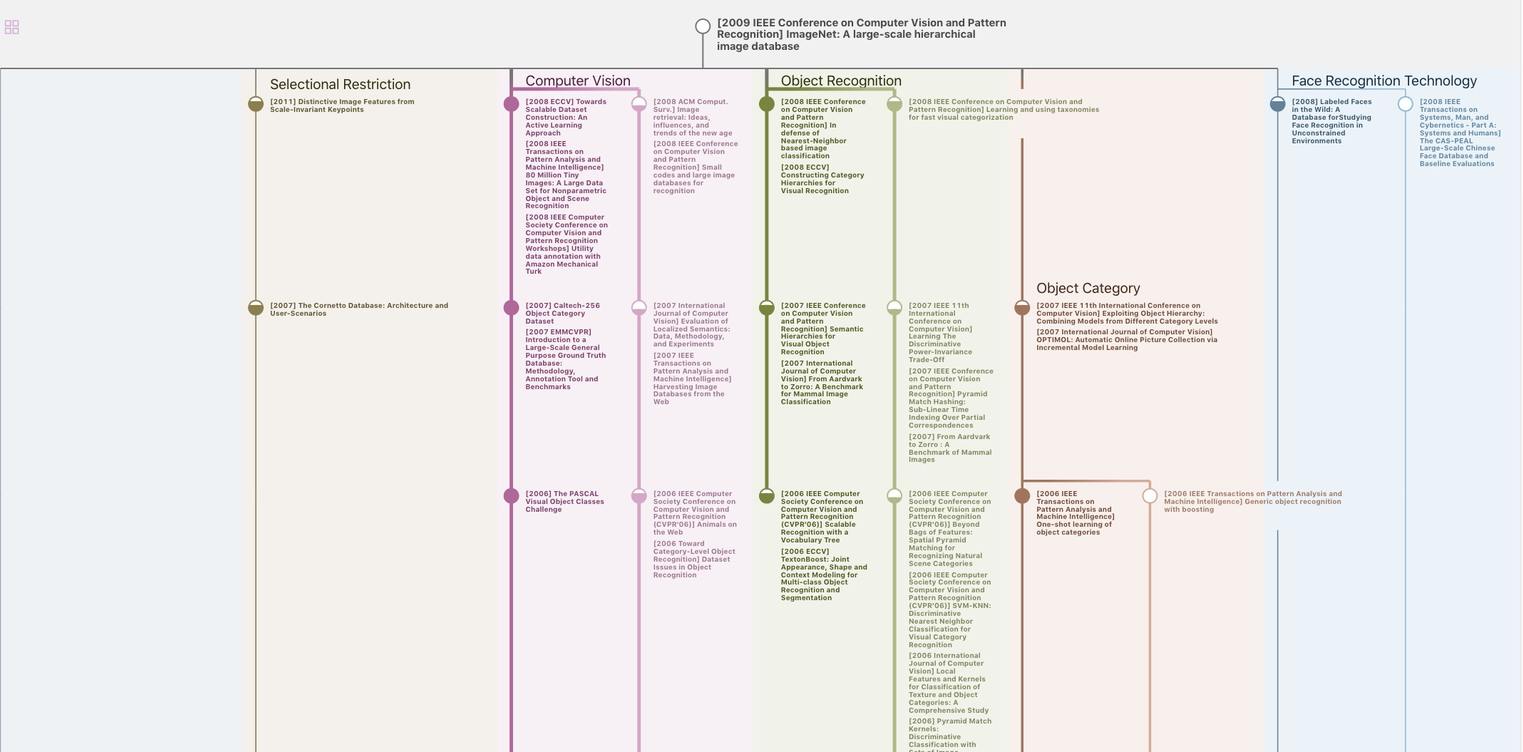
生成溯源树,研究论文发展脉络
Chat Paper
正在生成论文摘要