Label-Efficient Sleep Staging Using Transformers Pretrained with Position Prediction
2024 IEEE First International Conference on Artificial Intelligence for Medicine, Health and Care (AIMHC)(2024)
摘要
Sleep staging is a clinically important task for diagnosing various sleep disorders, but remains challenging to deploy at scale because it because it is both labor-intensive and time-consuming. Supervised deep learning-based approaches can automate sleep staging but at the expense of large labeled datasets, which can be unfeasible to procure for various settings, e.g., uncommon sleep disorders. While self-supervised learning (SSL) can mitigate this need, recent studies on SSL for sleep staging have shown performance gains saturate after training with labeled data from only tens of subjects, hence are unable to match peak performance attained with larger datasets. We hypothesize that the rapid saturation stems from applying a sub-optimal pretraining scheme that pretrains only a portion of the architecture, i.e, the feature encoder, but not the temporal encoder; therefore, we propose adopting an architecture that seamlessly couples the feature and temporal encoding and a suitable pretraining scheme that pretrains the entire model. On a sample sleep staging dataset, we find that the proposed scheme offers performance gains that do not saturate with amount of labeled training data (e.g., 3–5 % improvement in balanced sleep staging accuracy across low- to high-labeled data settings), reducing the amount of labeled training data needed for high performance (e.g., by 800 subjects). Based on our findings, we recommend adopting this SSL paradigm for subsequent work on SSL for sleep staging.
更多查看译文
AI 理解论文
溯源树
样例
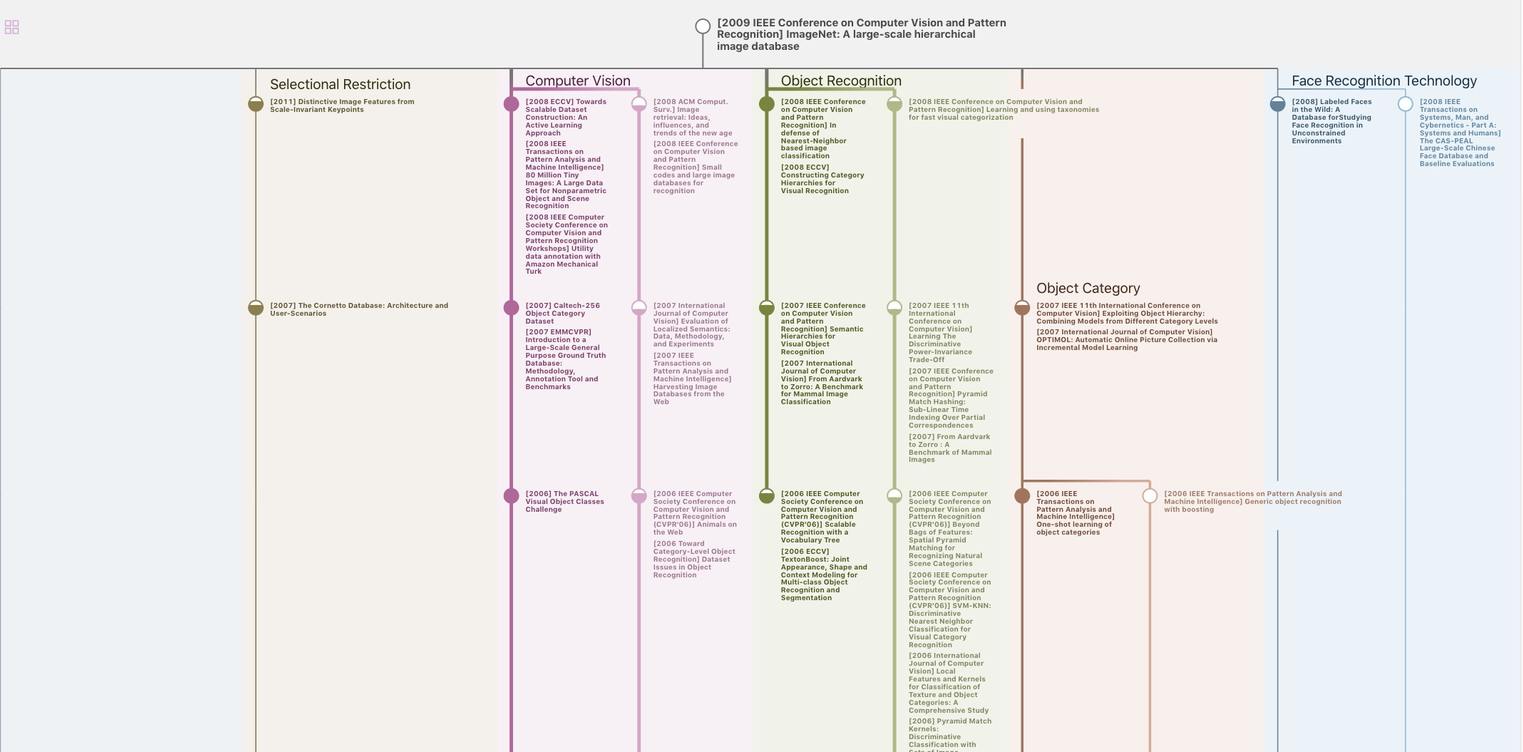
生成溯源树,研究论文发展脉络
Chat Paper
正在生成论文摘要