Machine Learned Classification of Ligand Intrinsic Activity at Human μ-Opioid Receptor.
bioRxiv : the preprint server for biology(2024)
摘要
Opioids are small-molecule agonists of μ-opioid receptor (μOR), while reversal agents such as naloxone are antagonists of mOR. Here we developed machine learning models to classify the intrinsic activities of ligands at the human μOR. We first manually curated a database of 983 small molecules with measured Emax values at the human μOR. Analysis of the chemical space allowed identification of dominant scaffolds and structurally similar agonists and antagonists. Decision tree models and directed message passing neural networks (MPNNs) were then trained to classify agonistic and antagonistic ligands. The hold-out test AUCs of the extra-tree (ET) and MPNN models are 91.5±3.9% and 91.8±4.4%, respectively, while the respective balanced accuracies (BAs) are 83.3±5.0% and 85.1±5.0%. To overcome the challenge of small dataset, a student-teacher learning method called tri-training with disagreement was tested using an unlabeled dataset comprised of 15,816 ligands of human, mouse, or rat μOR, κOR, or δOR. We found that the tri-training scheme was able to increase the MPNN AUC to as high as 9.7%. Taken together, our work provides a proof of concept for developing machine learning models to predict μOR ligand intrinsic activities despite small data size. We envisage many future applications of these models, including evaluation of pharmacologically uncharacterized substances that may pose a risk to public safety and discovery of new rescue agents to combat opioid overdoses.
更多查看译文
AI 理解论文
溯源树
样例
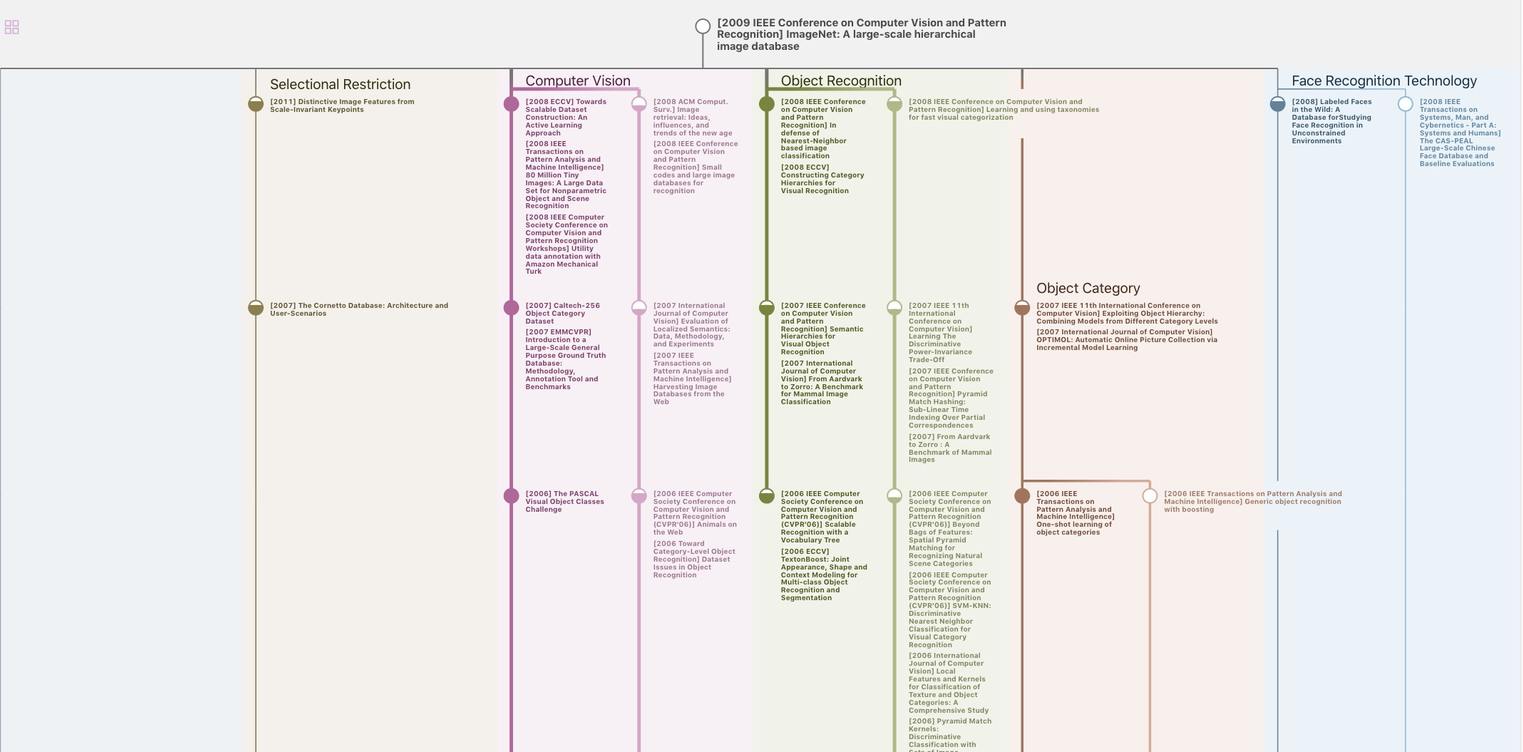
生成溯源树,研究论文发展脉络
Chat Paper
正在生成论文摘要