Graph learning with label attention and hyperbolic embedding for temporal event prediction in healthcare
Neurocomputing(2024)
摘要
The digitization of healthcare systems has led to the proliferation of electronic health records (EHRs), serving as comprehensive repositories of patient information. However, the vast volume and complexity of EHR data present challenges in extracting meaningful insights. This paper addresses the need for automated analysis of EHRs by proposing a novel graph learning model with label attention (GLLA) for temporal event prediction. GLLA utilizes graph neural networks to capture intricate relationships between medical codes and patients, incorporating hierarchical structures and shared risk factors. Furthermore, it introduces the Label Attention and Attention-based Transformer (LAAT) algorithm to analyze unstructured clinical notes as a multi-label classification problem. Evaluation on the widely-used MIMIC III dataset demonstrates the efficacy of GLLA in enhancing diagnostic prediction performance. The contributions of this research include a comprehensive analysis of existing models, the identification of limitations, and the development of innovative approaches to improve the accuracy and effectiveness of EHR analysis. Ultimately, GLLA aims to advance healthcare decision-making, disease management strategies, and patient outcomes.
更多查看译文
关键词
Temporal event prediction,Hierarchical embeddings,Graph neural networks,Clinical notes
AI 理解论文
溯源树
样例
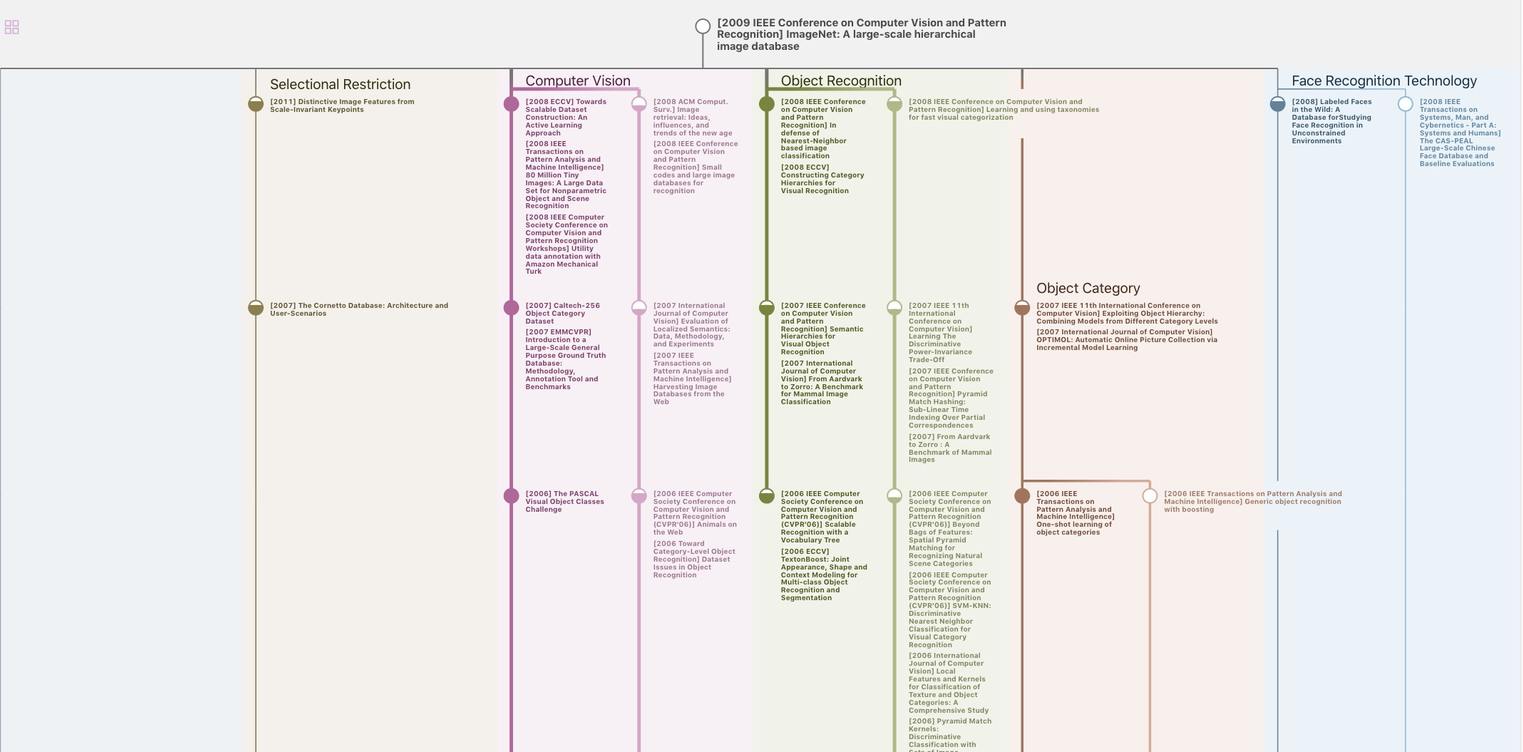
生成溯源树,研究论文发展脉络
Chat Paper
正在生成论文摘要