Short- and Medium-Term Electrical Load Forecasting in Bangladesh Using Machine Learning Approaches
2024 International Conference on Advances in Computing, Communication, Electrical, and Smart Systems (iCACCESS)(2024)
摘要
Planning and managing a power grid relies heavily on accurate predictions of future load. Operational choices involving resource utilization, infrastructural management, implementation schedule planning, investment plans, and maximizing power use while minimizing waste due to under or over-generation all rely on load prediction. In this study, it is utilized three different machine learning algorithms for medium- and short-term load forecasting, namely K-Nearest Neighbors (KNN) based Regression, Random Forest (RF) based regression, and Decision Tree (DT) Regression, to see how well these methods work on half-hourly historical electricity load data from the Bangladesh Power system (BPS) network, which can be found on the Power Grid Company of Bangladesh (PGCB) website and was downloaded between January 2021 and December 2022. To determine the most appropriate method for load forecasting of BPS, a comparison is made between the three approaches concerning the accuracy, mean absolute percentage error (MAPE), root means squared error (RMSE), and mean squared error (MSE). According to the findings, the RF model is superior to the others in making short- and medium-term forecasts due to its greater accuracy, minimal MSE, RMSE, and MAPE.
更多查看译文
关键词
Short-term,Medium-term,KNN,DT,RF,Load forecasting
AI 理解论文
溯源树
样例
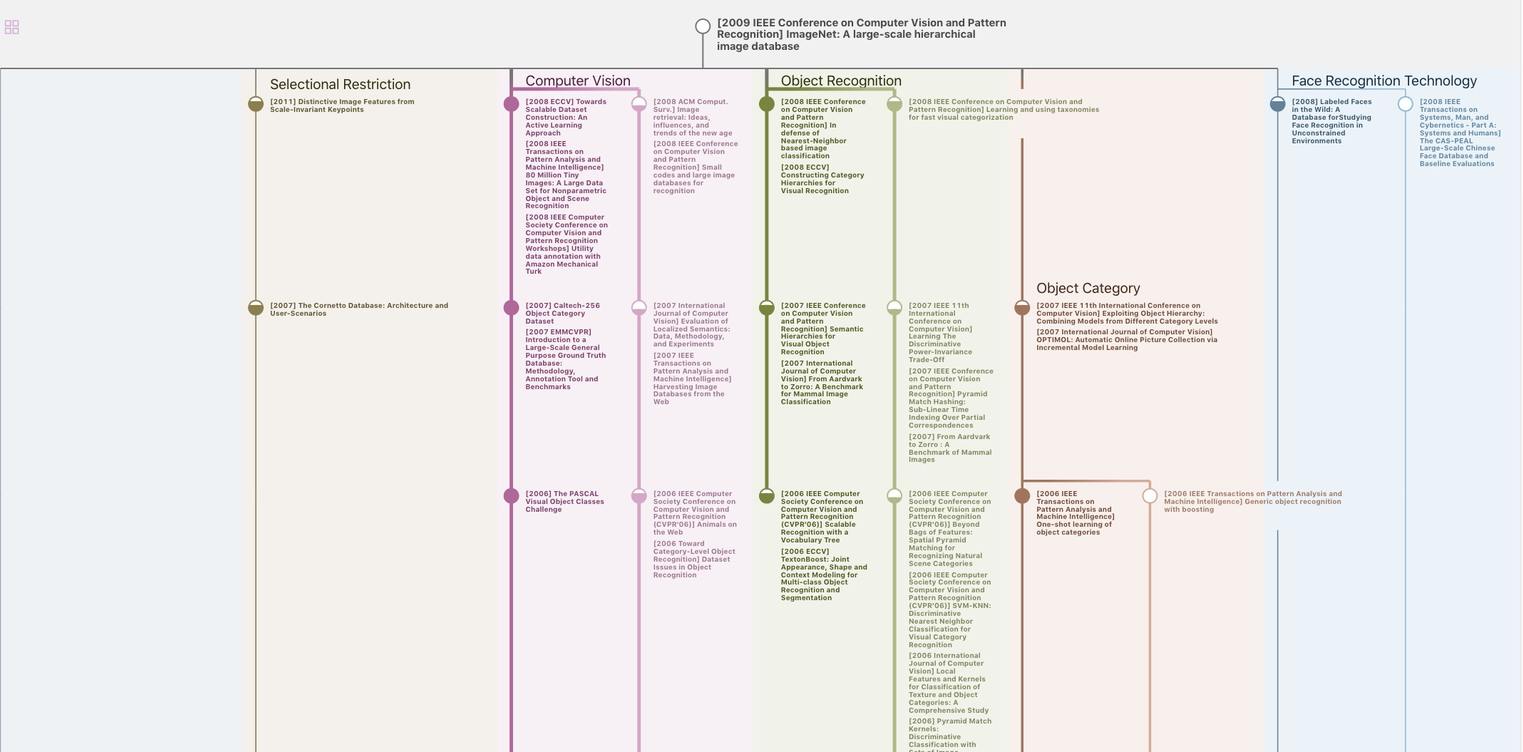
生成溯源树,研究论文发展脉络
Chat Paper
正在生成论文摘要