Body mass index-dependent shifts along large-scale gradients in human cortical organization explain dietary regulatory success.
Proceedings of the National Academy of Sciences of the United States of America(2024)
摘要
Making healthy dietary choices is essential for keeping weight within a normal range. Yet many people struggle with dietary self-control despite good intentions. What distinguishes neural processing in those who succeed or fail to implement healthy eating goals? Does this vary by weight status? To examine these questions, we utilized an analytical framework of gradients that characterize systematic spatial patterns of large-scale neural activity, which have the advantage of considering the entire suite of processes subserving self-control and potential regulatory tactics at the whole-brain level. Using an established laboratory food task capturing brain responses in natural and regulatory conditions (N = 123), we demonstrate that regulatory changes of dietary brain states in the gradient space predict individual differences in dietary success. Better regulators required smaller shifts in brain states to achieve larger goal-consistent changes in dietary behaviors, pointing toward efficient network organization. This pattern was most pronounced in individuals with lower weight status (low-BMI, body mass index) but absent in high-BMI individuals. Consistent with prior work, regulatory goals increased activity in frontoparietal brain circuits. However, this shift in brain states alone did not predict variance in dietary success. Instead, regulatory success emerged from combined changes along multiple gradients, showcasing the interplay of different large-scale brain networks subserving dietary control and possible regulatory strategies. Our results provide insights into how the brain might solve the problem of dietary control: Dietary success may be easier for people who adopt modes of large-scale brain activation that do not require significant reconfigurations across contexts and goals.
更多查看译文
AI 理解论文
溯源树
样例
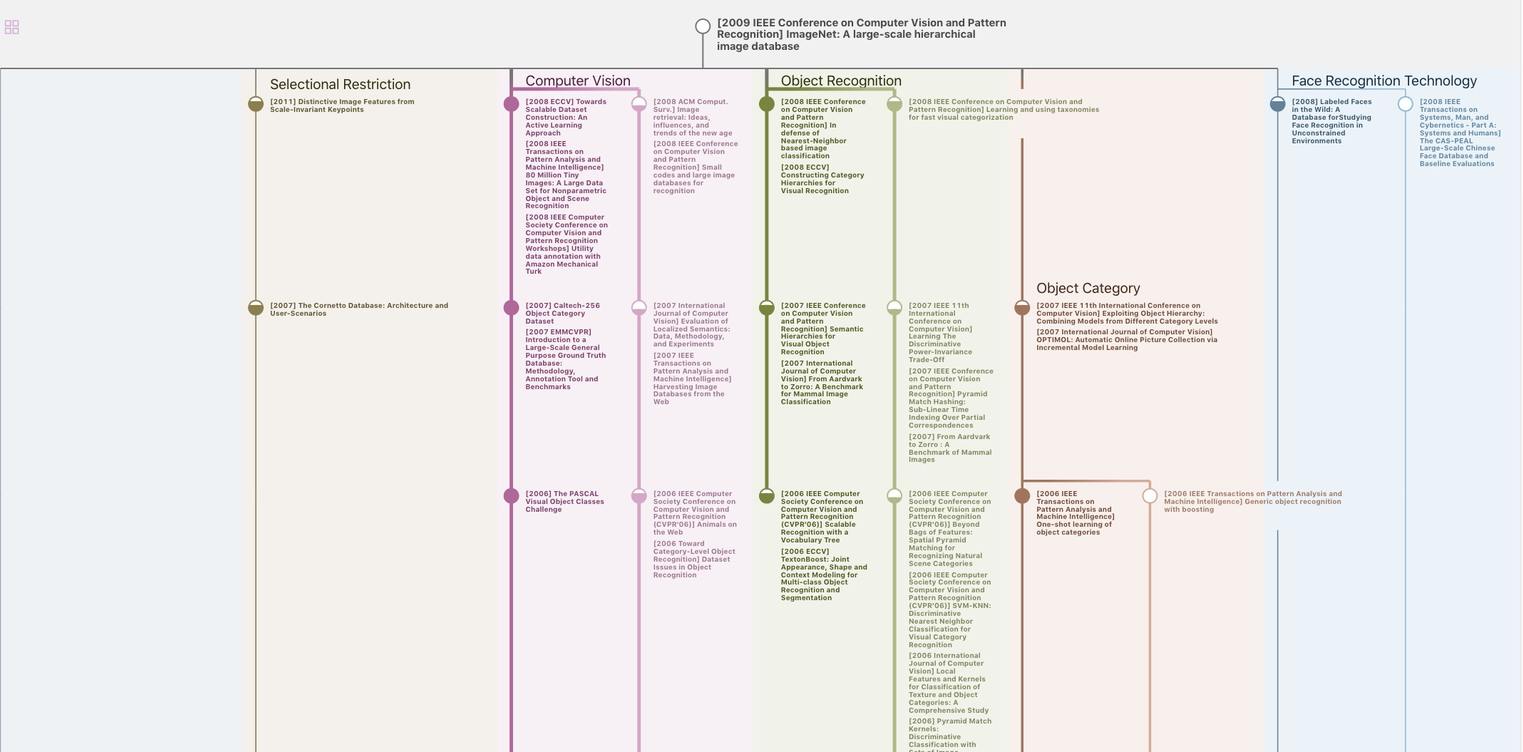
生成溯源树,研究论文发展脉络
Chat Paper
正在生成论文摘要