Efficient and Concise Explanations for Object Detection with Gaussian-Class Activation Mapping Explainer
arxiv(2024)
摘要
To address the challenges of providing quick and plausible explanations in
Explainable AI (XAI) for object detection models, we introduce the Gaussian
Class Activation Mapping Explainer (G-CAME). Our method efficiently generates
concise saliency maps by utilizing activation maps from selected layers and
applying a Gaussian kernel to emphasize critical image regions for the
predicted object. Compared with other Region-based approaches, G-CAME
significantly reduces explanation time to 0.5 seconds without compromising the
quality. Our evaluation of G-CAME, using Faster-RCNN and YOLOX on the MS-COCO
2017 dataset, demonstrates its ability to offer highly plausible and faithful
explanations, especially in reducing the bias on tiny object detection.
更多查看译文
AI 理解论文
溯源树
样例
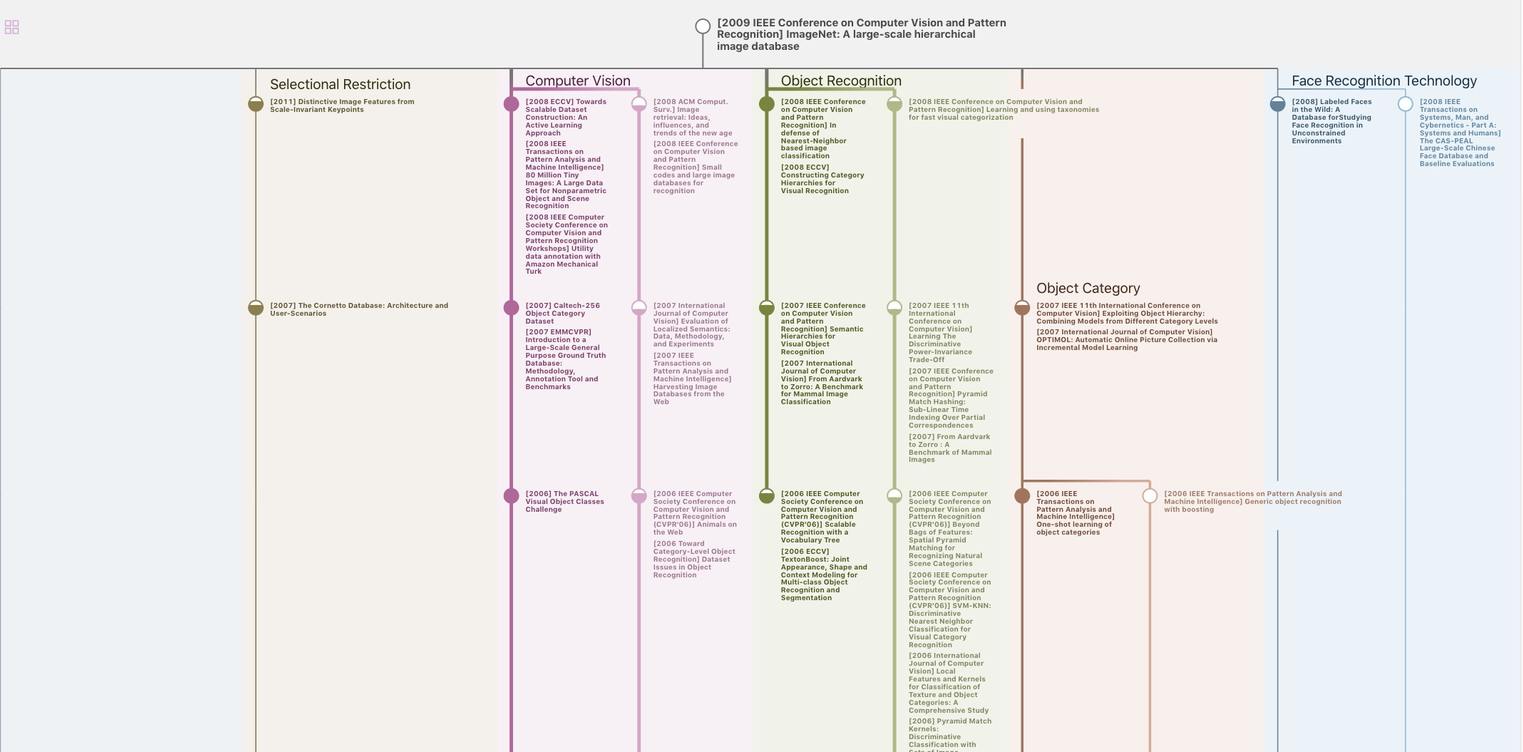
生成溯源树,研究论文发展脉络
Chat Paper
正在生成论文摘要