DQQS: Deep Reinforcement Learning based Technique for Enhancing Security and Performance in SDN-IoT Environments
IEEE Access(2024)
摘要
The Internet of Things (IoT) is an emerging technology that allow smart devices to communicate through various heterogeneous channels (wired or wireless). However, for conventional networks, it has become a challenging task to efficiently control and manage the data flows of a huge number of devices. Software-defined networking (SDN) is a new way of thinking about networking. Because it is programmable, flexible, agile, and gives you a big picture of the network, it has tried to solve some IoT problems, like scalability, heterogeneity, and complexity. In large-scale SDN-IoT networks, there is a requirement for routing protocols that are both efficient and secure in order to ensure a superior level of quality of service (QoS) and quality of experience (QoE). To address the above stated challenges, a novel deep reinforcement learning (DRL) known as DQQS model is proposed. The aim is to achieve QoS and QoE while also ensuring the security of the SDN-IoT network. The proposed DQQS model dynamically extracts patterns from the past network history by interacting with the underlying network and generating optimized routing policies. This article employs three network metrics—throughput, latency, and the probability of avoiding malicious nodes—to measure the performance of DQQS. Simulations reveal that the proposed framework outperforms four state-of-the-art routing algorithms: OSPF, L-L Routing, Sailfish Routing, and RL-Routing in terms of both throughput and latency. For instance, in an attacked environment, the proposed DQQS model achieved the highest throughput value of 14.5 Mbps, surpassing OSPF at 8 Mbps, L-L at 8.2 Mbps, Sailfish at 9 Mbps, and RL at 9.5 Mbps. Similarly, this model exhibited superior performance in latency, recording the lowest latency value of 52 ms, compared to OSPF 88 ms, L-L 85 ms, Sailfish 72 ms, and RL 75 ms routing algorithms. The experimental results demonstrate that this new DQQS model is a pioneering deep reinforcement learning-based technique that optimally addresses secure routing in the SDN-IoT environment, ensuring enhanced quality of service and experience, and outperforming state-of-the art DL methodologies in both security and network performance metrics.
更多查看译文
关键词
Deep Reinforcement Learning,Internet of Things,Malicious Node Detection,Optimal Network Management,Routing Optimization,Software Defined Network,Security
AI 理解论文
溯源树
样例
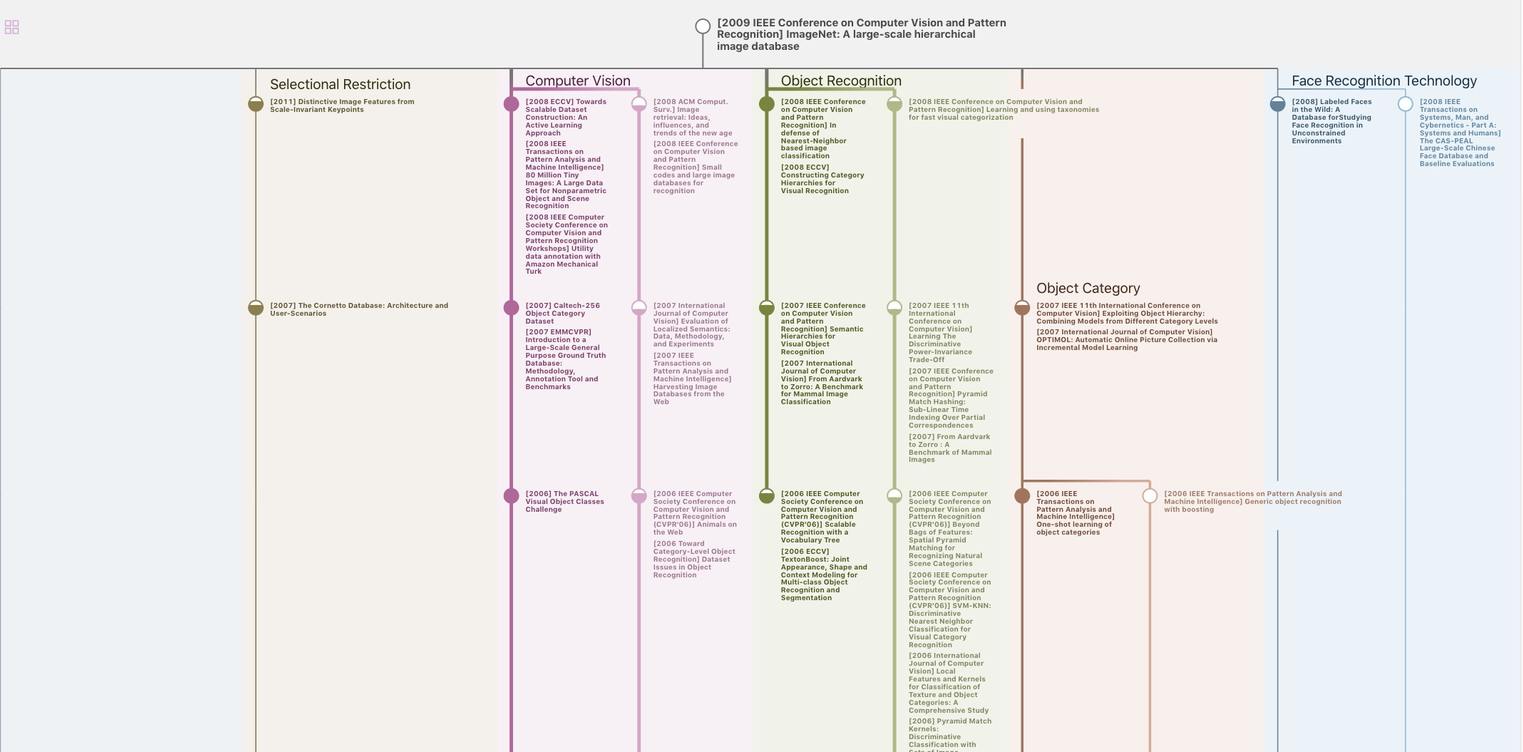
生成溯源树,研究论文发展脉络
Chat Paper
正在生成论文摘要