Caching Video by Distributed Multi-agent Reinforcement Learning
2024 4th International Conference on Neural Networks, Information and Communication (NNICE)(2024)
摘要
Mobile edge computing is a new paradigm to support video caching, aiming to optimize the user’s viewing experience. However, existing works have focused on centralized algorithms, which require a powerful computing center to do scheduling to determine the video content that should be stored on which edge server closest to the user. Therefore, these approach put too much pressure on the backbone network, resulting in increased application costs and reducing its usefulness. To address the above limitation, we consider this scenario without any computing center and propose an innovative distributed video caching algorithm that is different from the previous centralized methods. In our scenario, we no longer need the support of the computing center, but only consider the information interaction between the edge nodes. Our objective is to reduce the average latency to improve the user experience. To this end, we propose a novel decentralized multi-agent reinforcement learning (MARL) algorithm, Distributed Algorithm Without Center (DAWC), implementing in decentralized training and decentralized execution. The main difference between our algorithm and the existing MARL algorithm is that our algorithm is trained distributed, while other algorithms are trained centrally. We further utilize a neural communication protocol to reduce information loss and non-stationary by introducing hidden state and differentiable message encoding and extracting functions. Extensive performance results show that the performance of DAWC is not significantly weaker than that of MARL algorithm with central participation in centralized learning, but other independent learning algorithm and random offloading strategy.
更多查看译文
关键词
deep learning,multi-agent reinforcement learning,decentralize training,video chching
AI 理解论文
溯源树
样例
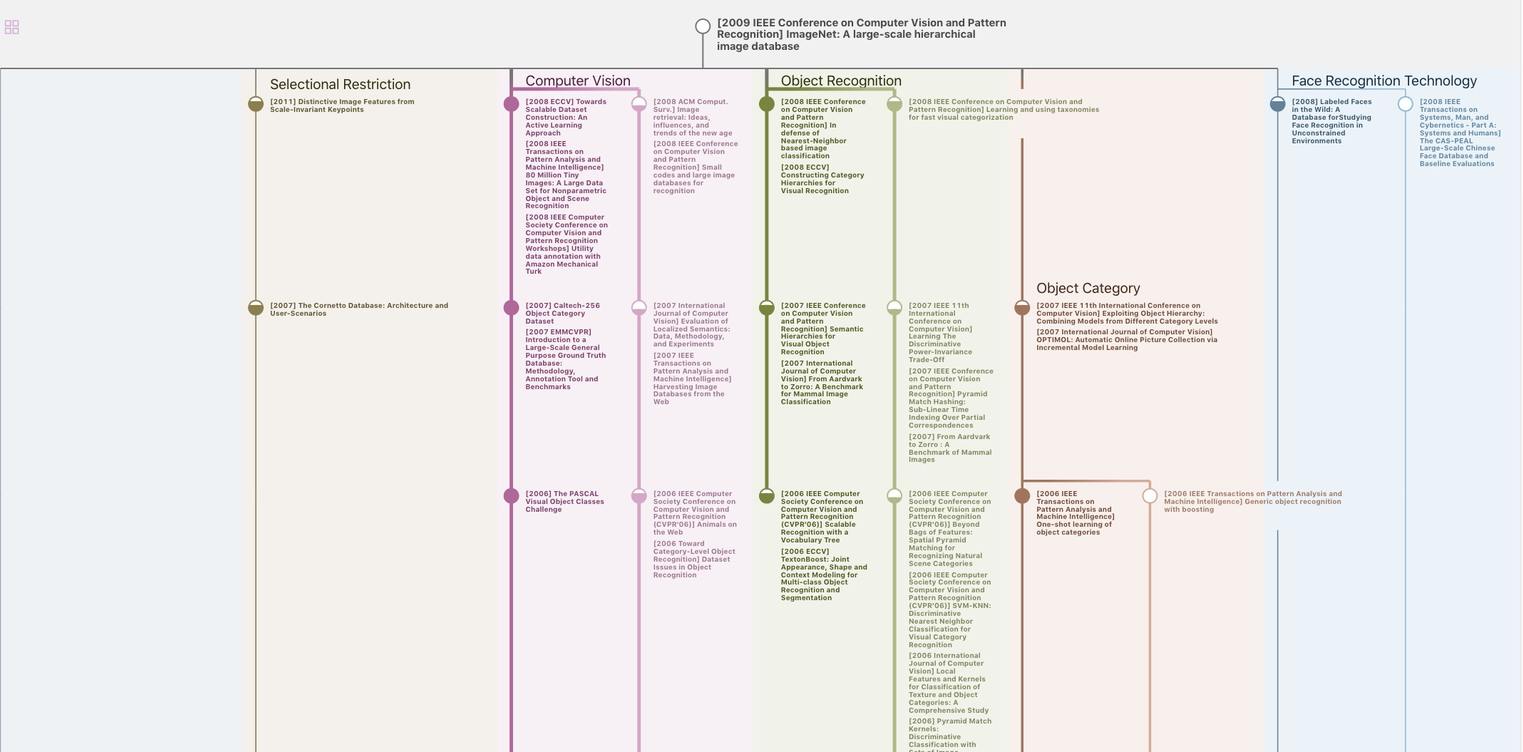
生成溯源树,研究论文发展脉络
Chat Paper
正在生成论文摘要