A Secure Neural Network Inference Framework for Intelligent Connected Vehicles
IEEE Network(2024)
摘要
Neural networks, as one of the most significant techniques in artificial intelligence, play a crucial role in various tasks associated with intelligent connected vehicles (ICVs), including but not limited to object detection, traffic flow prediction, and path planning. During the neural network inference process, the transmission and processing of sensitive data, such as images and vehicle location information, are susceptible to interception and misuse by malicious adversaries, thereby raising substantial security and privacy concerns. Existing generic secure neural network inference solutions face challenges in the context of ICVs, given constraints such as limited end-user resources and real-time requirements on inference tasks. In this article, we introduce a secure neural network inference framework comprising two distinct schemes tailored specifically for ICVs. Firstly, we propose a cloud-assisted secure inference scheme leveraging multi-key homomorphic encryption (MKHE). This scheme allows vehicles with limited computational resources to handle resource-intensive inference tasks by leveraging cloud assistance, while ensuring high-level data security. Additionally, we develop an edge-end collaborative inference scheme for real-time inference scenarios within the ICVs context. Simulation results indicate that both of our proposed inference schemes achieve excellent performance in their respective application scenarios.
更多查看译文
关键词
Intelligent connected vehicles,Secure neural network inference,MKHE,Collaborative inference
AI 理解论文
溯源树
样例
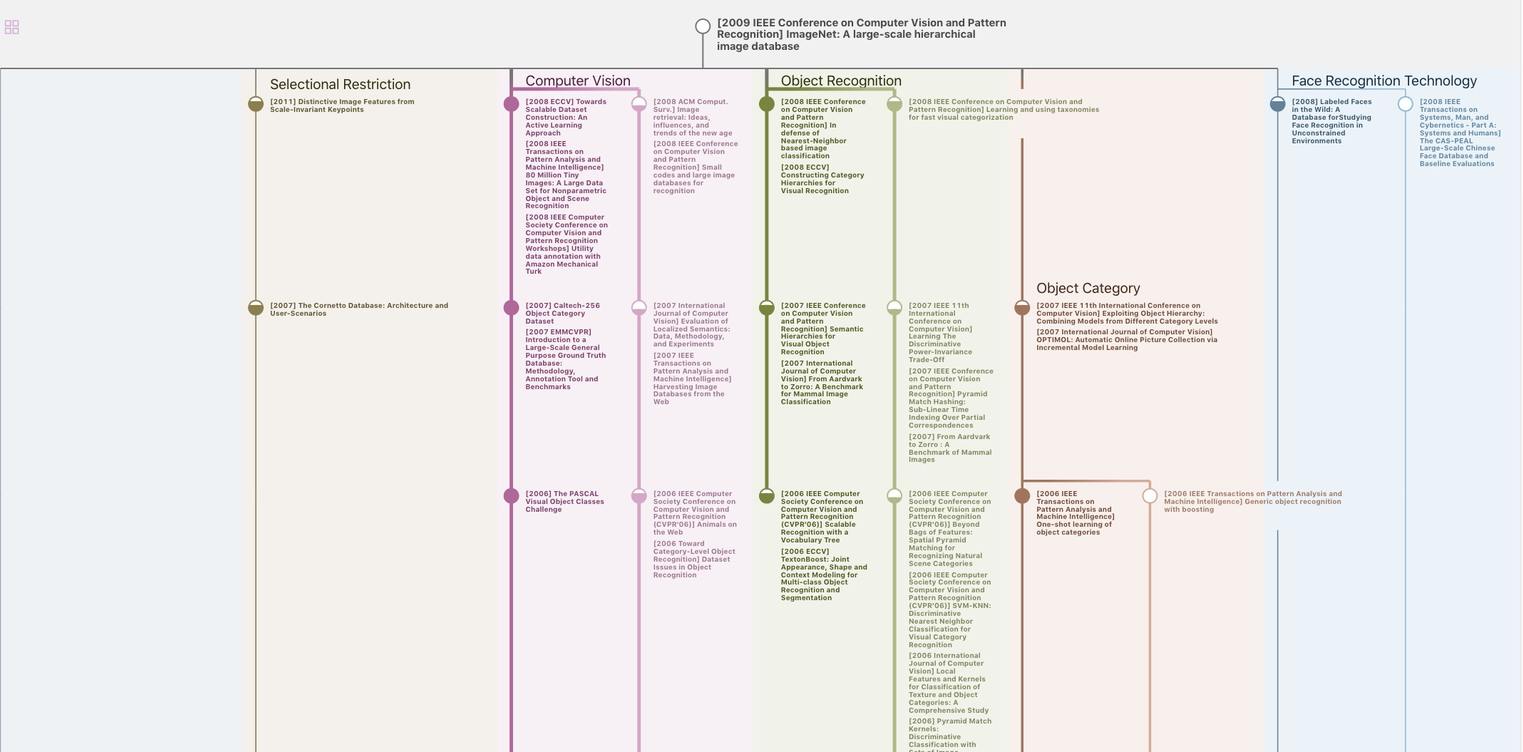
生成溯源树,研究论文发展脉络
Chat Paper
正在生成论文摘要