Rank-1 transition uncertainties in constrained Markov decision processes
European Journal of Operational Research(2024)
摘要
We consider an infinite-horizon discounted constrained Markov decision process (CMDP) with uncertain transition probabilities. We assume that the uncertainty in transition probabilities has a rank-1 matrix structure and the underlying uncertain parameters belong to a polytope. We formulate the uncertain CMDP problem using a robust optimization framework. To derive reformulation of the robust CMDP problem, we restrict to the class of stationary policies and show that it is equivalent to a bilinear programming problem. We provide a simple example where a Markov policy performs better than the optimal policy in the class of stationary policies, implying that, unlike in classical CMDP problem, an optimal policy of the robust CMDP problem need not be present in the class of stationary policies. For the case of a single uncertain parameter, we propose sufficient conditions under which an optimal policy of the restricted robust CMDP problem is unaffected by uncertainty. The numerical experiments are performed on randomly generated instances of a machine replacement problem and a well-known class of problems called Garnets.
更多查看译文
关键词
Robust optimization,Constrained Markov decision process,Bilinear programming problem,Machine replacement problem
AI 理解论文
溯源树
样例
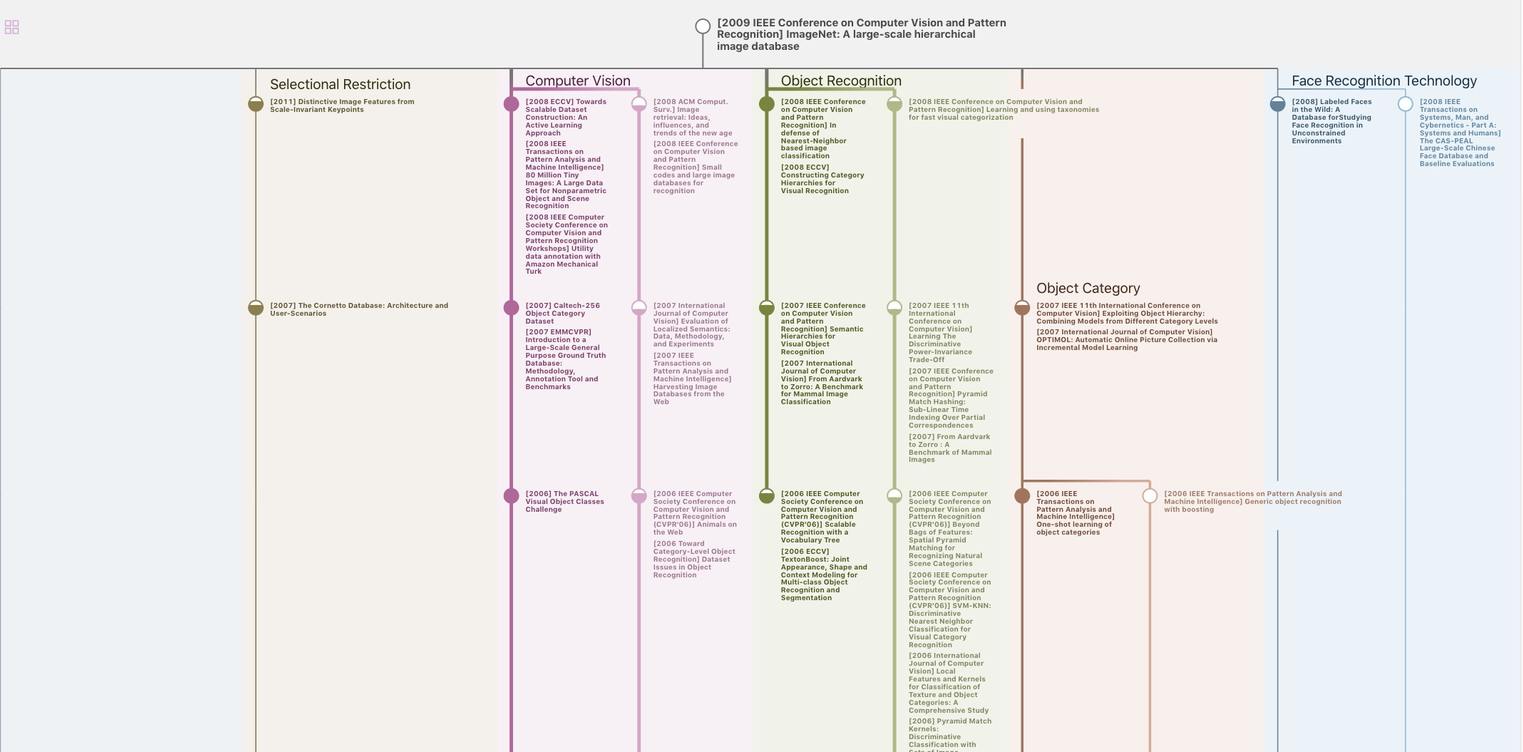
生成溯源树,研究论文发展脉络
Chat Paper
正在生成论文摘要