Lightweight robotic grasping detection network based on dual attention and inverted residual
Transactions of the Institute of Measurement and Control(2024)
摘要
Grasping detection is one of the crucial capabilities for robot systems. Deep learning has achieved remarkable outcomes in robot grasping tasks; however, many deep neural networks were at the expense of high computation cost with memory requirements, which hindered their deployment on computing-constrained devices. To solve this problem, this paper proposes an end-to-end lightweight network with dual attention and inverted residual strategies (LiDAIR), which adopts a generative pixel-level prediction to achieve grasp detection. The LiDAIR is composed of the convolution modules (Conv), the inverted residual convolution module (IRCM), the convolutional block attention connection module (CBACM), and the transposed convolution modules (TConv). The Convs are utilized in downsampling processes to extract the input image features. Then, the IRCM is proposed as a bridge between the downsampling and upsampling phases. In the upsampling phase, the CBACM is designed to focus on the valuable regions from spatial and channel dimensions, where the skip connection is employed to attain multi-level feature fusion. Afterwards, the TConvs are used to restore image resolution. The LiDAIR is lightweight with 704K parameters and enjoys a good tradeoff among lightweight structure, accuracy, and speed. It was evaluated on both the Cornell data set and the Jacquard data set within 10 ms inference time, and the detection accuracy on both the data sets were 97.7% and 92.7%, respectively.
更多查看译文
AI 理解论文
溯源树
样例
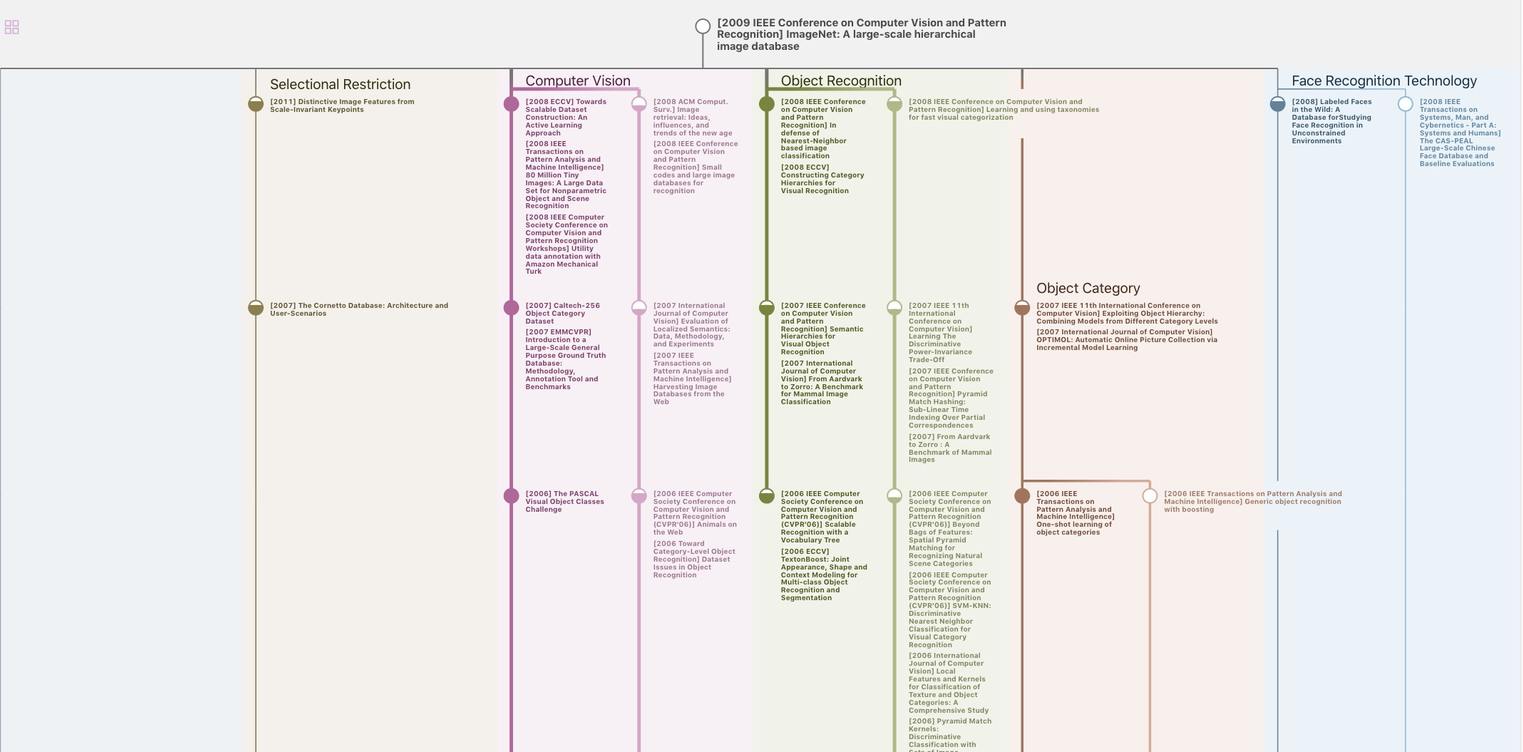
生成溯源树,研究论文发展脉络
Chat Paper
正在生成论文摘要