A Cooperative Control Methodology Considering Dynamic Interaction for Multiple Connected and Automated Vehicles in the Merging Zone
IEEE TRANSACTIONS ON INTELLIGENT TRANSPORTATION SYSTEMS(2024)
摘要
The dynamic interaction among Connected and Automated Vehicles (CAVs) is becoming increasingly complex, encompassing factors such as dynamic topology and the dynamic states of multiple CAVs. Existing cooperative control methods struggle to explicitly represent dynamic interaction, which can lead to dangerous behavior, severe congestion, and even accidents. In this paper, we propose a cooperative control methodology that aims to improve safety and efficiency in the merging zone by deeply representing dynamic interaction among multiple CAVs. Our proposed methodology, named GMA-DRL, utilizes a spatial graph convolutional encoder with a multi-head attention mechanism to explicitly represent dynamic interaction among vehicles. Furthermore, deep reinforcement learning based on Actor-Critic with temporal relation regularization is utilized to ensure the consistency of dynamic interaction and generate cooperative driving actions for multiple CAVs. The GMA-DRL is tested in a series of typical merging scenarios with dynamic interaction. Extensive experimental results show that the GMA-DRL outperforms the existing cooperative control models in term of headway, average speed and acceleration. It demonstrates that the GMA-DRL with explicitly represent dynamic interaction can improve safety and efficiency of multiple CAVs in the merging zone.
更多查看译文
关键词
Vehicle dynamics,Merging,Decision making,Deep reinforcement learning,Safety,Topology,Heuristic algorithms,Connected and automated vehicles (CAVs),cooperative control,dynamic interaction,graph convolutional network,deep reinforcement learning
AI 理解论文
溯源树
样例
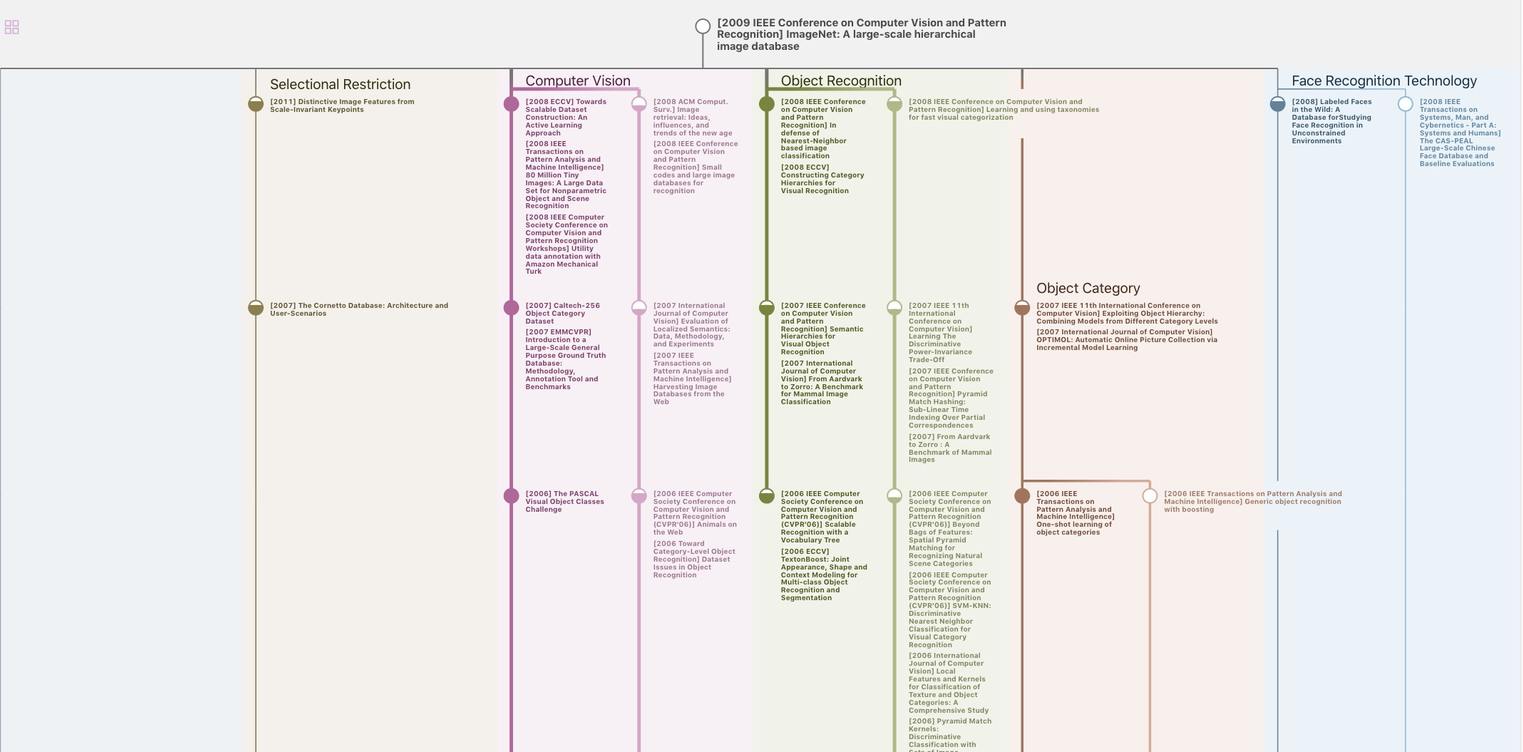
生成溯源树,研究论文发展脉络
Chat Paper
正在生成论文摘要