OntoMedRec: Logically-pretrained model-agnostic ontology encoders for medication recommendation
World Wide Web(2024)
摘要
Recommending medications with electronic health records (EHRs) is a challenging task for data-driven clinical decision support systems. Most existing models learnt representations for medical concepts based on EHRs and make recommendations with the learnt representations. However, most medications appear in EHR datasets for limited times (the frequency distribution of medications follows power law distribution), resulting in insufficient learning of their representations of the medications. Medical ontologies are the hierarchical classification systems for medical terms where similar terms will be in the same class on a certain level. In this paper, we propose OntoMedRec, the logically-pretrained and model-agnostic medical Ontology Encoders for Medication Recommendation that addresses data sparsity problem with medical ontologies. We conduct comprehensive experiments on real-world EHR datasets to evaluate the effectiveness of OntoMedRec by integrating it into various existing downstream medication recommendation models. The result shows the integration of OntoMedRec improves the performance of various models in both the entire EHR datasets and the admissions with few-shot medications. We provide the GitHub repository for the source code. ( https://github.com/WaicongTam/OntoMedRec )
更多查看译文
关键词
Medication recommendation,Logic tensor networks,Medical ontology
AI 理解论文
溯源树
样例
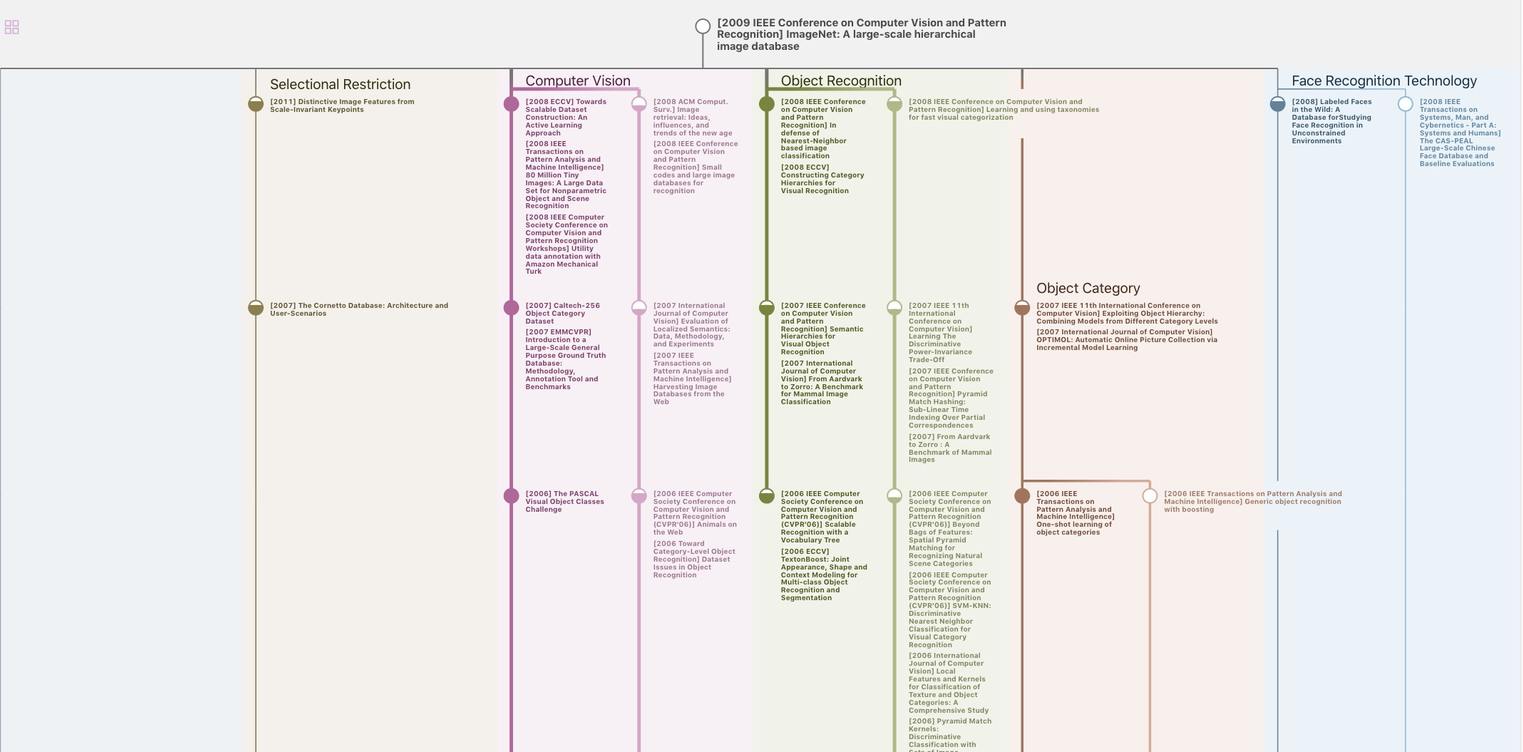
生成溯源树,研究论文发展脉络
Chat Paper
正在生成论文摘要