Micro Activity Recognition Using Multi-View 3D Point Clouds.
Annual IEEE International Conference on Pervasive Computing and Communications(2024)
摘要
In recent years, aiming to support residents’ daily lives and activities, research and development activities on indoor activity recognition have become increasingly active. However, existing methods of activity recognition still have challenges including (i) concerns about privacy infringement, (ii) the necessity of wearing sensors constantly, (iii) the need for regular battery charging, and (iv) the difficulty in recognizing certain activities. Additionally, the methods primarily focused on macro activities like cooking and cleaning. Still, it is believed that a more accurate application to life support requires recognition of more detailed activities known as micro activities, such as handling ingredients in cooking or cutting. In this paper, we propose a method for micro activity recognition using 3D point clouds as a solution to these issues. 3D point clouds represent objects as sets of 3D coordinates and are expected to offer lower privacy risks and higher accuracy compared to video images. The challenges to be addressed in the proposed method include defining the micro activities of interest and recognizing them from 3D point clouds. In the proposed method, micro activities are defined as single actions like “cutting,” “holding,” or “placing” that occur in cooking activities in typical households. We then apply a deep learning model called P4Transformer, which can classify time-series 3D point cloud input data obtained from multiple Azure Kinect devices installed in typical households, allowing for occlusion-tolerant micro activity recognition. We have collected a time-series 3D point cloud data for more than 40 sessions of cooking activities in five typical households, then evaluated the accuracy of the micro activity recognition model using Leave-One-Session-Out cross-validation. The results showed that the proposed method achieves an average recognition accuracy of 74.3%.
更多查看译文
AI 理解论文
溯源树
样例
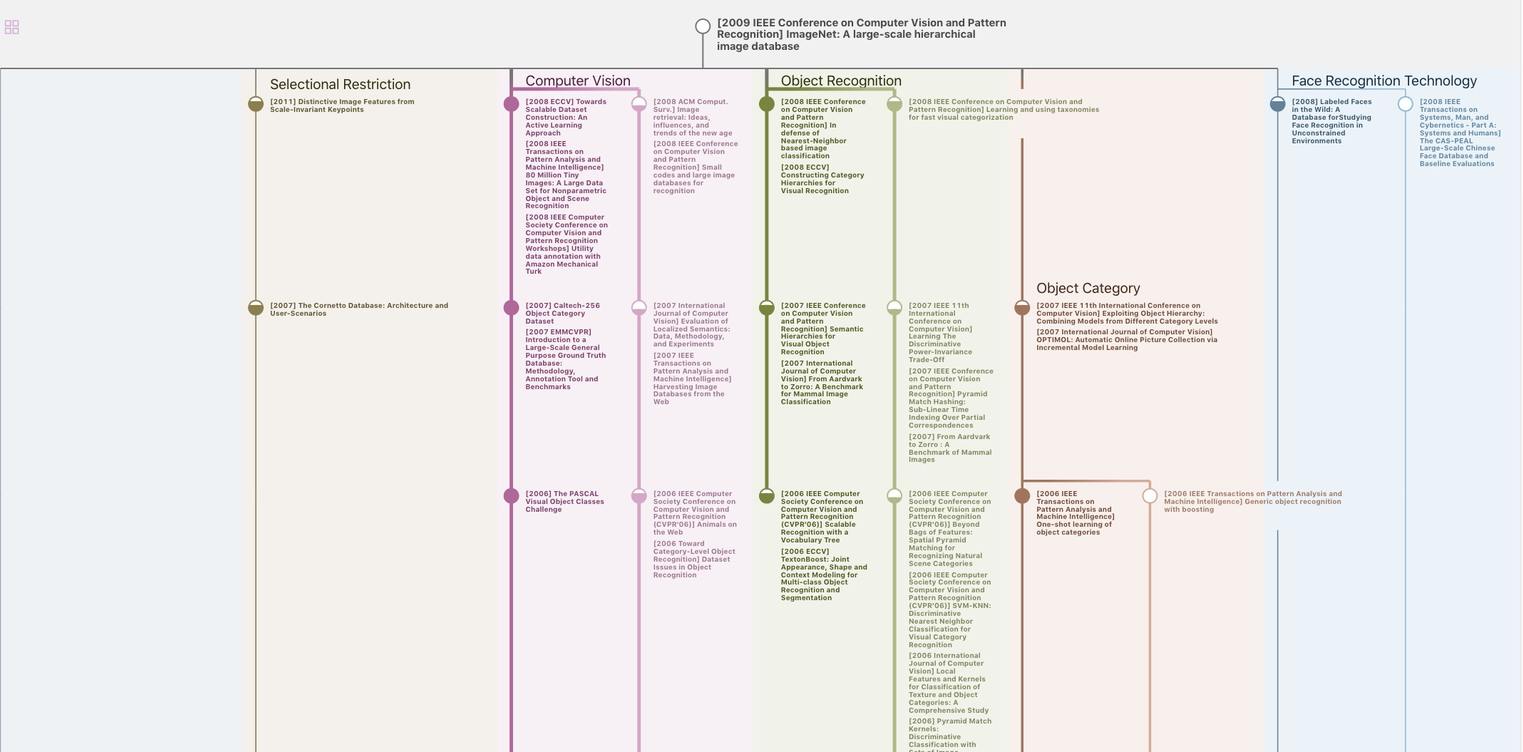
生成溯源树,研究论文发展脉络
Chat Paper
正在生成论文摘要