Neural Network Assisted Time-varying Non-sparse Underwater Acoustic Channel Estimation
2024 4th International Conference on Neural Networks, Information and Communication (NNICE)(2024)
摘要
Orthogonal frequency division multiplexing (OFDM) technique has received much attention in the field of underwater acoustics because of its high spectral utilization. However, its performance is susceptible to inter-carrier interference, so the channel estimation performance of OFDM systems under strong Doppler non-sparse channels is highly susceptible. Most of the traditional deep learning methods only consider sparse underwater acoustic channels. In this paper, we propose a neural network-assisted OFDM-based receiver to solve the communication problem under strong Doppler non-sparse channels. Distinguished from other works that used channel frequency domain parameters as neural network inputs, we use the time-domain channel impact response matrix as input. In addition, we propose a new network model for channel characterization called ALDNet, which is an integration of an autoencoder, a long short-term memory network, and a deep neural network. This network model is employed to further optimize the results after conventional channel estimation methods. The results show that the ALDNet network model is able to obtain good performance improvement under strong Doppler non-sparse channels, and the obtained BER performance gain is more than 8dB compared with the traditional method.
更多查看译文
关键词
OFDM,Channel Estimation,Neural Network,UWA-Channel
AI 理解论文
溯源树
样例
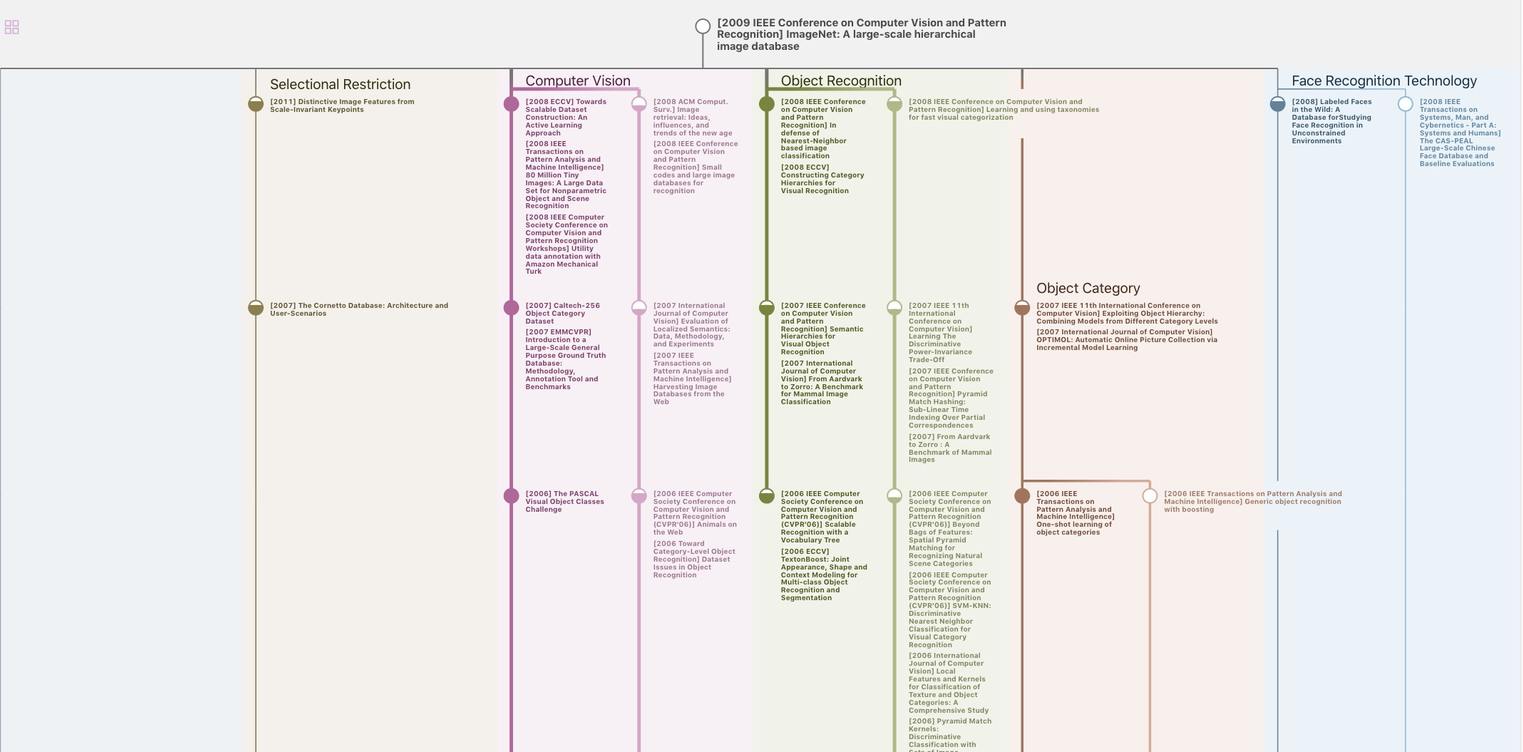
生成溯源树,研究论文发展脉络
Chat Paper
正在生成论文摘要