Guarantee structural anomaly detection in streaming data using the RRCF model: selection of detector parameters and its stabilization under additive noise conditions
Naučno-tehničeskij Vestnik Informacionnyh Tehnologij, Mehaniki i Optiki(2024)
摘要
A method for stabilizing structural anomaly detection under additive noise conditions as well as an algorithm for formal selection of the parameters of the solver rule in the structural anomaly detector based on the Robust Random Cut Forest (RRCF) method are proposed. In the framework of the developed approach, in order to stabilize the process of structural anomaly detection under the influence of additive noise, it is proposed to feed to the input of the RRCF-detector a data stream which is pre-processed by one of the digital filtering methods. In this case, the decision rule for anomaly detection is strictly formalized and transparently interpreted. The selection of parameters of the RRCF-based anomaly detector stabilized by pre-filtering methods of the input data stream is formalized. The RRCF-detector parameters choice within the proposed scheme guarantees a predetermined upper bound for the false alarm probability when deciding to detect a structural anomaly. This property is rigorously proved and formalized as a theorem. The performance of the stabilized RRCF-detector is investigated numerically. The achieved results confirm the performance of the proposed approach provided that the detection threshold is selected in the way proposed in this paper. An example of practical application of the proposed method is presented. The developed approach is promising for the detection of structural anomalies in conditions of observation additive noise, in a situation where it is important to guarantee an upper bound for the probability of false alarm. In particular, the approach can find application in monitoring technological regimes of liquid pumping in pipeline systems or in systems for detecting pre-failure states of technological equipment.
更多查看译文
关键词
robust random cut forest,structural anomaly detection,streaming data processing,guaranteed anomaly detection
AI 理解论文
溯源树
样例
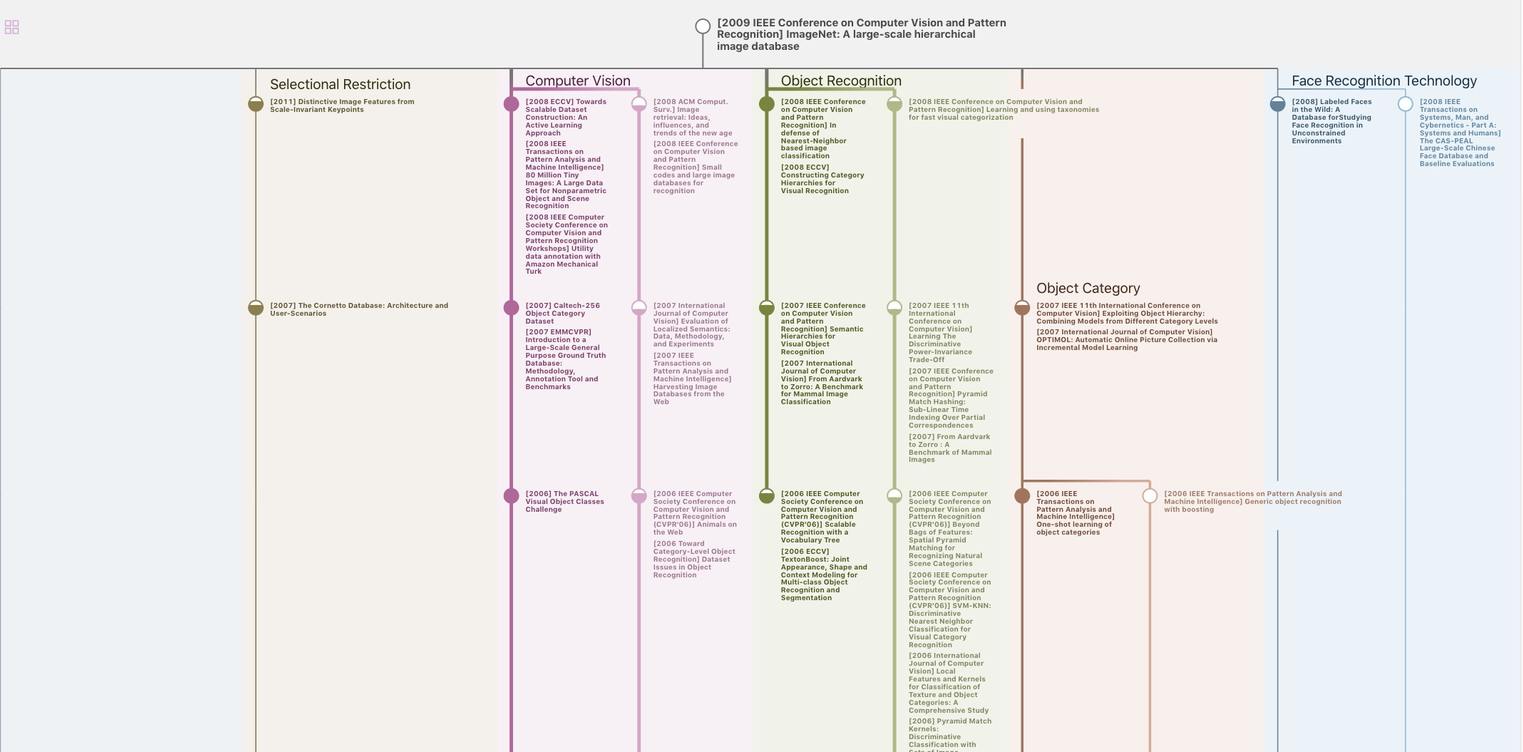
生成溯源树,研究论文发展脉络
Chat Paper
正在生成论文摘要