Dual-Channel Adaptive Scale Hypergraph Encoders With Cross-View Contrastive Learning for Knowledge Tracing
IEEE TRANSACTIONS ON NEURAL NETWORKS AND LEARNING SYSTEMS(2024)
摘要
Knowledge tracing (KT) refers to predicting learners' performance in the future according to their historical responses, which has become an essential task in intelligent tutoring systems. Most deep learning-based methods usually model the learners' knowledge states via recurrent neural networks (RNNs) or attention mechanisms. Recently emerging graph neural networks (GNNs) assist the KT model to capture the relationships such as question-skill and question-learner. However, non-pairwise and complex higher-order information among responses is ignored. In addition, a single-channel encoded hidden vector struggles to represent multigranularity knowledge states. To tackle the above problems, we propose a novel KT model named dual-channel adaptive scale hypergraph encoders with cross-view contrastive learning (HyperKT). Specifically, we design an adaptive scale hyperedge distillation component for generating knowledge-aware hyperedges and pattern-aware hyperedges that reflect non-pairwise higher-order features among responses. Then, we propose dual-channel hypergraph encoders to capture multigranularity knowledge states from global and local state hypergraphs. The encoders consist of a simplified hypergraph convolution network and a collaborative hypergraph convolution network. To enhance the supervisory signal in the state hypergraphs, we introduce the cross-view contrastive learning mechanism, which performs among state hypergraph views and their transformed line graph views. Extensive experiments on three real-world datasets demonstrate the superior performance of our HyperKT over the state-of-the-art (SOTA).
更多查看译文
关键词
Self-supervised learning,Adaptation models,Convolution,Computational modeling,Predictive models,Recurrent neural networks,Knowledge engineering,Contrastive learning,hyperedge distillation,hypergraph learning,knowledge tracing (KT)
AI 理解论文
溯源树
样例
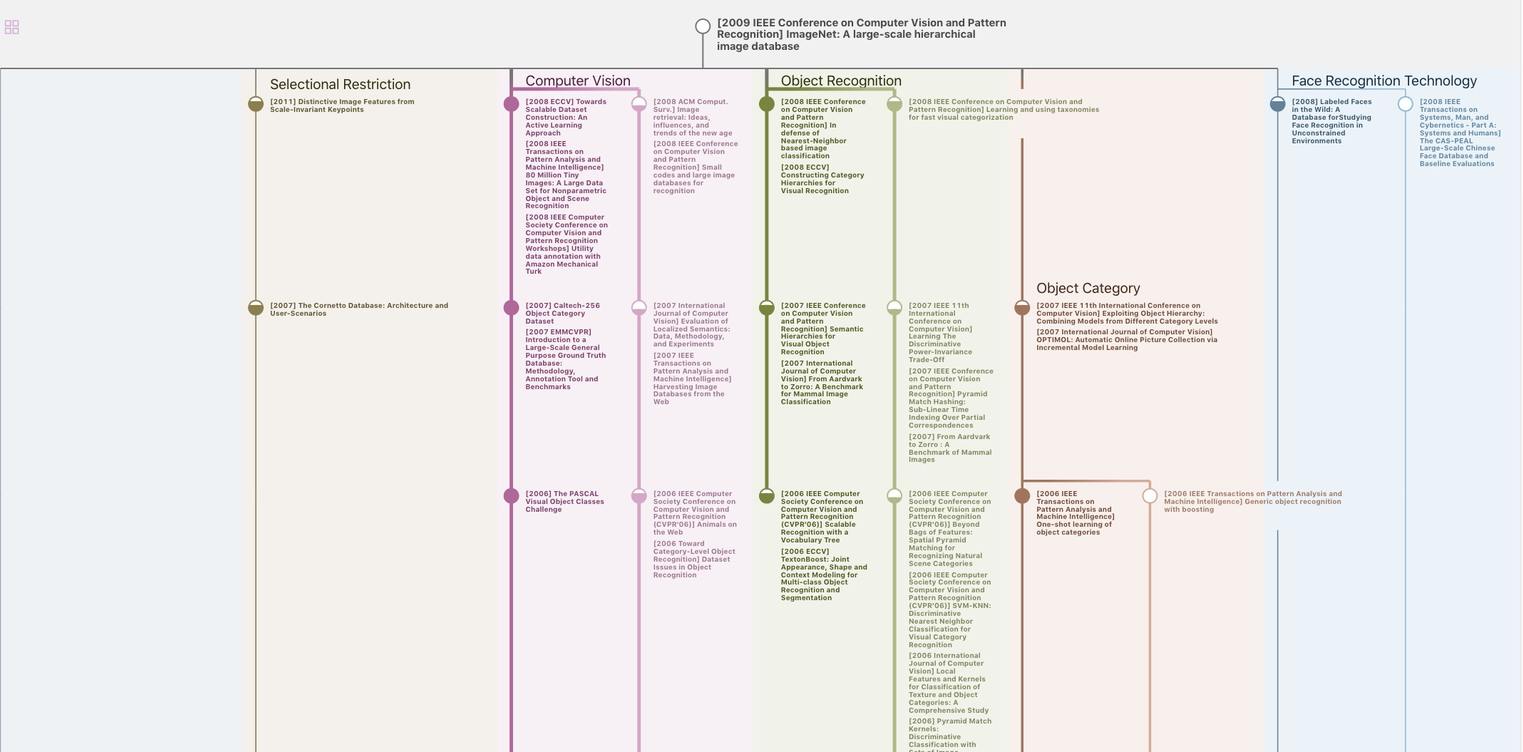
生成溯源树,研究论文发展脉络
Chat Paper
正在生成论文摘要